Hanoi University of Mining and Geology
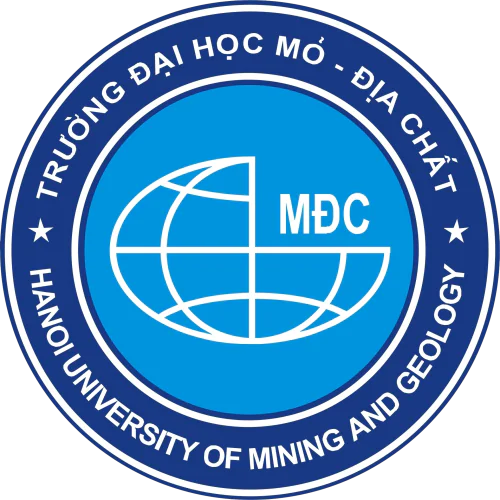
Since 1994
Total publications
1020
Total citations
19394
Citations per publication
19.01
Average publications per year
32.9
Average authors per publication
5.55
h-index
66
Metrics description
h-index
A scientist has an h-index if h of his N publications are cited at least h times each, while the remaining (N - h) publications are cited no more than h times each.
Top-30
Fields of science
20
40
60
80
100
120
|
|
Geology
|
Geology, 104, 10.2%
Geology
104 publications, 10.2%
|
General Materials Science
|
General Materials Science, 90, 8.82%
General Materials Science
90 publications, 8.82%
|
Geotechnical Engineering and Engineering Geology
|
Geotechnical Engineering and Engineering Geology, 87, 8.53%
Geotechnical Engineering and Engineering Geology
87 publications, 8.53%
|
General Chemistry
|
General Chemistry, 62, 6.08%
General Chemistry
62 publications, 6.08%
|
Pollution
|
Pollution, 62, 6.08%
Pollution
62 publications, 6.08%
|
General Medicine
|
General Medicine, 61, 5.98%
General Medicine
61 publications, 5.98%
|
Electrical and Electronic Engineering
|
Electrical and Electronic Engineering, 61, 5.98%
Electrical and Electronic Engineering
61 publications, 5.98%
|
Civil and Structural Engineering
|
Civil and Structural Engineering, 59, 5.78%
Civil and Structural Engineering
59 publications, 5.78%
|
General Engineering
|
General Engineering, 57, 5.59%
General Engineering
57 publications, 5.59%
|
Geochemistry and Petrology
|
Geochemistry and Petrology, 57, 5.59%
Geochemistry and Petrology
57 publications, 5.59%
|
General Earth and Planetary Sciences
|
General Earth and Planetary Sciences, 55, 5.39%
General Earth and Planetary Sciences
55 publications, 5.39%
|
Computer Science Applications
|
Computer Science Applications, 49, 4.8%
Computer Science Applications
49 publications, 4.8%
|
Building and Construction
|
Building and Construction, 47, 4.61%
Building and Construction
47 publications, 4.61%
|
Environmental Chemistry
|
Environmental Chemistry, 45, 4.41%
Environmental Chemistry
45 publications, 4.41%
|
Water Science and Technology
|
Water Science and Technology, 45, 4.41%
Water Science and Technology
45 publications, 4.41%
|
Geophysics
|
Geophysics, 42, 4.12%
Geophysics
42 publications, 4.12%
|
General Chemical Engineering
|
General Chemical Engineering, 41, 4.02%
General Chemical Engineering
41 publications, 4.02%
|
General Environmental Science
|
General Environmental Science, 41, 4.02%
General Environmental Science
41 publications, 4.02%
|
Earth-Surface Processes
|
Earth-Surface Processes, 41, 4.02%
Earth-Surface Processes
41 publications, 4.02%
|
Biochemistry
|
Biochemistry, 39, 3.82%
Biochemistry
39 publications, 3.82%
|
Condensed Matter Physics
|
Condensed Matter Physics, 39, 3.82%
Condensed Matter Physics
39 publications, 3.82%
|
Analytical Chemistry
|
Analytical Chemistry, 37, 3.63%
Analytical Chemistry
37 publications, 3.63%
|
Electronic, Optical and Magnetic Materials
|
Electronic, Optical and Magnetic Materials, 34, 3.33%
Electronic, Optical and Magnetic Materials
34 publications, 3.33%
|
Software
|
Software, 34, 3.33%
Software
34 publications, 3.33%
|
Instrumentation
|
Instrumentation, 33, 3.24%
Instrumentation
33 publications, 3.24%
|
Industrial and Manufacturing Engineering
|
Industrial and Manufacturing Engineering, 32, 3.14%
Industrial and Manufacturing Engineering
32 publications, 3.14%
|
Health, Toxicology and Mutagenesis
|
Health, Toxicology and Mutagenesis, 32, 3.14%
Health, Toxicology and Mutagenesis
32 publications, 3.14%
|
Atomic and Molecular Physics, and Optics
|
Atomic and Molecular Physics, and Optics, 31, 3.04%
Atomic and Molecular Physics, and Optics
31 publications, 3.04%
|
Mechanics of Materials
|
Mechanics of Materials, 30, 2.94%
Mechanics of Materials
30 publications, 2.94%
|
Process Chemistry and Technology
|
Process Chemistry and Technology, 29, 2.84%
Process Chemistry and Technology
29 publications, 2.84%
|
20
40
60
80
100
120
|
Journals
5
10
15
20
25
30
35
40
45
50
|
|
Lecture Notes in Civil Engineering
47 publications, 4.61%
|
|
Environmental Science and Engineering
28 publications, 2.75%
|
|
Natural Resources Research
21 publications, 2.06%
|
|
Vietnam Journal of Earth Sciences
21 publications, 2.06%
|
|
Remote Sensing
20 publications, 1.96%
|
|
Engineering with Computers
18 publications, 1.76%
|
|
Environmental Earth Sciences
17 publications, 1.67%
|
|
Construction and Building Materials
17 publications, 1.67%
|
|
E3S Web of Conferences
16 publications, 1.57%
|
|
Applied Sciences (Switzerland)
14 publications, 1.37%
|
|
Tunnelling and Underground Space Technology
13 publications, 1.27%
|
|
Vietnam Journal of Chemistry
13 publications, 1.27%
|
|
IOP Conference Series: Earth and Environmental Science
12 publications, 1.18%
|
|
Journal of Radioanalytical and Nuclear Chemistry
12 publications, 1.18%
|
|
Journal of Asian Earth Sciences
11 publications, 1.08%
|
|
Sensors
11 publications, 1.08%
|
|
Journal of Physics: Conference Series
9 publications, 0.88%
|
|
Lecture Notes of the Institute for Computer Sciences, Social-Informatics and Telecommunications Engineering
9 publications, 0.88%
|
|
Gornye nauki i tekhnologii = Mining Science and Technology (Russia)
9 publications, 0.88%
|
|
Natural Product Research
8 publications, 0.78%
|
|
Lecture Notes in Networks and Systems
8 publications, 0.78%
|
|
Gondwana Research
8 publications, 0.78%
|
|
Vietnam Journal of Marine Science and Technology
8 publications, 0.78%
|
|
Acta Geophysica
7 publications, 0.69%
|
|
Geocarto International
7 publications, 0.69%
|
|
Scientific Reports
7 publications, 0.69%
|
|
Natural Product Communications
7 publications, 0.69%
|
|
International Geology Review
6 publications, 0.59%
|
|
Ore Geology Reviews
6 publications, 0.59%
|
|
Geochimica et Cosmochimica Acta
6 publications, 0.59%
|
|
5
10
15
20
25
30
35
40
45
50
|
Publishers
50
100
150
200
250
300
350
|
|
Springer Nature
304 publications, 29.8%
|
|
Elsevier
244 publications, 23.92%
|
|
Multidisciplinary Digital Publishing Institute (MDPI)
86 publications, 8.43%
|
|
Taylor & Francis
64 publications, 6.27%
|
|
Wiley
49 publications, 4.8%
|
|
Publishing House for Science and Technology, Vietnam Academy of Science and Technology (Publications)
41 publications, 4.02%
|
|
IOP Publishing
31 publications, 3.04%
|
|
EDP Sciences
19 publications, 1.86%
|
|
Hindawi Limited
19 publications, 1.86%
|
|
Oxford University Press
14 publications, 1.37%
|
|
IEEE
11 publications, 1.08%
|
|
SAGE
9 publications, 0.88%
|
|
National University of Science & Technology (MISiS)
9 publications, 0.88%
|
|
American Chemical Society (ACS)
8 publications, 0.78%
|
|
World Scientific
6 publications, 0.59%
|
|
Royal Society of Chemistry (RSC)
6 publications, 0.59%
|
|
Copernicus
6 publications, 0.59%
|
|
Thomas Telford
6 publications, 0.59%
|
|
IWA Publishing
5 publications, 0.49%
|
|
Walter de Gruyter
4 publications, 0.39%
|
|
Pleiades Publishing
4 publications, 0.39%
|
|
Chinese Academy of Sciences
4 publications, 0.39%
|
|
Trans Tech Publications
3 publications, 0.29%
|
|
Frontiers Media S.A.
