SCImago
Q2
Impact factor
0.3
SJR
0.132
CiteScore
0.5
Categories
History
Literature and Literary Theory
Cultural Studies
Sociology and Political Science
Areas
Arts and Humanities
Social Sciences
Years of issue
1992-2002, 2010-2024
Top-3 citing journals
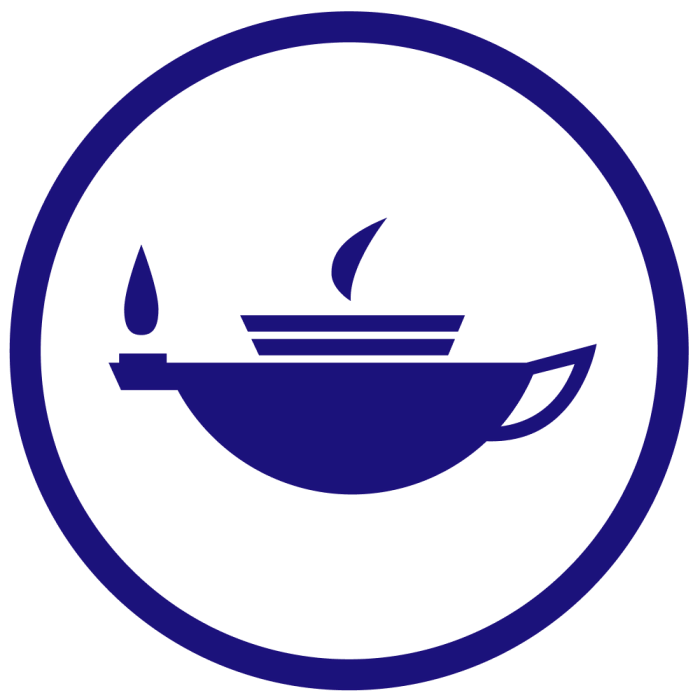
Irish Studies Review
(165 citations)

Irish University Review
(62 citations)
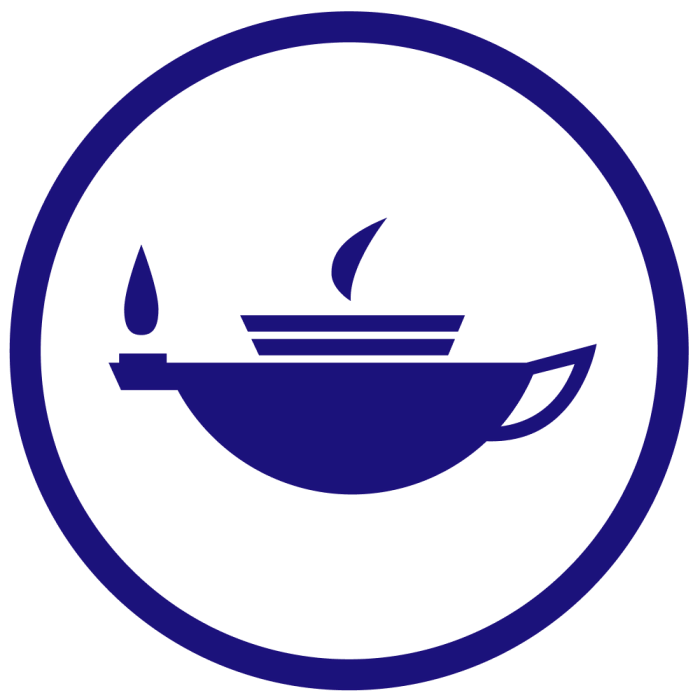
Immigrants and Minorities
(16 citations)
Top-3 organizations
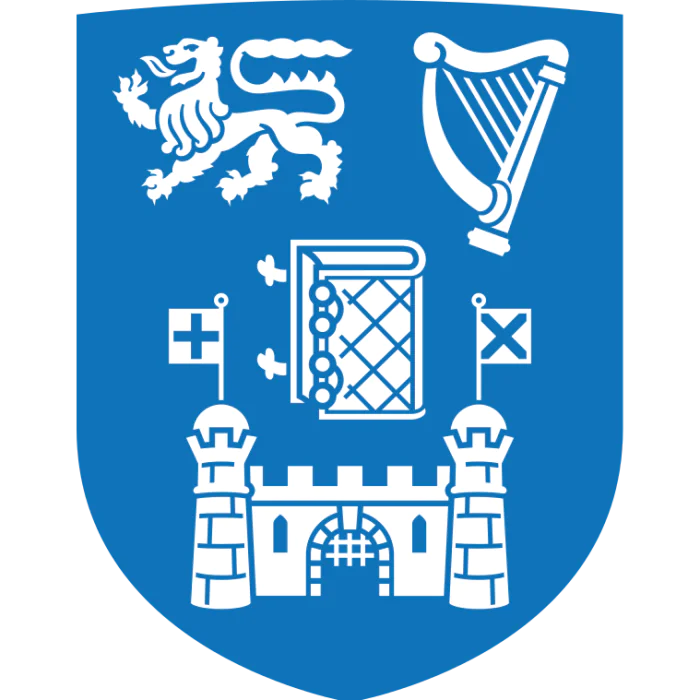
Trinity College Dublin
(66 publications)
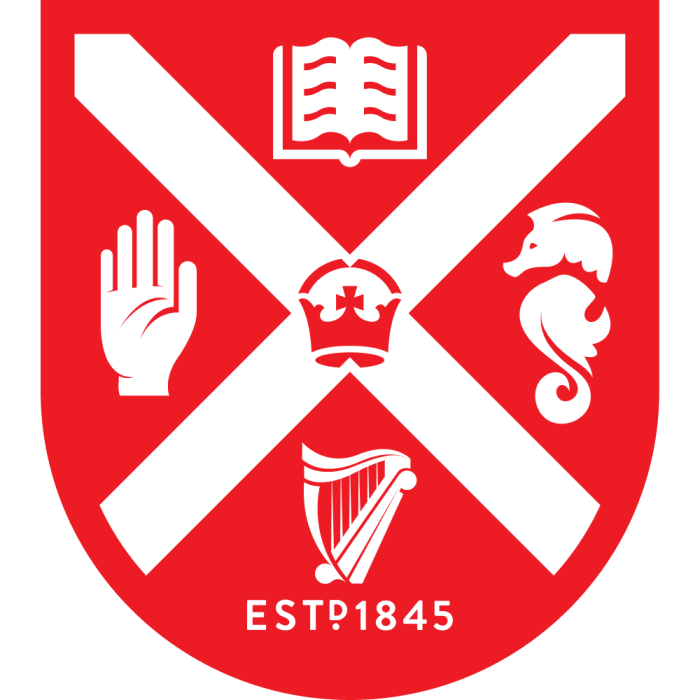
Queen's University Belfast
(37 publications)
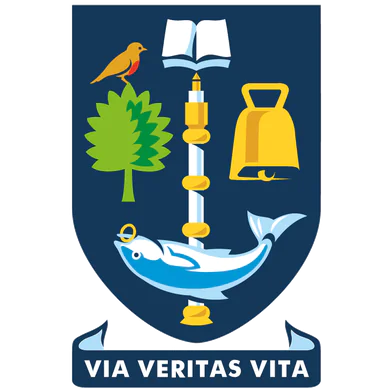
University of Glasgow
(21 publications)
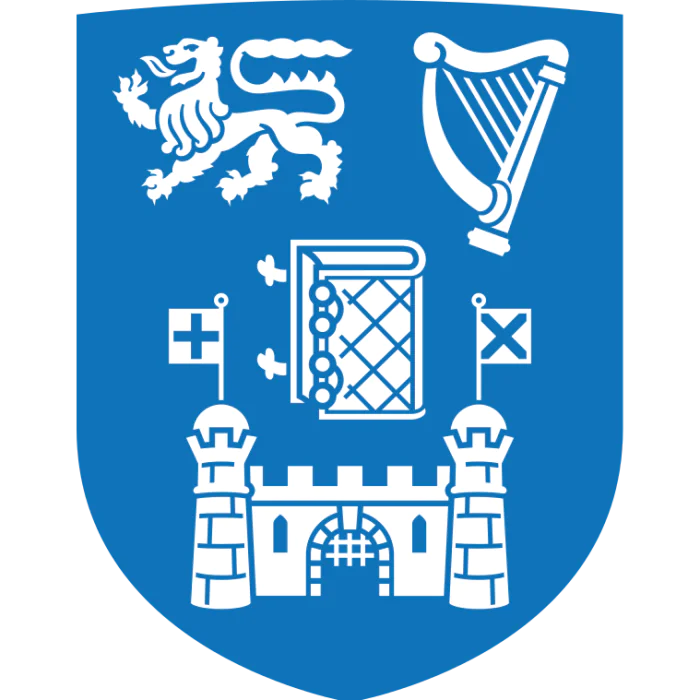
Trinity College Dublin
(22 publications)
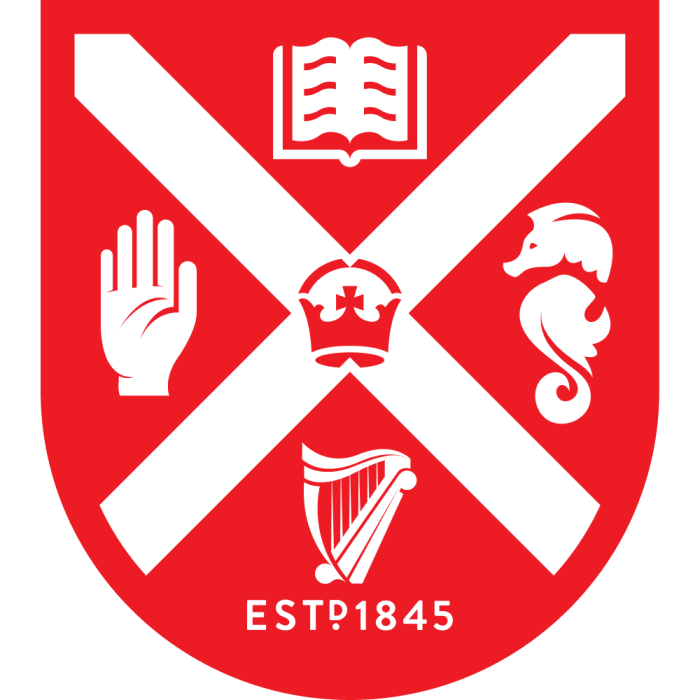
Queen's University Belfast
(8 publications)
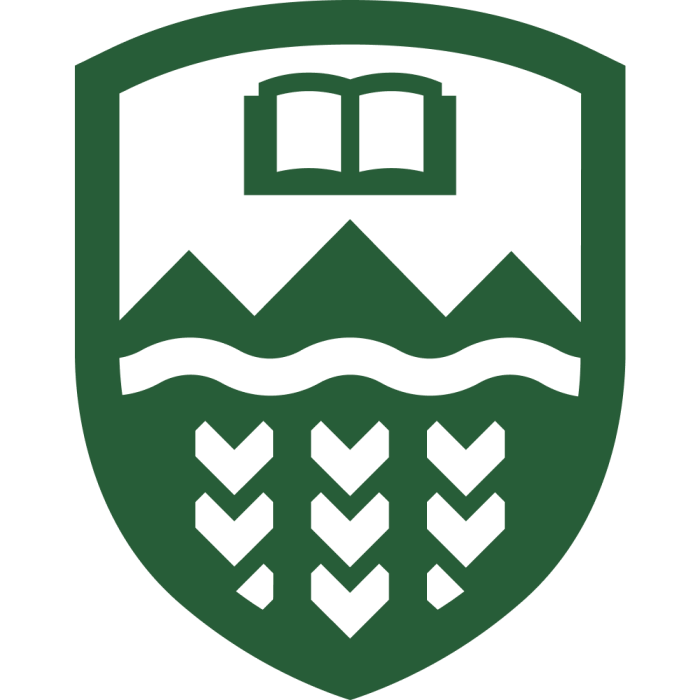
University of Alberta
