Fraunhofer Portugal Research
Are you a researcher?
Create a profile to get free access to personal recommendations for colleagues and new articles.
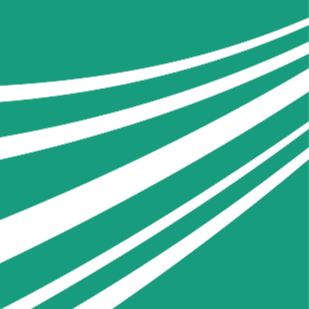
Publications
221
Citations
2 180
h-index
22
Top-3 journals
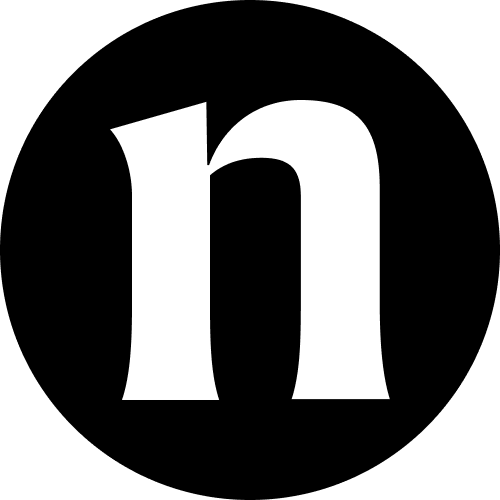
Lecture Notes in Computer Science
(33 publications)
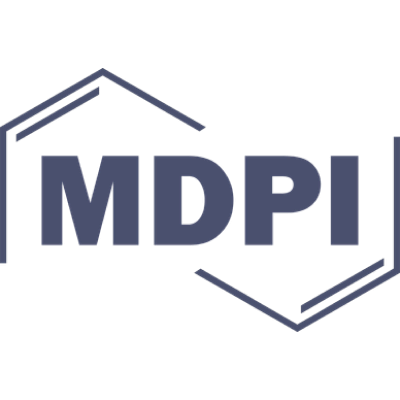
Sensors
(22 publications)
Top-3 organizations
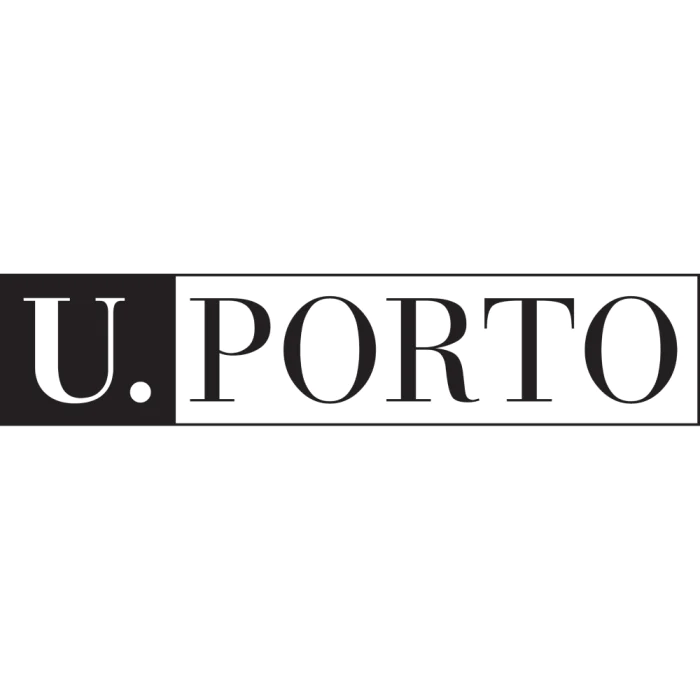
University of Porto
(72 publications)