3 publications, 0.29%
|
|
American Institute of Physics (AIP)
3 publications, 0.29%
|
|
International Union of Crystallography (IUCr)
3 publications, 0.29%
|
|
American Physical Society (APS)
3 publications, 0.29%
|
|
Chinese Society of Rare Earths
3 publications, 0.29%
|
|
Diponegoro university Indonesia - Center of Biomass and Renewable Energy (CBIORE)
3 publications, 0.29%
|
|
Institution of Chemical Engineers
3 publications, 0.29%
|
|
50
100
150
200
250
300
350
|
With other organizations
20
40
60
80
100
120
140
160
180
200
|
|
Vietnam Academy of Science and Technology
198 publications, 19.41%
|
|
Duy Tan University
122 publications, 11.96%
|
|
Ton Duc Thang University
65 publications, 6.37%
|
|
VNU University of Science
57 publications, 5.59%
|
|
Vietnam National University, Hanoi
46 publications, 4.51%
|
|
Hanoi University of Science and Technology
40 publications, 3.92%
|
|
Hanoi University of Natural Resources and Environment
39 publications, 3.82%
|
|
Hanoi National University of Education
37 publications, 3.63%
|
|
Grenoble Alpes University
35 publications, 3.43%
|
|
University of South-Eastern Norway
28 publications, 2.75%
|
|
Sejong University
24 publications, 2.35%
|
|
Institute for Tropical Technology
20 publications, 1.96%
|
|
Le Quy Don Technical University
20 publications, 1.96%
|
|
Michael Okpara University of Agriculture
19 publications, 1.86%
|
|
Hanoi University
18 publications, 1.76%
|
|
Hue University
18 publications, 1.76%
|
|
Tongji University
17 publications, 1.67%
|
|
Central South University
17 publications, 1.67%
|
|
Curtin University
16 publications, 1.57%
|
|
University of Bristol
16 publications, 1.57%
|
|
Institute of Marine Geology and Geophysics
15 publications, 1.47%
|
|
University of Technology, Malaysia
15 publications, 1.47%
|
|
Geological Survey of Denmark and Greenland
15 publications, 1.47%
|
|
University of Pannonia
15 publications, 1.47%
|
|
Saint Petersburg Mining University
14 publications, 1.37%
|
|
Moscow State University of Civil Engineering
14 publications, 1.37%
|
|
Pukyong National University
14 publications, 1.37%
|
|
Vietnam National University Ho Chi Minh City
12 publications, 1.18%
|
|
Thai Nguyen University
12 publications, 1.18%
|
|
Vietnam Maritime University
12 publications, 1.18%
|
|
20
40
60
80
100
120
140
160
180
200
|
With foreign organizations
5
10
15
20
25
30
35
|
|
Grenoble Alpes University
35 publications, 3.43%
|
|
University of South-Eastern Norway
28 publications, 2.75%
|
|
Sejong University
24 publications, 2.35%
|
|
Michael Okpara University of Agriculture
19 publications, 1.86%
|
|
Tongji University
17 publications, 1.67%
|
|
Central South University
17 publications, 1.67%
|
|
Curtin University
16 publications, 1.57%
|
|
University of Bristol
16 publications, 1.57%
|
|
University of Technology, Malaysia
15 publications, 1.47%
|
|
Geological Survey of Denmark and Greenland
15 publications, 1.47%
|
|
University of Pannonia
15 publications, 1.47%
|
|
Saint Petersburg Mining University
14 publications, 1.37%
|
|
Moscow State University of Civil Engineering
14 publications, 1.37%
|
|
Pukyong National University
14 publications, 1.37%
|
|
Norwegian University of Life Sciences
12 publications, 1.18%
|
|
Chonnam National University
12 publications, 1.18%
|
|
Polytechnic University of Turin
11 publications, 1.08%
|
|
Yonsei University
11 publications, 1.08%
|
|
University Putra Malaysia
10 publications, 0.98%
|
|
China University of Geosciences (Beijing)
10 publications, 0.98%
|
|
China University of Geosciences (Wuhan)
10 publications, 0.98%
|
|
Vienna University of Technology
9 publications, 0.88%
|
|
China University of Mining and Technology
8 publications, 0.78%
|
|
Norwegian University of Science and Technology
8 publications, 0.78%
|
|
Technical University of Denmark
8 publications, 0.78%
|
|
Hefei University of Technology
8 publications, 0.78%
|
|
Tarbiat Modares University
7 publications, 0.69%
|
|
University of Technology Sydney
7 publications, 0.69%
|
|
Helmholtz-Zentrum Dresden-Rossendorf
7 publications, 0.69%
|
|
Helmholtz Institute Freiberg for Resource Technology
7 publications, 0.69%
|
|
5
10
15
20
25
30
35
|
With other countries
20
40
60
80
100
120
140
|
|
China
|
China, 137, 13.43%
China
137 publications, 13.43%
|
France
|
France, 92, 9.02%
France
92 publications, 9.02%
|
Republic of Korea
|
Republic of Korea, 89, 8.73%
Republic of Korea
89 publications, 8.73%
|
Norway
|
Norway, 54, 5.29%
Norway
54 publications, 5.29%
|
Russia
|
Russia, 50, 4.9%
Russia
50 publications, 4.9%
|
Germany
|
Germany, 48, 4.71%
Germany
48 publications, 4.71%
|
Australia
|
Australia, 44, 4.31%
Australia
44 publications, 4.31%
|
USA
|
USA, 40, 3.92%
USA
40 publications, 3.92%
|
United Kingdom
|
United Kingdom, 39, 3.82%
United Kingdom
39 publications, 3.82%
|
Japan
|
Japan, 39, 3.82%
Japan
39 publications, 3.82%
|
India
|
India, 33, 3.24%
India
33 publications, 3.24%
|
Malaysia
|
Malaysia, 29, 2.84%
Malaysia
29 publications, 2.84%
|
Poland
|
Poland, 29, 2.84%
Poland
29 publications, 2.84%
|
Iran
|
Iran, 25, 2.45%
Iran
25 publications, 2.45%
|
Italy
|
Italy, 25, 2.45%
Italy
25 publications, 2.45%
|
Nigeria
|
Nigeria, 21, 2.06%
Nigeria
21 publications, 2.06%
|
Denmark
|
Denmark, 19, 1.86%
Denmark
19 publications, 1.86%
|
Austria
|
Austria, 17, 1.67%
Austria
17 publications, 1.67%
|
Canada
|
Canada, 16, 1.57%
Canada
16 publications, 1.57%
|
Hungary
|
Hungary, 15, 1.47%
Hungary
15 publications, 1.47%
|
Belgium
|
Belgium, 12, 1.18%
Belgium
12 publications, 1.18%
|
Romania
|
Romania, 11, 1.08%
Romania
11 publications, 1.08%
|
Spain
|
Spain, 10, 0.98%
Spain
10 publications, 0.98%
|
Thailand
|
Thailand, 9, 0.88%
Thailand
9 publications, 0.88%
|
Sweden
|
Sweden, 8, 0.78%
Sweden
8 publications, 0.78%
|
South Africa
|
South Africa, 7, 0.69%
South Africa
7 publications, 0.69%
|
Brazil
|
Brazil, 6, 0.59%
Brazil
6 publications, 0.59%
|
Greece
|
Greece, 6, 0.59%
Greece
6 publications, 0.59%
|
Egypt
|
Egypt, 6, 0.59%
Egypt
6 publications, 0.59%
|
Pakistan
|
Pakistan, 6, 0.59%
Pakistan
6 publications, 0.59%
|
20
40
60
80
100
120
140
|
- We do not take into account publications that without a DOI.
- Statistics recalculated daily.
- Publications published earlier than 1994 are ignored in the statistics.
- The horizontal charts show the 30 top positions.
