Are you a researcher?
Create a profile to get free access to personal recommendations for colleagues and new articles.
journal names
Fuelling the World Economy
Top-3 citing journals
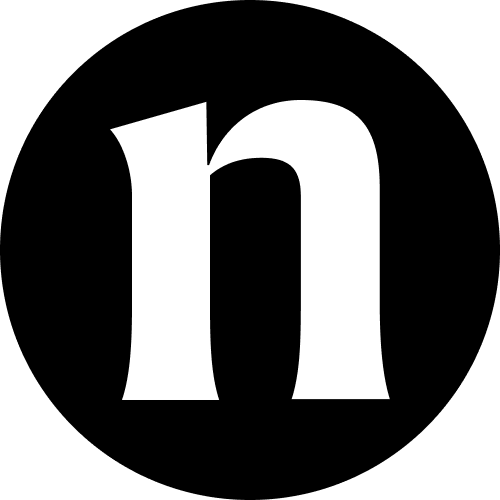
Journal of Shipping and Trade
(3 citations)
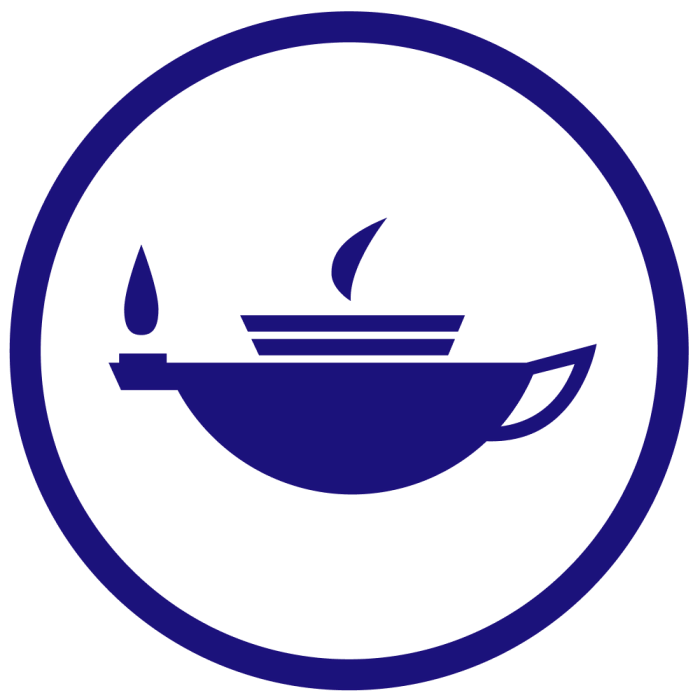
Maritime Policy and Management
(3 citations)
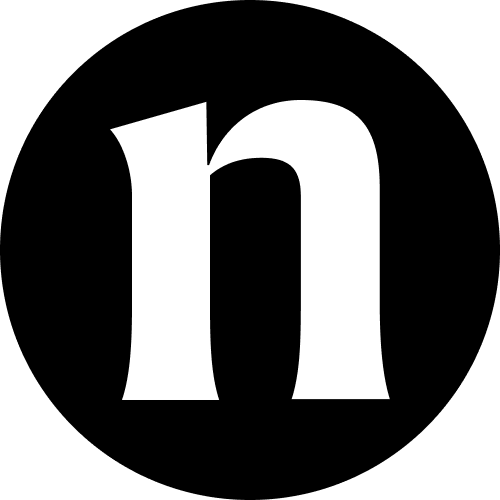
Maritime Economics and Logistics
(2 citations)
Top-3 organizations
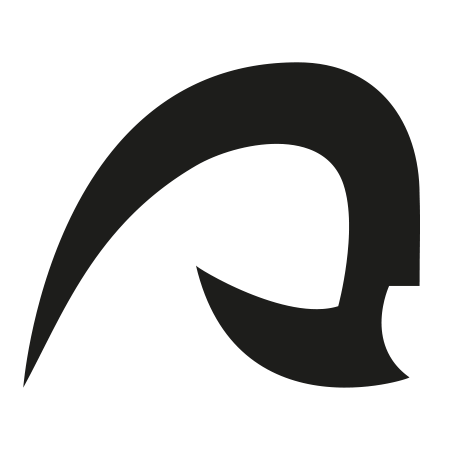
University of Las Palmas de Gran Canaria
(9 publications)
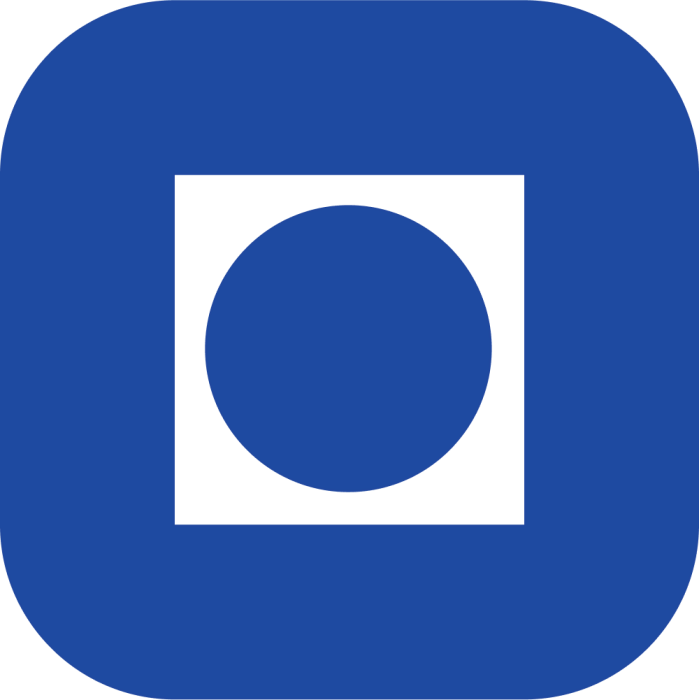
Norwegian University of Science and Technology
(7 publications)
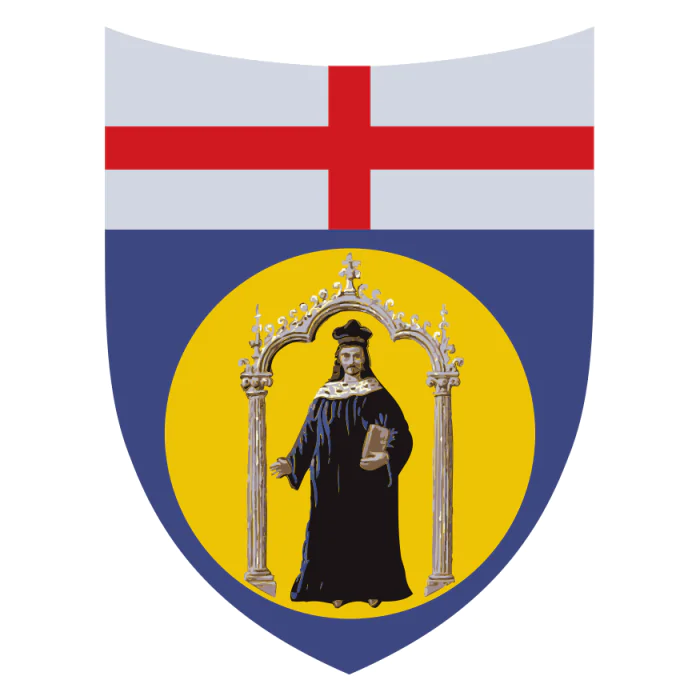
University of Genoa
(7 publications)
Top-3 countries
Most cited in 5 years
Found
Publications found: 70
A survey on deep learning for polyp segmentation: techniques, challenges and future trends
Mei J., Zhou T., Huang K., Zhang Y., Zhou Y., Wu Y., Fu H.
AbstractEarly detection and assessment of polyps play a crucial role in the prevention and treatment of colorectal cancer (CRC). Polyp segmentation provides an effective solution to assist clinicians in accurately locating and segmenting polyp regions. In the past, people often relied on manually extracted lower-level features such as color, texture, and shape, which often had problems capturing global context and lacked robustness to complex scenarios. With the advent of deep learning, more and more medical image segmentation algorithms based on deep learning networks have emerged, making significant progress in the field. This paper provides a comprehensive review of polyp segmentation algorithms. We first review some traditional algorithms based on manually extracted features and deep segmentation algorithms, and then describe benchmark datasets related to the topic. Specifically, we carry out a comprehensive evaluation of recent deep learning models and results based on polyp size, taking into account the focus of research topics and differences in network structures. Finally, we discuss the challenges of polyp segmentation and future trends in the field.