(8 publications)
Top-3 countries
Most cited in 5 years
Found
Top-100
Citing journals
Citing publishers
Publishing organizations
Publishing organizations in 5 years
Publishing countries
Publishing countries in 5 years
10
20
30
40
50
60
70
80
90
|
|
Ireland
|
Ireland, 90, 22.67%
Ireland
90 publications, 22.67%
|
United Kingdom
|
United Kingdom, 65, 16.37%
United Kingdom
65 publications, 16.37%
|
USA
|
USA, 45, 11.34%
USA
45 publications, 11.34%
|
Canada
|
Canada, 12, 3.02%
Canada
12 publications, 3.02%
|
Spain
|
Spain, 8, 2.02%
Spain
8 publications, 2.02%
|
Australia
|
Australia, 7, 1.76%
Australia
7 publications, 1.76%
|
Belgium
|
Belgium, 5, 1.26%
Belgium
5 publications, 1.26%
|
Thailand
|
Thailand, 4, 1.01%
Thailand
4 publications, 1.01%
|
Germany
|
Germany, 3, 0.76%
Germany
3 publications, 0.76%
|
Hungary
|
Hungary, 3, 0.76%
Hungary
3 publications, 0.76%
|
Czech Republic
|
Czech Republic, 3, 0.76%
Czech Republic
3 publications, 0.76%
|