- Journals quartiles values are relevant at the moment.
{"yearsFrom":1994,"yearsTo":2024,"articlesYearsTotal":26,"articlesYearsFrom":1994,"articlesYearsTo":2024,"citationsYearsTotal":26,"citationsYearsFrom":0,"citationsYearsTo":0,"totalCitations":19394,"totalPublications":1020,"averageCitations":"19.01","averagePublicationsPerYear":"32.9","averageAuthors":"5.55","hIndex":66,"iTenIndex":377,"mIndex":"2.13","oIndex":"238.66","gIndex":108,"wIndex":16,"journals":{"type":"bar","data":{"show":true,"labels":["Lecture Notes in Civil Engineering","Environmental Science and Engineering","Natural Resources Research","Vietnam Journal of Earth Sciences","Remote Sensing","Engineering with Computers","Environmental Earth Sciences","Construction and Building Materials","E3S Web of Conferences","Applied Sciences (Switzerland)","Tunnelling and Underground Space Technology","Vietnam Journal of Chemistry","IOP Conference Series: Earth and Environmental Science","Journal of Radioanalytical and Nuclear Chemistry","Journal of Asian Earth Sciences","Sensors","Journal of Physics: Conference Series","Lecture Notes of the Institute for Computer Sciences, Social-Informatics and Telecommunications Engineering","Gornye nauki i tekhnologii = Mining Science and Technology (Russia)","Natural Product Research","Lecture Notes in Networks and Systems","Gondwana Research","Vietnam Journal of Marine Science and Technology","Acta Geophysica","Geocarto International","Scientific Reports","Natural Product Communications","International Geology Review","Ore Geology Reviews","Geochimica et Cosmochimica Acta"],"ids":[6943,4418,920,31869,22827,15345,6025,19466,11409,16650,5830,27013,6042,12902,671,18435,3333,6646,36051,7474,17269,19462,27778,2042,12536,13767,15287,1281,10683,16961],"codes":[0,0,0,0,0,0,0,0,0,0,0,0,0,0,0,0,0,0,0,0,0,0,0,0,0,0,0,0,0,0],"imageUrls":["\/storage\/images\/resized\/voXLqlsvTwv5p3iMQ8Dhs95nqB4AXOG7Taj7G4ra_medium.webp","\/storage\/images\/resized\/voXLqlsvTwv5p3iMQ8Dhs95nqB4AXOG7Taj7G4ra_medium.webp","\/storage\/images\/resized\/voXLqlsvTwv5p3iMQ8Dhs95nqB4AXOG7Taj7G4ra_medium.webp","\/storage\/images\/resized\/aaZxju90lOnC0t1v0UdT44gk6x3DjeOtYm5Cj61p_medium.webp","\/storage\/images\/resized\/MjH1ITP7lMYGxeqUZfkt2BnVLgjkk413jwBV97XX_medium.webp","\/storage\/images\/resized\/voXLqlsvTwv5p3iMQ8Dhs95nqB4AXOG7Taj7G4ra_medium.webp","\/storage\/images\/resized\/voXLqlsvTwv5p3iMQ8Dhs95nqB4AXOG7Taj7G4ra_medium.webp","\/storage\/images\/resized\/GDnYOu1UpMMfMMRV6Aqle4H0YLLsraeD9IP9qScG_medium.webp","\/storage\/images\/resized\/7GcDgL5Sa2zewDk4sRy4fs0GznbYZIsSV9wqMs28_medium.webp","\/storage\/images\/resized\/MjH1ITP7lMYGxeqUZfkt2BnVLgjkk413jwBV97XX_medium.webp","\/storage\/images\/resized\/GDnYOu1UpMMfMMRV6Aqle4H0YLLsraeD9IP9qScG_medium.webp","\/storage\/images\/resized\/bRyGpdm98BkAUYiK1YFNpl5Z7hPu6Gd87gbIeuG3_medium.webp","\/storage\/images\/resized\/LsKy6OnmmmRGcAU6CZgWQvNiP1polbaSLNrN7zqj_medium.webp","\/storage\/images\/resized\/voXLqlsvTwv5p3iMQ8Dhs95nqB4AXOG7Taj7G4ra_medium.webp","\/storage\/images\/resized\/GDnYOu1UpMMfMMRV6Aqle4H0YLLsraeD9IP9qScG_medium.webp","\/storage\/images\/resized\/MjH1ITP7lMYGxeqUZfkt2BnVLgjkk413jwBV97XX_medium.webp","\/storage\/images\/resized\/LsKy6OnmmmRGcAU6CZgWQvNiP1polbaSLNrN7zqj_medium.webp","\/storage\/images\/resized\/voXLqlsvTwv5p3iMQ8Dhs95nqB4AXOG7Taj7G4ra_medium.webp","\/storage\/images\/resized\/dRvP3lMJcjXP3GTJzfOCMGs4rAy4Aq1F3yWpmEzN_medium.webp","\/storage\/images\/resized\/5YZtvLvkPZuc2JHOaZsjCvGSHFCuC3drUwN3YAc5_medium.webp","\/storage\/images\/resized\/voXLqlsvTwv5p3iMQ8Dhs95nqB4AXOG7Taj7G4ra_medium.webp","\/storage\/images\/resized\/GDnYOu1UpMMfMMRV6Aqle4H0YLLsraeD9IP9qScG_medium.webp","\/storage\/images\/resized\/aaZxju90lOnC0t1v0UdT44gk6x3DjeOtYm5Cj61p_medium.webp","\/storage\/images\/resized\/voXLqlsvTwv5p3iMQ8Dhs95nqB4AXOG7Taj7G4ra_medium.webp","\/storage\/images\/resized\/5YZtvLvkPZuc2JHOaZsjCvGSHFCuC3drUwN3YAc5_medium.webp","\/storage\/images\/resized\/voXLqlsvTwv5p3iMQ8Dhs95nqB4AXOG7Taj7G4ra_medium.webp","\/storage\/images\/resized\/ruydfaB80LDjlkYqsfOeUAZohOIODyq7bQzis5O7_medium.webp","\/storage\/images\/resized\/5YZtvLvkPZuc2JHOaZsjCvGSHFCuC3drUwN3YAc5_medium.webp","\/storage\/images\/resized\/GDnYOu1UpMMfMMRV6Aqle4H0YLLsraeD9IP9qScG_medium.webp","\/storage\/images\/resized\/GDnYOu1UpMMfMMRV6Aqle4H0YLLsraeD9IP9qScG_medium.webp"],"datasets":[{"label":"","data":[47,28,21,21,20,18,17,17,16,14,13,13,12,12,11,11,9,9,9,8,8,8,8,7,7,7,7,6,6,6],"backgroundColor":["#3B82F6","#3B82F6","#3B82F6","#3B82F6","#3B82F6","#3B82F6","#3B82F6","#3B82F6","#3B82F6","#3B82F6","#3B82F6","#3B82F6","#3B82F6","#3B82F6","#3B82F6","#3B82F6","#3B82F6","#3B82F6","#3B82F6","#3B82F6","#3B82F6","#3B82F6","#3B82F6","#3B82F6","#3B82F6","#3B82F6","#3B82F6","#3B82F6","#3B82F6","#3B82F6"],"percentage":[4.61,2.75,2.06,2.06,1.96,1.76,1.67,1.67,1.57,1.37,1.27,1.27,1.18,1.18,1.08,1.08,0.88,0.88,0.88,0.78,0.78,0.78,0.78,0.69,0.69,0.69,0.69,0.59,0.59,0.59],"barThickness":13}]},"options":{"indexAxis":"y","maintainAspectRatio":false,"scales":{"y":{"ticks":{"precision":0,"autoSkip":false,"font":{"family":"Montserrat"},"color":"#000000"},"stacked":false},"x":{"ticks":{"stepSize":null,"precision":0,"font":{"family":"Montserrat"},"color":"#000000"},"stacked":false}},"plugins":{"legend":{"position":"top","labels":{"font":{"family":"Montserrat"},"color":"#000000"}},"title":{"display":true,"text":"Journals","font":{"size":24,"family":"Montserrat","weight":600},"color":"#000000"}}}},"openAccess":{"type":"pie","data":{"show":true,"labels":["Closed access","Open access"],"datasets":[{"label":"Open access","data":[719,301],"backgroundColor":["#E5E7EB","#f68212"],"hoverOffset":4,"percentage":[70.49,29.51]}]},"options":{"plugins":{"legend":{"position":"top","labels":{"font":{"family":"Montserrat"},"color":"#000000"}},"title":{"display":true,"text":"Open