FusionMamba: dynamic feature enhancement for multimodal image fusion with Mamba
Xie X., Cui Y., Tan T., Zheng X., Yu Z.
AbstractMultimodal image fusion aims to integrate information from different imaging techniques to produce a comprehensive, detail-rich single image for downstream vision tasks. Existing methods based on local convolutional neural networks (CNNs) struggle to capture global features efficiently, while Transformer-based models are computationally expensive, although they excel at global modeling. Mamba addresses these limitations by leveraging selective structured state space models (S4) to effectively handle long-range dependencies while maintaining linear complexity. In this paper, we propose FusionMamba, a novel dynamic feature enhancement framework that aims to overcome the challenges faced by CNNs and Vision Transformers (ViTs) in computer vision tasks. The framework improves the visual state-space model Mamba by integrating dynamic convolution and channel attention mechanisms, which not only retains its powerful global feature modeling capability, but also greatly reduces redundancy and enhances the expressiveness of local features. In addition, we have developed a new module called the dynamic feature fusion module (DFFM). It combines the dynamic feature enhancement module (DFEM) for texture enhancement and disparity perception with the cross-modal fusion Mamba module (CMFM), which focuses on enhancing the inter-modal correlation while suppressing redundant information. Experiments show that FusionMamba achieves state-of-the-art performance in a variety of multimodal image fusion tasks as well as downstream experiments, demonstrating its broad applicability and superiority.
Unified regularity measures for sample-wise learning and generalization
Zhang C., Yuan M., Ma X., Liu Y., Lu H., Wang L., Su Y., Liu Y.
AbstractFundamental machine learning theory shows that different samples contribute unequally to both the learning and testing processes. Recent studies on deep neural networks (DNNs) suggest that such sample differences are rooted in the distribution of intrinsic pattern information, namely sample regularity. Motivated by recent discoveries in network memorization and generalization, we propose a pair of sample regularity measures with a formulation-consistent representation for both processes. Specifically, the cumulative binary training/generalizing loss (CBTL/CBGL), the cumulative number of correct classifications of the training/test sample within the training phase, is proposed to quantify the stability in the memorization-generalization process, while forgetting/mal-generalizing events (ForEvents/MgEvents), i.e., the misclassification of previously learned or generalized samples, are utilized to represent the uncertainty of sample regularity with respect to optimization dynamics. The effectiveness and robustness of the proposed approaches for mini-batch stochastic gradient descent (SGD) optimization are validated through sample-wise analyses. Further training/test sample selection applications show that the proposed measures, which share the unified computing procedure, could benefit both tasks.
An empirical study of LLaMA3 quantization: from LLMs to MLLMs
Huang W., Zheng X., Ma X., Qin H., Lv C., Chen H., Luo J., Qi X., Liu X., Magno M.
AbstractThe LLaMA family, a collection of foundation language models ranging from 7B to 65B parameters, has become one of the most powerful open-source large language models (LLMs) and the popular LLM backbone of multi-modal large language models (MLLMs), widely used in computer vision and natural language understanding tasks. In particular, LLaMA3 models have recently been released and have achieved impressive performance in various domains with super-large scale pre-training on over 15T tokens of data. Given the wide application of low-bit quantization for LLMs in resource-constrained scenarios, we explore LLaMA3’s capabilities when quantized to low bit-width. This exploration can potentially provide new insights and challenges for the low-bit quantization of LLaMA3 and other future LLMs, especially in addressing performance degradation issues that suffer in LLM compression. Specifically, we comprehensively evaluate the 10 existing post-training quantization and LoRA fine-tuning (LoRA-FT) methods of LLaMA3 on 1-8 bits and various datasets to reveal the low-bit quantization performance of LLaMA3. To uncover the capabilities of low-bit quantized MLLM, we assessed the performance of the LLaMA3-based LLaVA-Next-8B model under 2-4 ultra-low bits with post-training quantization methods. Our experimental results indicate that LLaMA3 still suffers from non-negligible degradation in linguistic and visual contexts, particularly under ultra-low bit widths. This highlights the significant performance gap at low bit-width that needs to be addressed in future developments. We expect that this empirical study will prove valuable in advancing future models, driving LLMs and MLLMs to achieve higher accuracy at lower bit to enhance practicality.
Spatial-temporal initialization dilemma: towards realistic visual tracking
Liu C., Yuan Y., Chen X., Lu H., Wang D.
