Theoretical Chemistry Group of the IOH RAS
Publications
74
Citations
1 679
h-index
18
Authorization required.
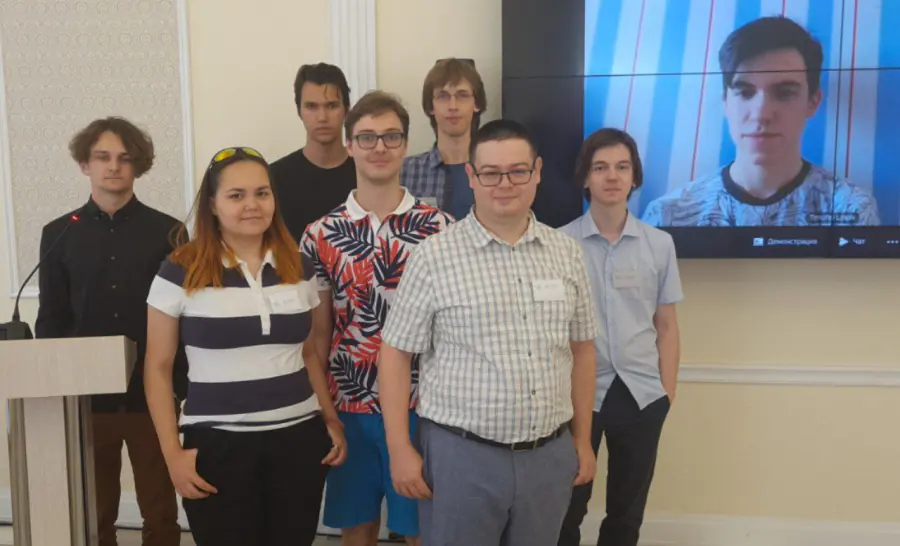
Our open online resources: https://t.me/joinchat/MC9AwMa1oItkZTli TheorChem News is an open Telegram channel used for important announcements concerning our Group.
https://github.com/TheorChemGroup TheorChem GitHub is the GitHub account of our Group, where open source software products developed by us are available, as well as the code used in our publications.
https://t.me/TheorChemLib The TheorChem Library is an open Telegram channel where we share interesting quotes from articles and slides from conferences.
- DFT calculations
- Machine learning
- Molecular dynamics and quantum chemical calculations
Michael Medvedev
Head of Laboratory
Daniil Artamonov
Research assistant
Ruslan Khalykov
Student
Alexey Bondarev
Student
Anna Lapteva
Student
Research directions
Neuromorphic networks
+
Studying the processes of the brain and thinking is one of the most difficult questions that researchers around the world are asking. Our work focuses on studying experimental data and testing hypotheses about the nature of processes, as well as building brain-inspired neural networks to solve deep learning problems. Our projects combine knowledge and methods from neuroscience, brain biochemistry, cell biophysics, machine learning and mathematics, which is a fairly interdisciplinary approach to research. Globally, all projects can be divided into 3 areas: 1. Study and modeling of brain processes - analysis of experimental data and further simulation based on them 2.Computational neuromorphic interfaces - training and evaluation of impulse neural networks (they are also neuromorphic) 3. Development of algorithms and tools - to facilitate modeling tasks In the future, it is planned to implement the acquired knowledge in other fields of science. For example, the study and modeling of brain processes can help in the fight against neurodegenerative diseases, and neuromorphic networks to improve existing models in machine learning.
Development of modeling methods
+
When modeling the mechanisms of chemical reactions and other processes, it is necessary to choose a method that will allow obtaining results close to experimental data in an adequate time. What should I do if there is no such method? What if the existing methods actually lie? The work of our Group is also related to the answers to these questions. As part of the development of new methods, we are working on approaches to the conformational analysis of small molecules, on improving ways to account for solvation energies and solvent free energy. In addition, we are trying to make more accurate methods designed to speed up calculations: we are creating new features for machine-learning functions and ways to "safely" train neural networks, and we are improving the reliability of force fields. We also do not forget about ab initio methods when developing new basic sets. The Group's research interests also include biochemical systems: an approach to the quantum chemical study of the activity of bioisosters has already been published, and work is underway on ways to refine the structure of proteins. As part of the study of the properties of solids and processes on the surface, we are developing approaches to molecular dynamics and crystal metadynamics to predict the crystal structures of organic substances. An important part of the Group's work is the validation of existing methods of theoretical chemistry. The main achievements in this field are the demonstration of the overfitting of some density functionals, as well as the detection of problems with the DeepMind functional 21. The group is working on ways to verify the reliability of TFP methods, as well as approaches to the study of stereoelectronic effects and interaction energies in organic molecules.
Modeling of chemical processes
+
One of the areas of work of our group is the modeling of chemical processes. By this we mean any processes described by chemical reactions. These include synthetic chemical reactions, biochemical processes, and processes in materials. Our interests include: 1) molecular modeling of chemical reactions, determination of their mechanisms, prediction of product properties, calculation of spectra and much more; 2) modeling of biochemical processes, calculation of binding abilities and structures of ligand and protein; 3) study of materials, their properties and the production process. To describe the world around us, we use a wide range of methods of theoretical chemistry, ranging from universal molecular dynamics to the analysis of the population of orbitals. These and many other methods make it possible to predict the result of chemical processes without having to carry it out in reality, which means that they allow screening with a wide range of initial parameters without having to spend time and resources on experiments. For example, we can answer the questions of which catalyst will maximize regioselectivity or which deposition conditions will lead to a material with the required properties. To answer these questions, a process model is needed, and this is the first thing we always do. That's why you hear the word modeling so often, building a model is a key step. The picture below shows the algorithm for building the model. Studying the literature and communicating with experimenters, we first build a "mental" model of the process in our head, and then transfer it to a computer. Which approximations to choose, which atoms to consider, and the answers to these questions create a model of the process that describes it accurately enough to predict certain properties.
Artificial intelligence
+
One of the areas of work of our group is the application of artificial intelligence and machine learning methods to solve problems in the field of chemistry. We are developing algorithms that allow computers to analyze chemical data, build models of chemical processes and make reliable predictions. We also actively use physically based models. This allows the algorithms to include knowledge about chemical principles and patterns. Thanks to this, our models are better generalized to new data, and predictions become more reliable. Our area of interest includes: 1) predicting the properties of chemical compounds using machine learning methods; 2) planning synthetic pathways to obtain the right substances using artificial intelligence; 3) modeling the structure and properties of materials based on big data and deep learning. We use advanced algorithms such as neural networks, graph convolutional networks, variational autoencoders and others. These methods allow us to build highly accurate models without the need for a complete understanding of the physical principles.
Publications and patents
Lab address
Ленинский просп., 47, стр. 1, Москва
Authorization required.