access","font":{"size":24,"family":"Montserrat","weight":600},"color":"#000000"}}}},"publishers":{"type":"bar","data":{"show":true,"labels":["Springer Nature","Elsevier","Multidisciplinary Digital Publishing Institute (MDPI)","Taylor & Francis","Wiley","Publishing House for Science and Technology, Vietnam Academy of Science and Technology (Publications)","IOP Publishing","EDP Sciences","Hindawi Limited","Oxford University Press","IEEE","SAGE","National University of Science & Technology (MISiS)","American Chemical Society (ACS)","World Scientific","Royal Society of Chemistry (RSC)","Copernicus","Thomas Telford","IWA Publishing","Walter de Gruyter","Pleiades Publishing","Chinese Academy of Sciences","Trans Tech Publications","Frontiers Media S.A.","American Institute of Physics (AIP)","International Union of Crystallography (IUCr)","American Physical Society (APS)","Chinese Society of Rare Earths","Diponegoro university Indonesia - Center of Biomass and Renewable Energy (CBIORE)","Institution of Chemical Engineers"],"ids":[8,17,202,18,11,7297,2075,76,6921,19,6953,31,7232,40,23,123,3207,6981,1283,4,101,1837,104,208,250,730,1539,3836,4969,5735],"codes":[0,0,0,0,0,0,0,0,0,0,0,0,0,0,0,0,0,0,0,0,0,0,0,0,0,0,0,0,0,0],"imageUrls":["\/storage\/images\/resized\/voXLqlsvTwv5p3iMQ8Dhs95nqB4AXOG7Taj7G4ra_medium.webp","\/storage\/images\/resized\/GDnYOu1UpMMfMMRV6Aqle4H0YLLsraeD9IP9qScG_medium.webp","\/storage\/images\/resized\/MjH1ITP7lMYGxeqUZfkt2BnVLgjkk413jwBV97XX_medium.webp","\/storage\/images\/resized\/5YZtvLvkPZuc2JHOaZsjCvGSHFCuC3drUwN3YAc5_medium.webp","\/storage\/images\/resized\/bRyGpdm98BkAUYiK1YFNpl5Z7hPu6Gd87gbIeuG3_medium.webp","\/storage\/images\/resized\/aaZxju90lOnC0t1v0UdT44gk6x3DjeOtYm5Cj61p_medium.webp","\/storage\/images\/resized\/LsKy6OnmmmRGcAU6CZgWQvNiP1polbaSLNrN7zqj_medium.webp","\/storage\/images\/resized\/7GcDgL5Sa2zewDk4sRy4fs0GznbYZIsSV9wqMs28_medium.webp","\/storage\/images\/resized\/hqfzhQAjTGlNSRs6yzFNITgjSMm9Jr2QuotJHIvE_medium.webp","\/storage\/images\/resized\/yNSijlgQghQF53VZuyFLA30CKDe4j3HK74Vtpnxa_medium.webp","\/storage\/images\/resized\/6scCJegesojp2jubwY3uKCzTAmgsaH2GIFlg6Hfk_medium.webp","\/storage\/images\/resized\/ruydfaB80LDjlkYqsfOeUAZohOIODyq7bQzis5O7_medium.webp","\/storage\/images\/resized\/dRvP3lMJcjXP3GTJzfOCMGs4rAy4Aq1F3yWpmEzN_medium.webp","\/storage\/images\/resized\/iLiQsFqFaSEx6chlGQ5fbAwF6VYU3WWa08hkss0g_medium.webp","\/storage\/images\/resized\/jZQkhOOcY9wrGwZm7XGRwMe8H7fThQu3KamISuun_medium.webp","\/storage\/images\/resized\/leiAYcRDGTSl5B1eCnwpSGqmDEUEfDPPoYisFGhT_medium.webp","\/storage\/images\/resized\/cxBZptDQQvWgQeXj5GoTXDfgUEoGVvFgpDRctnDr_medium.webp","\/storage\/images\/resized\/ljAuhpSMREOSIGmvHXfCUKFQbLqQkW0Ce2oBFgc8_medium.webp","\/storage\/images\/resized\/uGx6c1W3cHqbKXWIuICsBj3302lg4GbPWL7ng21d_medium.webp","\/storage\/images\/resized\/3SpVxcYL33bOvPq4sHxJLH2NeKNeDloahSUpNiO4_medium.webp","\/storage\/images\/resized\/oZgeErrVFhuDksyqFURLvYS1wtVSBWczh001igGo_medium.webp","\/storage\/images\/resized\/xZL5jEVSjdrNMvIKGWHz7ACmqdmtuMd9Aj5EzxgN_medium.webp","\/storage\/images\/resized\/lZMRu5MhhE0HtEBGlENti9uMMEivcrzFOV6rqpGN_medium.webp","\/storage\/images\/resized\/4QWA67eqfcfyOiA8Wk7YnqroHFqQbTsmDJUYTCTg_medium.webp","\/storage\/images\/resized\/ARM4e6URKRsbRZvIF0vFis9DjxGloBjnBYJXbHmZ_medium.webp","\/storage\/images\/resized\/xG3oC19920mmFVqkoVEGKGaMCeoalzyvWxPkVox5_medium.webp","\/storage\/images\/resized\/nrK64iXHTzj43wMrfN1ZoUQ0vanswGzWPN45K3jA_medium.webp","\/storage\/images\/resized\/qSRMPRcU9e305ZndYbSqzvtlXr55B5FcWpS97I4M_medium.webp","","\/storage\/images\/resized\/MTi1s8E8zKDDF3SWt8DobBiteEPA40WPDiKEYgvd_medium.webp"],"datasets":[{"label":"","data":[304,244,86,64,49,41,31,19,19,14,11,9,9,8,6,6,6,6,5,4,4,4,3,3,3,3,3,3,3,3],"backgroundColor":["#3B82F6","#3B82F6","#3B82F6","#3B82F6","#3B82F6","#3B82F6","#3B82F6","#3B82F6","#3B82F6","#3B82F6","#3B82F6","#3B82F6","#3B82F6","#3B82F6","#3B82F6","#3B82F6","#3B82F6","#3B82F6","#3B82F6","#3B82F6","#3B82F6","#3B82F6","#3B82F6","#3B82F6","#3B82F6","#3B82F6","#3B82F6","#3B82F6","#3B82F6","#3B82F6"],"percentage":[29.8,23.92,8.43,6.27,4.8,4.02,3.04,1.86,1.86,1.37,1.08,0.88,0.88,0.78,0.59,0.59,0.59,0.59,0.49,0.39,0.39,0.39,0.29,0.29,0.29,0.29,0.29,0.29,0.29,0.29],"barThickness":13}]},"options":{"indexAxis":"y","maintainAspectRatio":false,"scales":{"y":{"ticks":{"precision":0,"autoSkip":false,"font":{"family":"Montserrat"},"color":"#000000"},"stacked":false},"x":{"ticks":{"stepSize":null,"precision":0,"font":{"family":"Montserrat"},"color":"#000000"},"stacked":false}},"plugins":{"legend":{"position":"top","labels":{"font":{"family":"Montserrat"},"color":"#000000"}},"title":{"display":true,"text":"Publishers","font":{"size":24,"family":"Montserrat","weight":600},"color":"#000000"}}}},"quartiles":{"type":"pie","data":{"show":true,"labels":["Quartile not defined","Q1","Q2","Q3","Q4"],"datasets":[{"label":"Quartiles by SCImago","data":[103,452,250,110,105],"backgroundColor":["#E5E7EB","rgb(164, 207, 99)","rgb(232, 213, 89)","rgb(251, 163,83)","rgb(221,90,78)"],"hoverOffset":4,"percentage":[10.1,44.31,24.51,10.78,10.29]}]},"options":{"plugins":{"legend":{"position":"top","labels":{"font":{"family":"Montserrat"},"color":"#000000"}},"title":{"display":true,"text":"Quartiles by SCImago","font":{"size":24,"family":"Montserrat","weight":600},"color":"#000000"}}}},"quartilesWs":{"type":"pie","data":{"show":true,"labels":["Quartile not defined","Q1","Q2","Q3","Q4"],"datasets":[{"label":"Quartiles by WoS","data":[326,302,241,114,37],"backgroundColor":["#E5E7EB","rgb(164, 207, 99)","rgb(232, 213, 89)","rgb(251, 