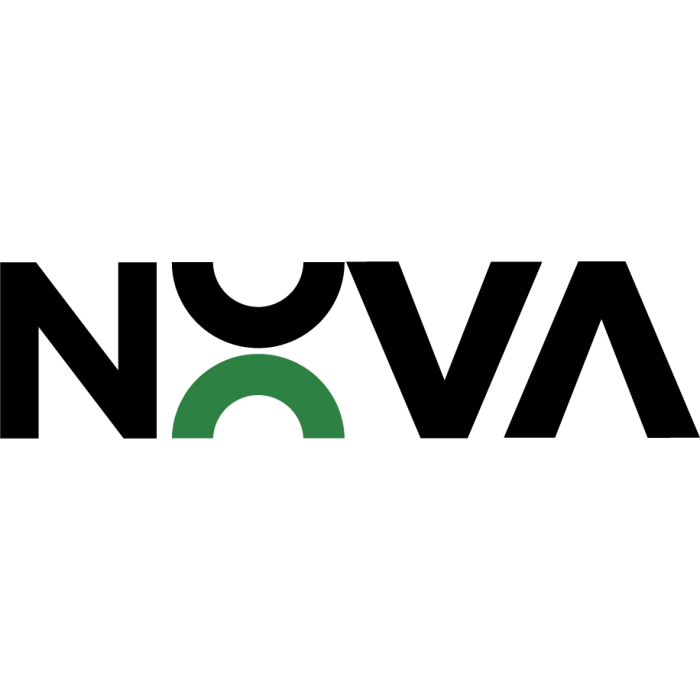
NOVA University Lisbon
(28 publications)
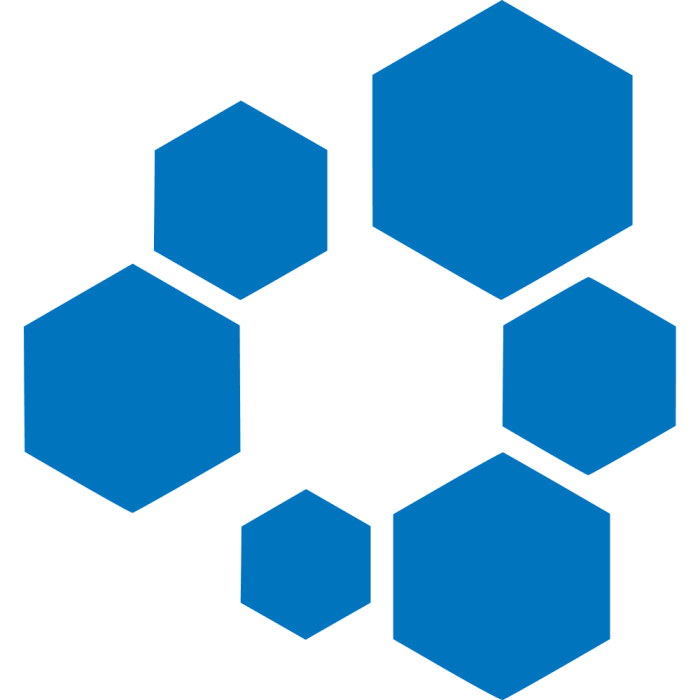
Federal University of Goiás
(20 publications)
Top-3 foreign organizations
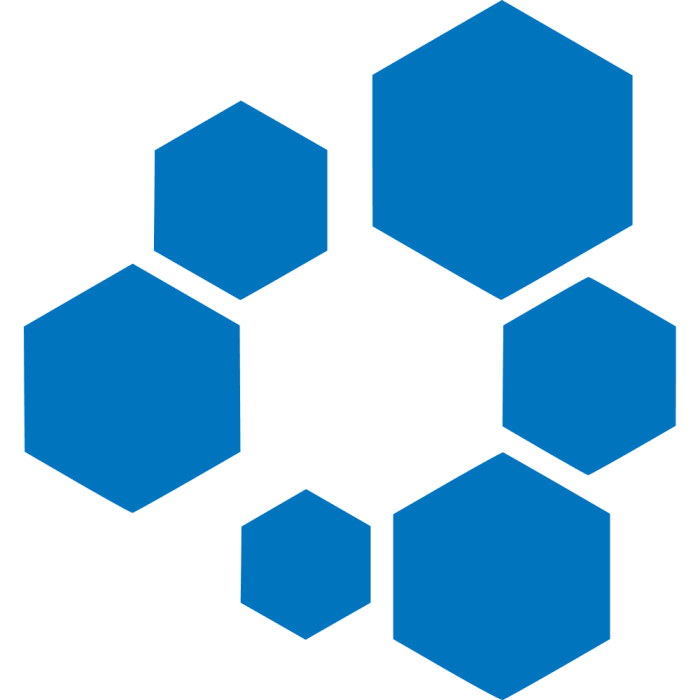
Federal University of Goiás
(20 publications)
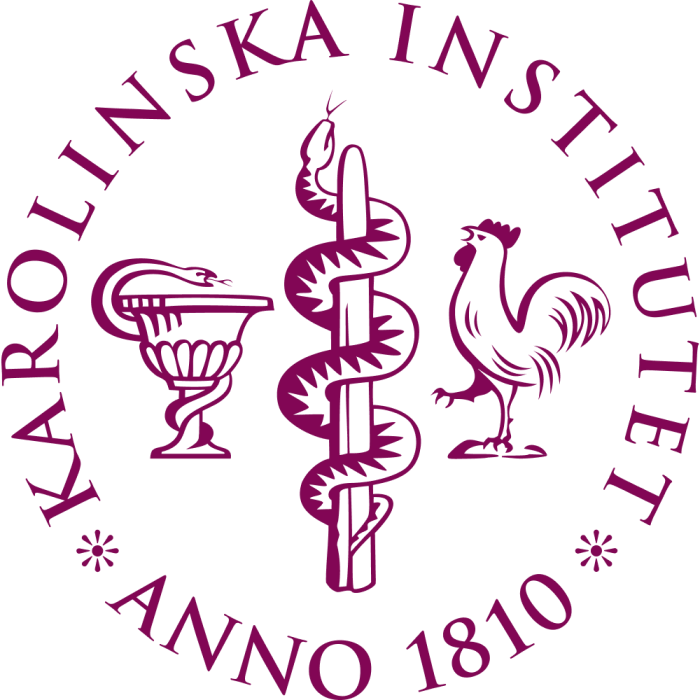
Karolinska Institute
(10 publications)
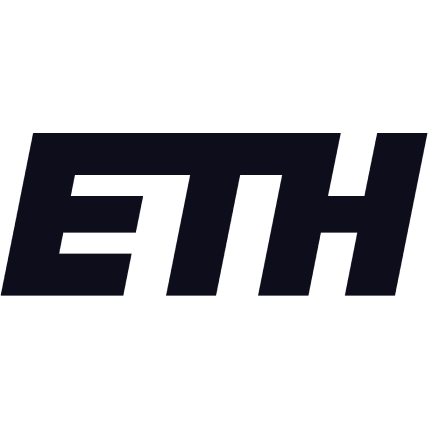
ETH Zurich
(8 publications)
Most cited in 5 years
Nothing found, try to update filter.
