Scientific and Educational Laboratory of Big Data Analysis Methods
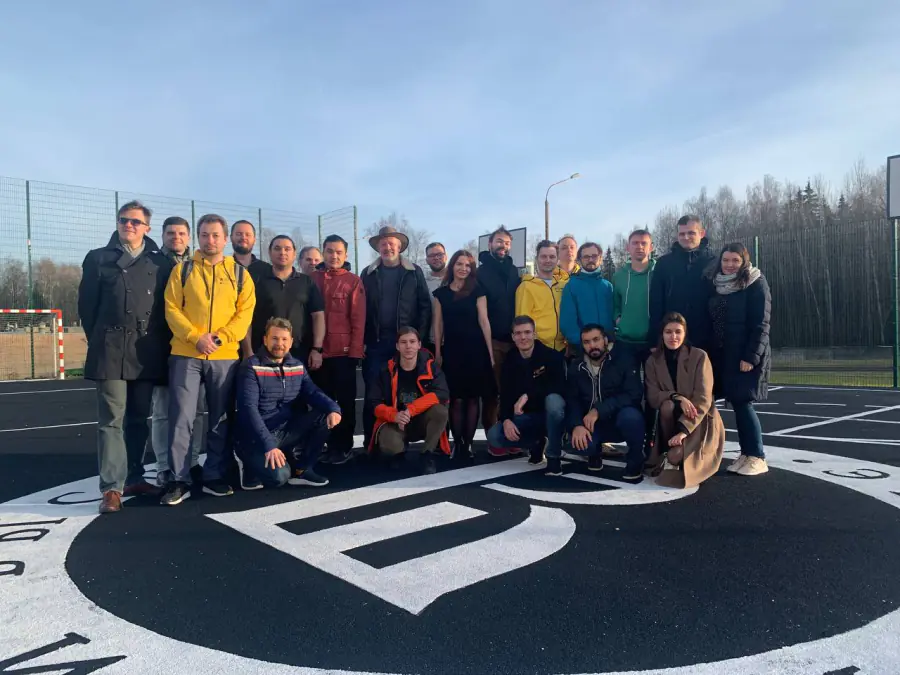
The activity of the Laboratory of Big data Analysis methods is to develop and apply machine learning and data analysis methods to solve problems of fundamental sciences such as particle physics and astrophysics. The search for answers to the mysteries of the universe with leading scientists from these fields is the main direction of the laboratory's development. In particular, we cooperate with the European Center for Nuclear Research (CERN), and our joint work consists both in research on the physics of the events of the Large Hadron Collider and in solving problems of improving the efficiency of data processing. In addition, the laboratory's educational activities include organizing and conducting academic seminars and