163,83)","rgb(221,90,78)"],"hoverOffset":4,"percentage":[31.96,29.61,23.63,11.18,3.63]}]},"options":{"plugins":{"legend":{"position":"top","labels":{"font":{"family":"Montserrat"},"color":"#000000"}},"title":{"display":true,"text":"Quartiles by WoS","font":{"size":24,"family":"Montserrat","weight":600},"color":"#000000"}}}},"quartilesByYears":{"type":"bar","data":{"show":true,"labels":[1994,1995,1996,1997,1998,1999,2000,2001,2002,2003,2004,2005,2006,2007,2008,2009,2010,2011,2012,2013,2014,2015,2016,2017,2018,2019,2020,2021,2022,2023,2024],"ids":[],"codes":[],"imageUrls":[],"datasets":[{"label":"Q4","backgroundColor":"rgb(221,90,78)","data":[0,0,0,0,0,0,0,0,0,0,0,0,0,0,0,0,0,1,0,0,0,1,0,5,3,7,32,10,11,34,1],"percentage":[0,0,0,0,0,0,0,0,0,0,0,0,0,0,0,0,0,7.69,0,0,0,3.13,0,13.16,3.19,5.22,23.36,6.25,9.91,26.15,2.17]},{"label":"Q3","backgroundColor":"rgb(251, 163,83)","data":[0,0,0,0,0,0,0,0,0,0,0,1,0,0,0,0,0,0,2,3,2,3,6,7,16,14,15,17,11,8,5],"percentage":[0,0,0,0,0,0,0,0,0,0,0,50,0,0,0,0,0,0,10.53,18.75,11.11,9.38,13.95,18.42,17.02,10.45,10.95,10.63,9.91,6.15,10.87]},{"label":"Q2","backgroundColor":"rgb(232, 213, 89)","data":[1,0,0,0,1,0,1,0,0,0,0,1,0,0,3,1,0,3,5,4,3,7,13,8,27,27,23,41,35,31,15],"percentage":[100,0,0,0,100,0,100,0,0,0,0,50,0,0,50,20,0,23.08,26.32,25,16.67,21.88,30.23,21.05,28.72,20.15,16.79,25.63,31.53,23.85,32.61]},{"label":"Q1","backgroundColor":"rgb(164, 207, 99)","data":[0,1,0,0,0,0,0,0,0,1,0,0,1,3,3,3,4,9,10,6,10,18,18,15,35,75,59,79,42,40,20],"percentage":[0,100,0,0,0,0,0,0,0,50,0,0,50,100,50,60,100,69.23,52.63,37.5,55.56,56.25,41.86,39.47,37.23,55.97,43.07,49.38,37.84,30.77,43.48]},{"label":"Quartile not defined","backgroundColor":"#E5E7EB","data":[0,0,0,0,0,1,0,0,0,1,0,0,1,0,0,1,0,0,2,3,3,3,6,3,13,11,8,13,12,17,5],"percentage":[0,0,0,0,0,100,0,0,0,50,0,0,50,0,0,20,0,0,10.53,18.75,16.67,9.38,13.95,7.89,13.83,8.21,5.84,8.13,10.81,13.08,10.87]}]},"options":{"indexAxis":"x","maintainAspectRatio":true,"scales":{"y":{"ticks":{"precision":0,"autoSkip":false,"font":{"family":"Montserrat"},"color":"#000000"},"stacked":true},"x":{"ticks":{"stepSize":1,"precision":0,"font":{"family":"Montserrat"},"color":"#000000"},"stacked":true}},"plugins":{"legend":{"position":"top","labels":{"font":{"family":"Montserrat"},"color":"#000000"}},"title":{"display":true,"text":"Quartiles by SCImago per year","font":{"size":24,"family":"Montserrat","weight":600},"color":"#000000"}}}},"quartilesWsByYears":{"type":"bar","data":{"show":true,"labels":[1994,1995,1996,1997,1998,1999,2000,2001,2002,2003,2004,2005,2006,2007,2008,2009,2010,2011,2012,2013,2014,2015,2016,2017,2018,2019,2020,2021,2022,2023,2024],"ids":[],"codes":[],"imageUrls":[],"datasets":[{"label":"Q4","backgroundColor":"rgb(221,90,78)","data":[0,0,0,0,0,1,0,0,0,0,0,1,0,0,0,0,0,1,0,0,2,2,2,3,3,5,3,7,2,2,3],"percentage":[0,0,0,0,0,100,0,0,0,0,0,50,0,0,0,0,0,7.69,0,0,11.11,6.25,4.65,7.89,3.19,3.73,2.19,4.38,1.8,1.54,6.52]},{"label":"Q3","backgroundColor":"rgb(251, 163,83)","data":[1,0,0,0,1,0,1,0,0,0,0,1,0,0,0,1,1,0,1,3,1,2,6,8,11,14,16,19,12,8,7],"percentage":[100,0,0,0,100,0,100,0,0,0,0,50,0,0,0,20,25,0,5.26,18.75,5.56,6.25,13.95,21.05,11.7,10.45,11.68,11.88,10.81,6.15,15.22]},{"label":"Q2","backgroundColor":"rgb(232, 213, 89)","data":[0,0,0,0,0,0,0,0,0,1,0,0,2,1,5,0,0,6,9,4,6,9,7,9,23,31,21,38,29,27,13],"percentage":[0,0,0,0,0,0,0,0,0,50,0,0,100,33.33,83.33,0,0,46.15,47.37,25,33.33,28.13,16.28,23.68,24.47,23.13,15.33,23.75,26.13,20.77,28.26]},{"label":"Q1","backgroundColor":"rgb(164, 207, 99)","data":[0,1,0,0,0,0,0,0,0,0,0,0,0,2,1,3,3,5,5,3,6,14,16,8,21,49,44,53,28,28,12],"percentage":[0,100,0,0,0,0,0,0,0,0,0,0,0,66.67,16.67,60,75,38.46,26.32,18.75,33.33,43.75,37.21,21.05,22.34,36.57,32.12,33.13,25.23,21.54,26.09]},{"label":"Quartile not defined","backgroundColor":"#E5E7EB","data":[0,0,0,0,0,0,0,0,0,1,0,0,0,0,0,1,0,1,4,6,3,5,12,10,36,35,53,43,40,65,11],"percentage":[0,0,0,0,0,0,0,0,0,50,0,0,0,0,0,20,0,7.69,21.05,37.5,16.67,15.63,27.91,26.32,38.3,26.12,38.69,26.88,36.04,50,23.91]}]},"options":{"indexAxis":"x","maintainAspectRatio":true,"scales":{"y":{"ticks":{"precision":0,"autoSkip":false,"font":{"family":"Montserrat"},"color":"#000000"},"stacked":true},"x":{"ticks":{"stepSize":1,"precision":0,"font":{"family":"Montserrat"},"color":"#000000"},"stacked":true}},"plugins":{"legend":{"position":"top","labels":{"font":{"family":"Montserrat"},"color":"#000000"}},"title":{"display":true,"text":"Quartiles by WoS per year","font":{"size":24,"family":"Montserrat","weight":600},"color":"#000000"}}}},"subjects":{"type":"bar","data":{"show":true,"labels":["Geology","General Materials Science","Geotechnical Engineering and Engineering Geology","General Chemistry","Pollution","General Medicine","Electrical and Electronic Engineering","Civil and Structural Engineering","General Engineering","Geochemistry and Petrology","General Earth and Planetary Sciences","Computer Science Applications","Building and Construction","Environmental Chemistry","Water Science and Technology","Geophysics","General Chemical Engineering","General Environmental Science","Earth-Surface Processes","Biochemistry","Condensed Matter Physics","Analytical Chemistry","Electronic, Optical and Magnetic Materials","Software","Instrumentation","Industrial and Manufacturing Engineering","Health, Toxicology and Mutagenesis","Atomic and Molecular Physics, and Optics","Mechanics of Materials","Process Chemistry and