Nothing found, try to update filter.












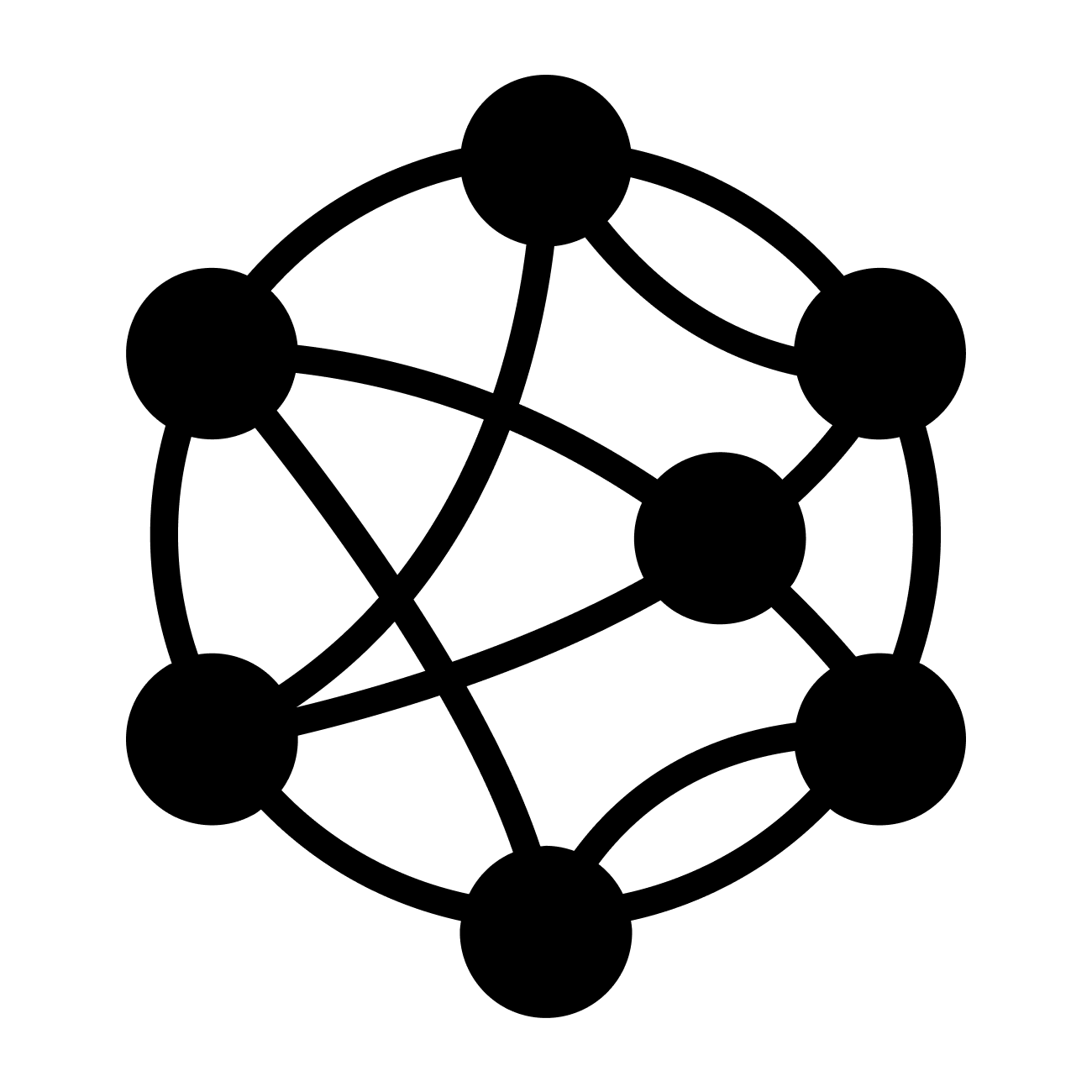