summer/winter schools on big data analysis and providing scientific guidance to graduate and dissertation papers. The Laboratory of Big Data Analysis Methods was founded in 2015.
- New materials
- Computer search for materials
- Artificial intelligence
Research directions
Natural language for machine learning
Interpreted machine learning models and the search for the laws of nature
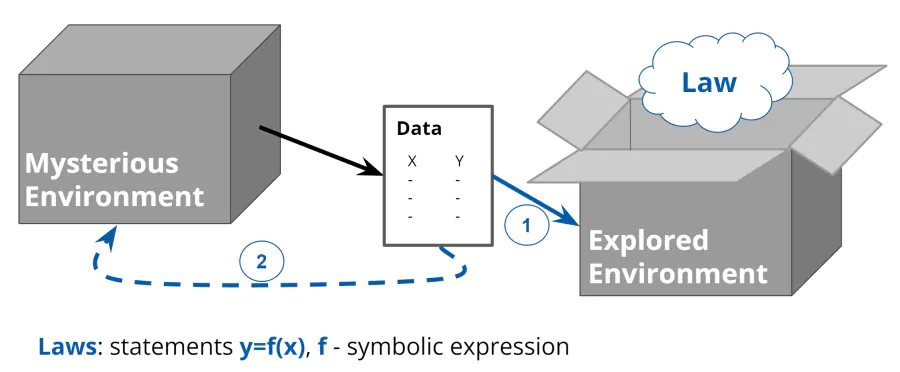
Platforms for evaluating ML models
High-precision digital twin of data storage systems (DSS)
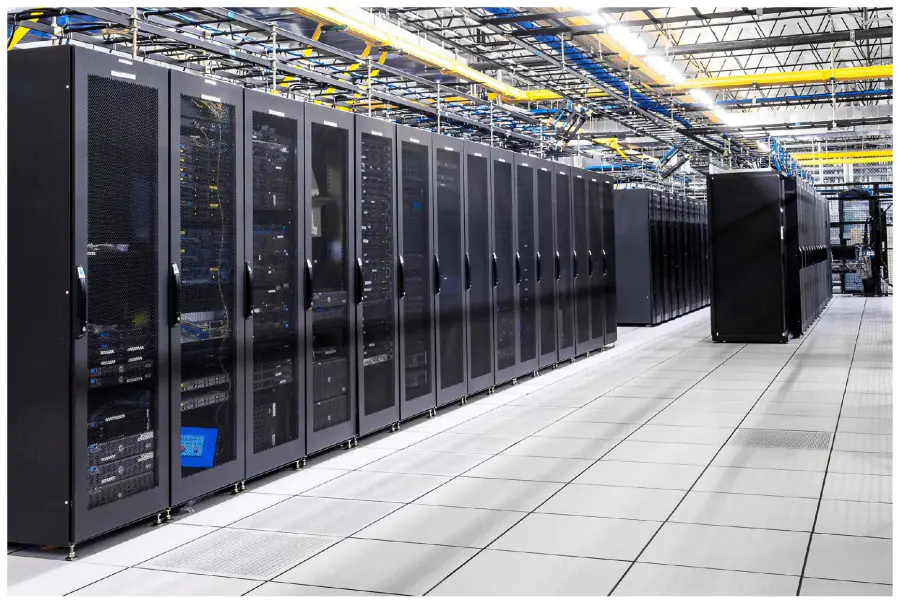
Detecting temporary changes for predictive analytics systems
Updating the weather forecast
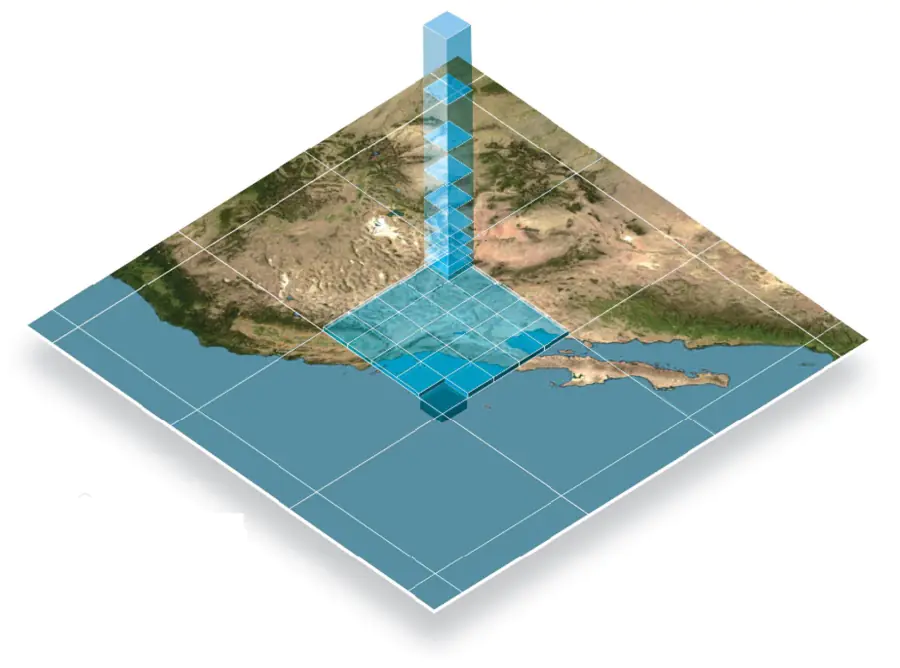
Investigation of two-dimensional materials: prediction of properties and generation according to specified parameters
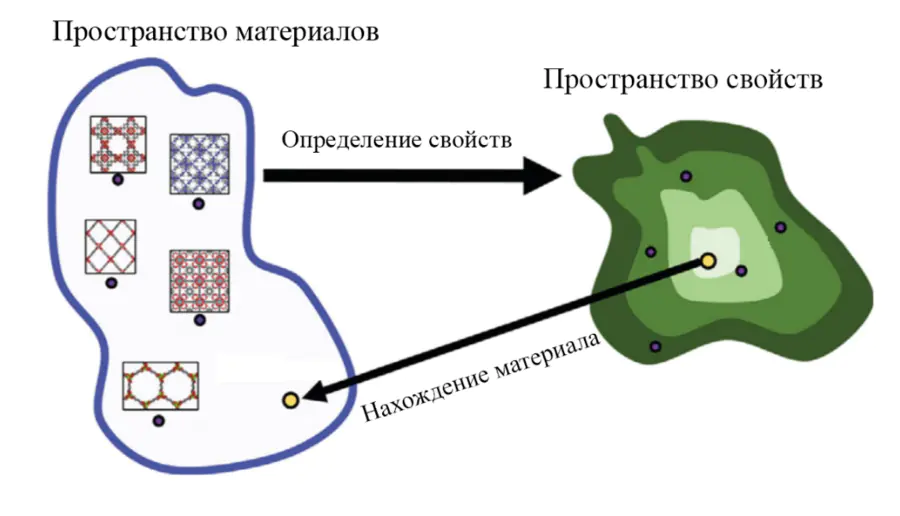