Technology"],"ids":[204,50,195,4,98,20,53,116,70,82,212,17,115,80,141,172,33,111,168,10,49,37,6,133,59,61,96,48,72,47],"codes":[0,0,0,0,0,0,0,0,0,0,0,0,0,0,0,0,0,0,0,0,0,0,0,0,0,0,0,0,0,0],"imageUrls":["","","","","","","","","","","","","","","","","","","","","","","","","","","","","",""],"datasets":[{"label":"","data":[104,90,87,62,62,61,61,59,57,57,55,49,47,45,45,42,41,41,41,39,39,37,34,34,33,32,32,31,30,29],"backgroundColor":["#3B82F6","#3B82F6","#3B82F6","#3B82F6","#3B82F6","#3B82F6","#3B82F6","#3B82F6","#3B82F6","#3B82F6","#3B82F6","#3B82F6","#3B82F6","#3B82F6","#3B82F6","#3B82F6","#3B82F6","#3B82F6","#3B82F6","#3B82F6","#3B82F6","#3B82F6","#3B82F6","#3B82F6","#3B82F6","#3B82F6","#3B82F6","#3B82F6","#3B82F6","#3B82F6"],"percentage":[10.2,8.82,8.53,6.08,6.08,5.98,5.98,5.78,5.59,5.59,5.39,4.8,4.61,4.41,4.41,4.12,4.02,4.02,4.02,3.82,3.82,3.63,3.33,3.33,3.24,3.14,3.14,3.04,2.94,2.84],"barThickness":13}]},"options":{"indexAxis":"y","maintainAspectRatio":false,"scales":{"y":{"ticks":{"precision":0,"autoSkip":false,"font":{"family":"Montserrat"},"color":"#000000"},"stacked":false},"x":{"ticks":{"stepSize":null,"precision":0,"font":{"family":"Montserrat"},"color":"#000000"},"stacked":false}},"plugins":{"legend":{"position":"top","labels":{"font":{"family":"Montserrat"},"color":"#000000"}},"title":{"display":true,"text":"Fields of science","font":{"size":24,"family":"Montserrat","weight":600},"color":"#000000"}}}},"yearsArticles":{"type":"bar","data":{"show":true,"labels":[1994,1995,1996,1997,1998,1999,2000,2001,2002,2003,2004,2005,2006,2007,2008,2009,2010,2011,2012,2013,2014,2015,2016,2017,2018,2019,2020,2021,2022,2023,2024],"ids":[0,0,0,0,0,0,0,0,0,0,0,0,0,0,0,0,0,0,0,0,0,0,0,0,0,0,0,0,0,0,0],"codes":[0,0,0,0,0,0,0,0,0,0,0,0,0,0,0,0,0,0,0,0,0,0,0,0,0,0,0,0,0,0,0],"imageUrls":["","","","","","","","","","","","","","","","","","","","","","","","","","","","","","",""],"datasets":[{"label":"Articles number","data":[1,1,0,0,1,1,1,0,0,2,0,2,2,3,6,5,4,13,19,16,18,32,43,38,94,134,137,160,111,130,46],"backgroundColor":["#3B82F6","#3B82F6","#3B82F6","#3B82F6","#3B82F6","#3B82F6","#3B82F6","#3B82F6","#3B82F6","#3B82F6","#3B82F6","#3B82F6","#3B82F6","#3B82F6","#3B82F6","#3B82F6","#3B82F6","#3B82F6","#3B82F6","#3B82F6","#3B82F6","#3B82F6","#3B82F6","#3B82F6","#3B82F6","#3B82F6","#3B82F6","#3B82F6","#3B82F6","#3B82F6","#3B82F6"],"percentage":[0.1,0.1,0,0,0.1,0.1,0.1,0,0,0.2,0,0.2,0.2,0.29,0.59,0.49,0.39,1.27,1.86,1.57,1.76,3.14,4.22,3.73,9.22,13.14,13.43,15.69,10.88,12.75,4.51],"barThickness":null}]},"options":{"indexAxis":"x","maintainAspectRatio":true,"scales":{"y":{"ticks":{"precision":0,"autoSkip":false,"font":{"family":"Montserrat"},"color":"#000000"},"stacked":false},"x":{"ticks":{"stepSize":1,"precision":0,"font":{"family":"Montserrat"},"color":"#000000"},"stacked":false}},"plugins":{"legend":{"position":"top","labels":{"font":{"family":"Montserrat"},"color":"#000000"}},"title":{"display":true,"text":"Articles per year","font":{"size":24,"family":"Montserrat","weight":600},"color":"#000000"}}}},"yearsCitations":{"type":"bar","data":{"show":true,"labels":[1995,1996,1997,1998,1999,2000,2001,2002,2003,2004,2005,2006,2007,2008,2009,2010,2011,2012,2013,2014,2015,2016,2017,2018,2019,2020,2021,2022,2023,2024,2025],"ids":[0,0,0,0,0,0,0,0,0,0,0,0,0,0,0,0,0,0,0,0,0,0,0,0,0,0,0,0,0,0,0],"codes":[0,0,0,0,0,0,0,0,0,0,0,0,0,0,0,0,0,0,0,0,0,0,0,0,0,0,0,0,0,0,0],"imageUrls":["","","","","","","","","","","","","","","","","","","","","","","","","","","","","","",""],"datasets":[{"label":"Citations number","data":[1,0,0,1,0,1,1,0,1,0,5,5,11,32,43,60,85,100,126,246,247,417,604,915,1752,2153,3094,3707,3645,2129,1],"backgroundColor":["#3B82F6","#3B82F6","#3B82F6","#3B82F6","#3B82F6","#3B82F6","#3B82F6","#3B82F6","#3B82F6","#3B82F6","#3B82F6","#3B82F6","#3B82F6","#3B82F6","#3B82F6","#3B82F6","#3B82F6","#3B82F6","#3B82F6","#3B82F6","#3B82F6","#3B82F6","#3B82F6","#3B82F6","#3B82F6","#3B82F6","#3B82F6","#3B82F6","#3B82F6","#3B82F6","#3B82F6"],"percentage":[0.01,0,0,0.01,0,0.01,0.01,0,0.01,0,0.03,0.03,0.06,0.16,0.22,0.31,0.44,0.52,0.65,1.27,1.27,2.15,3.11,4.72,9.03,11.1,15.95,19.11,18.79,10.98,0.01],"barThickness":null}]},"options":{"indexAxis":"x","maintainAspectRatio":true,"scales":{"y":{"ticks":{"precision":0,"autoSkip":false,"font":{"family":"Montserrat"},"color":"#000000"},"stacked":false},"x":{"ticks":{"stepSize":1,"precision":0,"font":{"family":"Montserrat"},"color":"#000000"},"stacked":false}},"plugins":{"legend":{"position":"top","labels":{"font":{"family":"Montserrat"},"color":"#000000"}},"title":{"display":true,"text":"Citations per year","font":{"size":24,"family":"Montserrat","weight":600},"color":"#000000"}}}},"countries":{"type":"bar","data":{"show":true,"labels":["China","France","Republic of Korea","Norway","Russia","Germany","Australia","USA","United Kingdom","Japan","India","Malaysia","Poland","Iran","Italy","Nigeria","Denmark","Austria","Canada","Hungary","Belgium","Romania","Spain","Thailand","Sweden","South Africa","Brazil","Greece","Egypt","Pakistan"],"ids":[9,3,175,153,1,2,11,7,48,250,84,129,173,88,92,147,74,12,97,49,32,178,91,207,238,247,40,71,79,166],"codes":["cn","fr","kr","no","ru","de","au","us","gb","jp","in","my","pl","ir","it","ng","dk","at","ca","hu","be","ro","es","th","se","za","br","gr","eg","pk"],"imageUrls":["","","","","","","","","","","","","","","","","","","","","","","","","","","","","",""],"datasets":[{"label":"","data":[137,92,89,54,50,48,44,40,39,39,33,29,29,25,25,21,19,17,16,15,12,11,10,9,8,7,6,6,6,6],"backgroundColor":["#3B82F6","#3B82F6","#3B82F6","#3B82F6","#3B82F6","#3B82F6","#3B82F6","#3B82F6","#3B82F6","#3B82F6","#3B82F6","#3B82F6","#3B82F6","#3B82F6","#3B82F6","#3B82F6","#3B82F6","#3B82F6","#3B82F6","#3B82F6","#3B82F6","#3B82F6","#3B82F6","#3B82F6","#3B82F6","#3B82F6","#3B82F6","#3B82F6","#3B82F6","#3B82F6"],"percentage":[13.43,9.02,8.73,5.29,4.9,4.71,4.31,3.92,3.82,3.82,3.24,2.84,2.84,2.45,2.45,2.06,1.86,1.67,1.57,1.47,1.18,1.08,0.98,0.88,0.78,0.69,0.59,0.59,0.59,0.59],"barThickness":13}]},"options":{"indexAxis":"y","maintainAspectRatio":false,"scales":{"y":{"ticks":{"precision":0,"autoSkip":false,"font":{"family":"Montserrat"},"color":"#000000"},"stacked":false},"x":{"ticks":{"stepSize":null,"precision":0,"font":{"family":"Montserrat"},"color":"#000000"},"stacked":false}},"plugins":{"legend":{"position":"top","labels":{"font":{"family":"Montserrat"},"color":"#000000"}},"title":{"display":true,"text":"With