AbstractIn this paper, we first investigate the phenomenon of the spatial-temporal initialization dilemma towards realistic visual tracking, which may adversely affect tracking performance. We summarize the aforementioned phenomenon by comparing differences of the initialization manners in existing tracking benchmarks and in real-world applications. The existing tracking benchmarks provide offline sequences and the expert annotations in the initial frame for trackers. However, in real-world applications, a tracker is often initialized by user annotations or an object detector, which may provide rough and inaccurate initialization. Moreover, annotation from the external feedback also introduces extra time costs while the video stream will not pause for waiting. We select four representative trackers and conduct full performance comparison on popular datasets with simulated initialization to intuitively describe the initialization dilemma of the task. Then, we propose a simple compensation framework to address this dilemma. The framework contains spatial-refine and temporal-chasing modules to mitigate performance degradation caused by the initialization dilemma. Furthermore, the proposed framework can be compatible with various popular trackers without retraining. Extensive experiments verify the effectiveness of our compensation framework.
An overview of large AI models and their applications
Tu X., He Z., Huang Y., Zhang Z., Yang M., Zhao J.
AbstractIn recent years, large-scale artificial intelligence (AI) models have become a focal point in technology, attracting widespread attention and acclaim. Notable examples include Google’s BERT and OpenAI’s GPT, which have scaled their parameter sizes to hundreds of billions or even tens of trillions. This growth has been accompanied by a significant increase in the amount of training data, significantly improving the capabilities and performance of these models. Unlike previous reviews, this paper provides a comprehensive discussion of the algorithmic principles of large-scale AI models and their industrial applications from multiple perspectives. We first outline the evolutionary history of these models, highlighting milestone algorithms while exploring their underlying principles and core technologies. We then evaluate the challenges and limitations of large-scale AI models, including computational resource requirements, model parameter inflation, data privacy concerns, and specific issues related to multi-modal AI models, such as reliance on text-image pairs, inconsistencies in understanding and generation capabilities, and the lack of true “multi-modality”. Various industrial applications of these models are also presented. Finally, we discuss future trends, predicting further expansion of model scale and the development of cross-modal fusion. This study provides valuable insights to inform and inspire future future research and practice.
Patch is enough: naturalistic adversarial patch against vision-language pre-training models
Kong D., Liang S., Zhu X., Zhong Y., Ren W.
AbstractVisual language pre-training (VLP) models have demonstrated significant success in various domains, but they remain vulnerable to adversarial attacks. Addressing these adversarial vulnerabilities is crucial for enhancing security in multi-modal learning. Traditionally, adversarial methods that target VLP models involve simultaneous perturbation of images and text. However, this approach faces significant challenges. First, adversarial perturbations often fail to translate effectively into real-world scenarios. Second, direct modifications to the text are conspicuously visible. To overcome these limitations, we propose a novel strategy that uses only image patches for attacks, thus preserving the integrity of the original text. Our method leverages prior knowledge from diffusion models to enhance the authenticity and naturalness of the perturbations. Moreover, to optimize patch placement and improve the effectiveness of our attacks, we utilize the cross-attention mechanism, which encapsulates inter-modal interactions by generating attention maps to guide strategic patch placement. Extensive experiments conducted in a white-box setting for image-to-text scenarios reveal that our proposed method significantly outperforms existing techniques, achieving a 100% attack success rate.
Mini-InternVL: a flexible-transfer pocket multi-modal model with 5% parameters and 90% performance
Gao Z., Chen Z., Cui E., Ren Y., Wang W., Zhu J., Tian H., Ye S., He J., Zhu X., Lu L., Lu T., Qiao Y., Dai J., Wang W.
AbstractMulti-modal large language models (MLLMs) have demonstrated impressive performance in vision-language tasks across a wide range of domains. However, the large model scale and associated high computational cost pose significant challenges for training and deploying MLLMs on consumer-grade GPUs or edge devices, thereby hindering their widespread application. In this work, we introduce Mini-InternVL, a series of MLLMs with parameters ranging from 1 billion to 4 billion, which achieves 90% of the performance with only 5% of the parameters. This significant improvement in efficiency and effectiveness makes our models more accessible and applicable in various real-world scenarios. To further promote the adoption of our models, we are developing a unified adaptation framework for Mini-InternVL, which enables our models to transfer and outperform specialized models in downstream tasks, including autonomous driving, medical image processing, and remote sensing. We believe that our models can provide valuable insights and resources to advance the development of efficient and effective MLLMs.