China
|
China, 2, 0.5%
China
2 publications, 0.5%
|
Netherlands
|
Netherlands, 2, 0.5%
Netherlands
2 publications, 0.5%
|
New Zealand
|
New Zealand, 2, 0.5%
New Zealand
2 publications, 0.5%
|
Norway
|
Norway, 2, 0.5%
Norway
2 publications, 0.5%
|
Finland
|
Finland, 2, 0.5%
Finland
2 publications, 0.5%
|
Japan
|
Japan, 2, 0.5%
Japan
2 publications, 0.5%
|
Argentina
|
Argentina, 1, 0.25%
Argentina
1 publication, 0.25%
|
Brazil
|
Brazil, 1, 0.25%
Brazil
1 publication, 0.25%
|
Greece
|
Greece, 1, 0.25%
Greece
1 publication, 0.25%
|
Egypt
|
Egypt, 1, 0.25%
Egypt
1 publication, 0.25%
|
Malta
|
Malta, 1, 0.25%
Malta
1 publication, 0.25%
|
Poland
|
Poland, 1, 0.25%
Poland
1 publication, 0.25%
|
Singapore
|
Singapore, 1, 0.25%
Singapore
1 publication, 0.25%
|
Turkey
|
Turkey, 1, 0.25%
Turkey
1 publication, 0.25%
|
Chile
|
Chile, 1, 0.25%
Chile
1 publication, 0.25%
|
Sweden
|
Sweden, 1, 0.25%
Sweden
1 publication, 0.25%
|
10
20
30
40
50
60
70
80
90
|