other countries","font":{"size":24,"family":"Montserrat","weight":600},"color":"#000000"}}}},"organizations":{"type":"bar","data":{"show":true,"labels":["Vietnam Academy of Science and Technology","Duy Tan University","Ton Duc Thang University","VNU University of Science","Vietnam National University, Hanoi","Hanoi University of Science and Technology","Hanoi University of Natural Resources and Environment","Hanoi National University of Education","Grenoble Alpes University","University of South-Eastern Norway","Sejong University","Institute for Tropical Technology","Le Quy Don Technical University","Michael Okpara University of Agriculture","Hanoi University","Hue University","Tongji University","Central South University","Curtin University","University of Bristol","Institute of Marine Geology and Geophysics","University of Technology, Malaysia","Geological Survey of Denmark and Greenland","University of Pannonia","Saint Petersburg Mining University","Moscow State University of Civil Engineering","Pukyong National University","Vietnam National University Ho Chi Minh City","Thai Nguyen University","Vietnam Maritime University"],"ids":[2071,2015,2014,2086,2074,2085,2070,2099,2717,3677,4380,2109,2117,4227,2091,2108,2327,2667,3948,5249,2102,2588,3313,4924,230,250,4605,2034,2051,2080],"codes":[0,0,0,0,0,0,0,0,0,0,0,0,0,0,0,0,0,0,0,0,0,0,0,0,0,0,0,0,0,0],"imageUrls":["\/storage\/images\/resized\/aYpr5j5n6aaZBjE1i2ffijELRX7FKyys5cw75dIc_medium.webp","\/storage\/images\/resized\/cW8xlgazQzZ45VDBKDedEPABUavTitMRyFSHE56X_medium.webp","\/storage\/images\/resized\/cMqOyPGlwAJVc11A2bz6YsBxmLGNN8nLGY9GX3pe_medium.webp","\/storage\/images\/resized\/MUz9zFlxdpN8bwCwjGGI4ad9BMTGvWv2wWvU9ReL_medium.webp","\/storage\/images\/resized\/vn4HRp78lo9MBjFJsU6G2JSQ2WrJSzDjEmF2cSjj_medium.webp","\/storage\/images\/resized\/jvoHyiBSXVOaATOu7DCIK9vF7ec2ABnLIZyoCfDO_medium.webp","\/storage\/images\/resized\/qiYzPhAM4wHx9ABkKo87vqSpW5bDAPyHPTxSaq3Q_medium.webp","\/storage\/images\/resized\/PGLMKHgsX4qVVl3JJthN6pIXIC3kh85lSAWlPHBA_medium.webp","\/storage\/images\/resized\/sH1bSRYr9QhGc0Le5WYcAOb8DDkyZLCip0vwUyJr_medium.webp","\/storage\/images\/resized\/3RnXlEGliP5OyuVyXobkNh37B0ttH8Zhhw3B9Hn7_medium.webp","\/storage\/images\/resized\/8yW61qRtdQRnlgSmERKEXCoAhpHlkPtPXrL3TRfi_medium.webp","\/storage\/images\/resized\/iSssUz61PNHAnQRxVo0VgSbnfrdofQ2pRVXMcdBw_medium.webp","\/storage\/images\/resized\/HJhgDWJmfmNteBJvvBSYLh3tcId5elF4XnJg6JFu_medium.webp","\/storage\/images\/resized\/IaDvx0M9da0r6KMIAMy4EBfVTM4hZzbEAulMpvIT_medium.webp","\/storage\/images\/resized\/94WWtrLoIfCqTbVA9GV8xWW12Qn1lhwRcWIlwhp3_medium.webp","\/storage\/images\/resized\/V3MFUjkLf5Ii4LCDYyaBE9pd61RNXtWmxvc8wML2_medium.webp","\/storage\/images\/resized\/gZJVE1MCeZjecYxbxh0l2W1pL1WycWr9whS2UMjD_medium.webp","\/storage\/images\/resized\/HXRQ7mwmj29qm6JxmSbdUXOO3p5LIRyHc6poIMgo_medium.webp","\/storage\/images\/resized\/9ixugvniShqsfhUc27joFQ7y7KpTLJ2EfgpLagww_medium.webp","\/storage\/images\/resized\/sHTOJfsRuYHaJ2T7sXmbMfCclIzNbm6cbEClZ8If_medium.webp","\/storage\/images\/resized\/q2Cy6l8OCsbn5Y7h642DzTHFy3Bbg8UZHu5TrVPz_medium.webp","\/storage\/images\/resized\/72vlBBbmKqN6MdU9JdrhG5BQk3189DyqRzYYekDJ_medium.webp","\/storage\/images\/resized\/eBGD6YDIGqTMtZbrhlutIjspLi2uBaTdBaFtZH74_medium.webp","\/storage\/images\/resized\/6PNess7jUTHStbM7TwkEEA5nYci8jYMqGm8UVMSx_medium.webp","\/storage\/images\/resized\/jlKB1H49BHz9OyDCoJhgy9YQTP0iyIL19UNv0Wfu_medium.webp","\/storage\/images\/resized\/gS8yxPauS4XQtnMfZdATOX1ntQ0yHAbJxXNYDqaa_medium.webp","\/storage\/images\/resized\/wvA2SA4SQiB3Dr35VPrM2IqMjZSPAjGZInemMn5t_medium.webp","\/storage\/images\/resized\/bt31ke7DVdclPmhmbKbgFQujhSZH8IXLk4LdHjgj_medium.webp","\/storage\/images\/resized\/1Ms7MBV2G3rakiLJrONuvZISWCEPwKhaktdYUbpD_medium.webp","\/storage\/images\/resized\/CxjcndaEtWvlwU7t4JIdqRrt8d65dAOhT7ITBmFi_medium.webp"],"datasets":[{"label":"","data":[198,122,65,57,46,40,39,37,35,28,24,20,20,19,18,18,17,17,16,16,15,15,15,15,14,14,14,12,12,12],"backgroundColor":["#3B82F6","#3B82F6","#3B82F6","#3B82F6","#3B82F6","#3B82F6","#3B82F6","#3B82F6","#3B82F6","#3B82F6","#3B82F6","#3B82F6","#3B82F6","#3B82F6","#3B82F6","#3B82F6","#3B82F6","#3B82F6","#3B82F6","#3B82F6","#3B82F6","#3B82F6","#3B82F6","#3B82F6","#3B82F6","#3B82F6","#3B82F6","#3B82F6","#3B82F6","#3B82F6"],"percentage":[19.41,11.96,6.37,5.59,4.51,3.92,3.82,3.63,3.43,2.75,2.35,1.96,1.96,1.86,1.76,1.76,1.67,1.67,1.57,1.57,1.47,1.47,1.47,1.47,1.37,1.37,1.37,1.18,1.18,1.18],"barThickness":13}],"rorIds":{"2071":"02wsd5p50","2015":"05ezss144","2014":"01drq0835","2086":"05w54hk79","2074":"02jmfj006","2085":"04nyv3z04","2070":"00gxs8147","2099":"0360g3z42","2717":"02rx3b187","3677":"05ecg5h20","4380":"00aft1q37","2109":"00tp4wm41","2117":"04wgyjv21","4227":"050850526","2091":"01mxx0e62","2108":"00qaa6j11","2327":"03rc6as71","2667":"00f1zfq44","3948":"02n415q13","5249":"0524sp257","2102":"057as5086","2588":"026w31v75","3313":"01b40r146","4924":"03y5egs41","230":"01ma17032","250":"00em04n91","4605":"0433kqc49","2034":"00waaqh38","2051":"02128gy91","2080":"03z9fzp04"}},"options":{"indexAxis":"y","maintainAspectRatio":false,"scales":{"y":{"ticks":{"precision":0,"autoSkip":false,"font":{"family":"Montserrat"},"color":"#000000"},"stacked":false},"x":{"ticks":{"stepSize":null,"precision":0,"font":{"family":"Montserrat"},"color":"#000000"},"stacked":false}},"plugins":{"legend":{"position":"top","labels":{"font":{"family":"Montserrat"},"color":"#000000"}},"title":{"display":true,"text":"With