ViTGaze: gaze following with interaction features in vision transformers
Song Y., Wang X., Yao J., Liu W., Zhang J., Xu X.
AbstractGaze following aims to interpret human-scene interactions by predicting the person’s focal point of gaze. Prevailing approaches often adopt a two-stage framework, whereby multi-modality information is extracted in the initial stage for gaze target prediction. Consequently, the efficacy of these methods highly depends on the precision of the previous modality extraction. Others use a single-modality approach with complex decoders, increasing network computational load. Inspired by the remarkable success of pre-trained plain vision transformers (ViTs), we introduce a novel single-modality gaze following framework called ViTGaze. In contrast to previous methods, it creates a novel gaze following framework based mainly on powerful encoders (relative decoder parameters less than 1%). Our principal insight is that the inter-token interactions within self-attention can be transferred to interactions between humans and scenes. Leveraging this presumption, we formulate a framework consisting of a 4D interaction encoder and a 2D spatial guidance module to extract human-scene interaction information from self-attention maps. Furthermore, our investigation reveals that ViT with self-supervised pre-training has an enhanced ability to extract correlation information. Many experiments have been conducted to demonstrate the performance of the proposed method. Our method achieves state-of-the-art performance among all single-modality methods (3.4% improvement in the area under curve score, 5.1% improvement in the average precision) and very comparable performance against multi-modality methods with 59% fewer parameters.
A divide-and-conquer reconstruction method for defending against adversarial example attacks
Liu X., Hu J., Yang Q., Jiang M., He J., Fang H.
AbstractIn recent years, defending against adversarial examples has gained significant importance, leading to a growing body of research in this area. Among these studies, pre-processing defense approaches have emerged as a prominent research direction. However, existing adversarial example pre-processing techniques often employ a single pre-processing model to counter different types of adversarial attacks. Such a strategy may miss the nuances between different types of attacks, limiting the comprehensiveness and effectiveness of the defense strategy. To address this issue, we propose a divide-and-conquer reconstruction pre-processing algorithm via multi-classification and multi-network training to more effectively defend against different types of mainstream adversarial attacks. The premise and challenge of the divide-and-conquer reconstruction defense is to distinguish between multiple types of adversarial attacks. Our method designs an adversarial attack classification module that exploits the high-frequency information differences between different types of adversarial examples for their multi-classification, which can hardly be achieved by existing adversarial example detection methods. In addition, we construct a divide-and-conquer reconstruction module that utilizes different trained image reconstruction models for each type of adversarial attack, ensuring optimal defense effectiveness. Extensive experiments show that our proposed divide-and-conquer defense algorithm exhibits superior performance compared to state-of-the-art pre-processing methods.
Counterfactual discriminative micro-expression recognition
Li Y., Liu M., Lao L., Wang Y., Cui Z.
AbstractMicro-expressions are spontaneous, rapid and subtle facial movements that can hardly be suppressed or fabricated. Micro-expression recognition (MER) is one of the most challenging topics in affective computing. It aims to recognize subtle facial movements which are quite difficult for humans to perceive in a fleeting period. Recently, many deep learning-based MER methods have been developed. However, how to effectively capture subtle temporal variations for robust MER still perplexes us. We propose a counterfactual discriminative micro-expression recognition (CoDER) method to effectively learn the slight temporal variations for video-based MER. To explicitly capture the causality from temporal dynamics hidden in the micro-expression (ME) sequence, we propose ME counterfactual reasoning by comparing the effects of the facts w.r.t. original ME sequences and the counterfactuals w.r.t. counterfactually-revised ME sequences, and then perform causality-aware prediction to encourage the model to learn those latent ME temporal cues. Extensive experiments on four widely-used ME databases demonstrate the effectiveness of CoDER, which results in comparable and superior MER performance compared with that of the state-of-the-art methods. The visualization results show that CoDER successfully perceives the meaningful temporal variations in sequential faces.
Learning a generalizable re-identification model from unlabelled data with domain-agnostic expert
Liu F., Ye M., Du B.