other organizations","font":{"size":24,"family":"Montserrat","weight":600},"color":"#000000"}}}},"foreignOrganizations":{"type":"bar","data":{"show":true,"labels":["Grenoble Alpes University","University of South-Eastern Norway","Sejong University","Michael Okpara University of Agriculture","Tongji University","Central South University","Curtin University","University of Bristol","University of Technology, Malaysia","Geological Survey of Denmark and Greenland","University of Pannonia","Saint Petersburg Mining University","Moscow State University of Civil Engineering","Pukyong National University","Norwegian University of Life Sciences","Chonnam National University","Polytechnic University of Turin","Yonsei University","University Putra Malaysia","China University of Geosciences (Beijing)","China University of Geosciences (Wuhan)","Vienna University of Technology","China University of Mining and Technology","Norwegian University of Science and Technology","Technical University of Denmark","Hefei University of Technology","Tarbiat Modares University","University of Technology Sydney","Helmholtz-Zentrum Dresden-Rossendorf","Helmholtz Institute Freiberg for Resource Technology"],"ids":[2717,3677,4380,4227,2327,2667,3948,5249,2588,3313,4924,230,250,4605,3537,4427,3102,4330,2585,2798,2799,4753,2497,3127,3272,3476,1319,3029,3265,5292],"codes":[0,0,0,0,0,0,0,0,0,0,0,0,0,0,0,0,0,0,0,0,0,0,0,0,0,0,0,0,0,0],"imageUrls":["\/storage\/images\/resized\/sH1bSRYr9QhGc0Le5WYcAOb8DDkyZLCip0vwUyJr_medium.webp","\/storage\/images\/resized\/3RnXlEGliP5OyuVyXobkNh37B0ttH8Zhhw3B9Hn7_medium.webp","\/storage\/images\/resized\/8yW61qRtdQRnlgSmERKEXCoAhpHlkPtPXrL3TRfi_medium.webp","\/storage\/images\/resized\/IaDvx0M9da0r6KMIAMy4EBfVTM4hZzbEAulMpvIT_medium.webp","\/storage\/images\/resized\/gZJVE1MCeZjecYxbxh0l2W1pL1WycWr9whS2UMjD_medium.webp","\/storage\/images\/resized\/HXRQ7mwmj29qm6JxmSbdUXOO3p5LIRyHc6poIMgo_medium.webp","\/storage\/images\/resized\/9ixugvniShqsfhUc27joFQ7y7KpTLJ2EfgpLagww_medium.webp","\/storage\/images\/resized\/sHTOJfsRuYHaJ2T7sXmbMfCclIzNbm6cbEClZ8If_medium.webp","\/storage\/images\/resized\/72vlBBbmKqN6MdU9JdrhG5BQk3189DyqRzYYekDJ_medium.webp","\/storage\/images\/resized\/eBGD6YDIGqTMtZbrhlutIjspLi2uBaTdBaFtZH74_medium.webp","\/storage\/images\/resized\/6PNess7jUTHStbM7TwkEEA5nYci8jYMqGm8UVMSx_medium.webp","\/storage\/images\/resized\/jlKB1H49BHz9OyDCoJhgy9YQTP0iyIL19UNv0Wfu_medium.webp","\/storage\/images\/resized\/gS8yxPauS4XQtnMfZdATOX1ntQ0yHAbJxXNYDqaa_medium.webp","\/storage\/images\/resized\/wvA2SA4SQiB3Dr35VPrM2IqMjZSPAjGZInemMn5t_medium.webp","\/storage\/images\/resized\/XJuoA7jS05be4jXanvDCqpHyEnMHizLHDAHC0LMi_medium.webp","\/storage\/images\/resized\/tqXapyrpQkyRwR3vkYHvuFbjeusfvVX4I07YAexl_medium.webp","\/storage\/images\/resized\/LqCVasUSVZcjbYTfF7pjyZWWvp9MqKxUL2b17OCq_medium.webp","\/storage\/images\/resized\/dqShm2NSO54GwyEz5OPi5QgwnKyuVuASAZ2uoDpp_medium.webp","\/storage\/images\/resized\/yL65V3eZkCkaT7Nzm2MFVXIMbG75hT9bdTgUU4xD_medium.webp","\/storage\/images\/resized\/nXz4Xlgl3Mki9Y2nzp9ezITNaEmhKPBjmVfSsHXb_medium.webp","\/storage\/images\/resized\/L7Cpsz0eZEsb3kt8LISUIt40escT0KWPnqNNzYkp_medium.webp","\/storage\/images\/resized\/8hc5kVcnhtvGxD7o2yso9q5RbTchXtI3Tidha1N7_medium.webp","\/storage\/images\/resized\/KrtecedrLgm6F6k2ViVHWcw0bE610leDQwwU82pJ_medium.webp","\/storage\/images\/resized\/GQg8uUJ7j0ZUSPRJhH0xxAvArGQLptFdzWXy3cRh_medium.webp","\/storage\/images\/resized\/XrSWMbmkMP2MgzryQ4wCllpVgoSltxAsDuEjt4ck_medium.webp","\/storage\/images\/resized\/KoeuMlbu6ydoktzGhrCzPoVyyFZx5vmcYQ1DPjqH_medium.webp","\/storage\/images\/resized\/JYdppbKAHIiD9BqHmU6bmLPIaigEY12caCmQZEhA_medium.webp","\/storage\/images\/resized\/P47RAUqymEp94Gc7QA1uiXtgCYYYUoQpQxX97tIL_medium.webp","\/storage\/images\/resized\/kn4TiGTkaFCCVZJY7VyfWeTdqbiXjHJNQFTJlKIF_medium.webp","\/storage\/images\/resized\/0AsYEbjtI6YWlMGM8EkX0a53mLW7XSg2cwzHRkYp_medium.webp"],"datasets":[{"label":"","data":[35,28,24,19,17,17,16,16,15,15,15,14,14,14,12,12,11,11,10,10,10,9,8,8,8,8,7,7,7,7],"backgroundColor":["#3B82F6","#3B82F6","#3B82F6","#3B82F6","#3B82F6","#3B82F6","#3B82F6","#3B82F6","#3B82F6","#3B82F6","#3B82F6","#3B82F6","#3B82F6","#3B82F6","#3B82F6","#3B82F6","#3B82F6","#3B82F6","#3B82F6","#3B82F6","#3B82F6","#3B82F6","#3B82F6","#3B82F6","#3B82F6","#3B82F6","#3B82F6","#3B82F6","#3B82F6","#3B82F6"],"percentage":[3.43,2.75,2.35,1.86,1.67,1.67,1.57,1.57,1.47,1.47,1.47,1.37,1.37,1.37,1.18,1.18,1.08,1.08,0.98,0.98,0.98,0.88,0.78,0.78,0.78,0.78,0.69,0.69,0.69,0.69],"barThickness":13}],"rorIds":{"2717":"02rx3b187","3677":"05ecg5h20","4380":"00aft1q37","4227":"050850526","2327":"03rc6as71","2667":"00f1zfq44","3948":"02n415q13","5249":"0524sp257","2588":"026w31v75","3313":"01b40r146","4924":"03y5egs41","230":"01ma17032","250":"00em04n91","4605":"0433kqc49","3537":"04a1mvv97","4427":"05kzjxq56","3102":"00bgk9508","4330":"01wjejq96","2585":"02e91jd64","2798":"04q6c7p66","2799":"04gcegc37","4753":"04d836q62","2497":"01xt2dr21","3127":"05xg72x27","3272":"04qtj9h94","3476":"02czkny70","1319":"03mwgfy56","3029":"03f0f6041","3265":"01zy2cs03","5292":"04kdb0j04"}},"options":{"indexAxis":"y","maintainAspectRatio":false,"scales":{"y":{"ticks":{"precision":0,"autoSkip":false,"font":{"family":"Montserrat"},"color":"#000000"},"stacked":false},"x":{"ticks":{"stepSize":null,"precision":0,"font":{"family":"Montserrat"},"color":"#000000"},"stacked":false}},"plugins":{"legend":{"position":"top","labels":{"font":{"family":"Montserrat"},"color":"#000000"}},"title":{"display":true,"text":"With foreign organizations","font":{"size":24,"family":"Montserrat","weight":600},"color":"#000000"}}}}}