AbstractIn response to real-world scenarios, the domain generalization (DG) problem has spurred considerable research in person re-identification (ReID). This challenge arises when the target domain, which is significantly different from the source domains, remains unknown. However, the performance of current DG ReID relies heavily on labor-intensive source domain annotations. Considering the potential of unlabeled data, we investigate unsupervised domain generalization (UDG) in ReID. Our goal is to create a model that can generalize from unlabeled source domains to semantically retrieve images in an unseen target domain. To address this, we propose a new approach that trains a domain-agnostic expert (DaE) for unsupervised domain-generalizable person ReID. This involves independently training multiple experts to account for label space inconsistencies between source domains. At the same time, the DaE captures domain-generalizable information for testing. Our experiments demonstrate the effectiveness of this method for learning generalizable features under the UDG setting. The results demonstrate the superiority of our method over state-of-the-art techniques. We will make our code and models available for public use.
Review on synergizing the Metaverse and AI-driven synthetic data: enhancing virtual realms and activity recognition in computer vision
Rajendran M., Tan C.T., Atmosukarto I., Ng A.B., See S.
AbstractThe Metaverse’s emergence is redefining digital interaction, enabling seamless engagement in immersive virtual realms. This trend’s integration with AI and virtual reality (VR) is gaining momentum, albeit with challenges in acquiring extensive human action datasets. Real-world activities involve complex intricate behaviors, making accurate capture and annotation difficult. VR compounds this difficulty by requiring meticulous simulation of natural movements and interactions. As the Metaverse bridges the physical and digital realms, the demand for diverse human action data escalates, requiring innovative solutions to enrich AI and VR capabilities. This need is underscored by state-of-the-art models that excel but are hampered by limited real-world data. The overshadowing of synthetic data benefits further complicates the issue. This paper systematically examines both real-world and synthetic datasets for activity detection and recognition in computer vision. Introducing Metaverse-enabled advancements, we unveil SynDa’s novel streamlined pipeline using photorealistic rendering and AI pose estimation. By fusing real-life video datasets, large-scale synthetic datasets are generated to augment training and mitigate real data scarcity and costs. Our preliminary experiments reveal promising results in terms of mean average precision (mAP), where combining real data and synthetic video data generated using this pipeline to train models presents an improvement in mAP (32.35%), compared to the mAP of the same model when trained on real data (29.95%). This demonstrates the transformative synergy between Metaverse and AI-driven synthetic data augmentation.
Face shape transfer via semantic warping
Li Z., Lv X., Yu W., Liu Q., Lin J., Zhang S.
AbstractFace reshaping aims to adjust the shape of a face in a portrait image to make the face aesthetically beautiful, which has many potential applications. Existing methods 1) operate on the pre-defined facial landmarks, leading to artifacts and distortions due to the limited number of landmarks, 2) synthesize new faces based on segmentation masks or sketches, causing generated faces to look dissatisfied due to the losses of skin details and difficulties in dealing with hair and background blurring, and 3) project the positions of the deformed feature points from the 3D face model to the 2D image, making the results unrealistic because of the misalignment between feature points. In this paper, we propose a novel method named face shape transfer (FST) via semantic warping, which can transfer both the overall face and individual components (e.g., eyes, nose, and mouth) of a reference image to the source image. To achieve controllability at the component level, we introduce five encoding networks, which are designed to learn feature embedding specific to different face components. To effectively exploit the features obtained from semantic parsing maps at different scales, we employ a straightforward method of directly connecting all layers within the global dense network. This direct connection facilitates maximum information flow between layers, efficiently utilizing diverse scale semantic parsing information. To avoid deformation artifacts, we introduce a spatial transformer network, allowing the network to handle different types of semantic warping effectively. To facilitate extensive evaluation, we construct a large-scale high-resolution face dataset, which contains 14,000 images with a resolution of 1024 × 1024. Superior performance of our method is demonstrated by qualitative and quantitative experiments on the benchmark dataset.
A fast mask synthesis method for face recognition
Guo K., Zhao C., Wang J.
AbstractMask face recognition has recently gained increasing attention in the current context. Face mask occlusion seriously affects the performance of face recognition systems, because more than 75% of the face area remains unexposed and the mask directly causes an increase in intra-class differences and a decrease in inter-class separability in the feature space. To improve the performance of face recognition model against mask occlusion, we propose a fast and efficient method for mask generation in this paper, which can avoid the need for large-scale collection of real-world mask face training sets. This approach can be embedded in the training process of any mask face model as a module and is very flexible. Experiments on the MLFW, MFR2 and RMFD datasets show the effectiveness and flexibility of our approach that outperform the state-of-the-art methods.