Fraunhofer Institute for Integrated Circuits
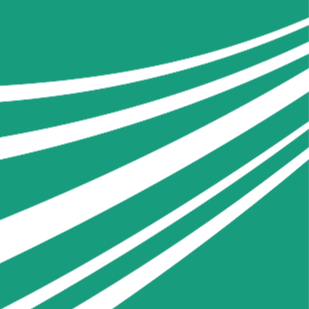
Publications
1 065
Citations
12 304
h-index
47
Top-3 journals
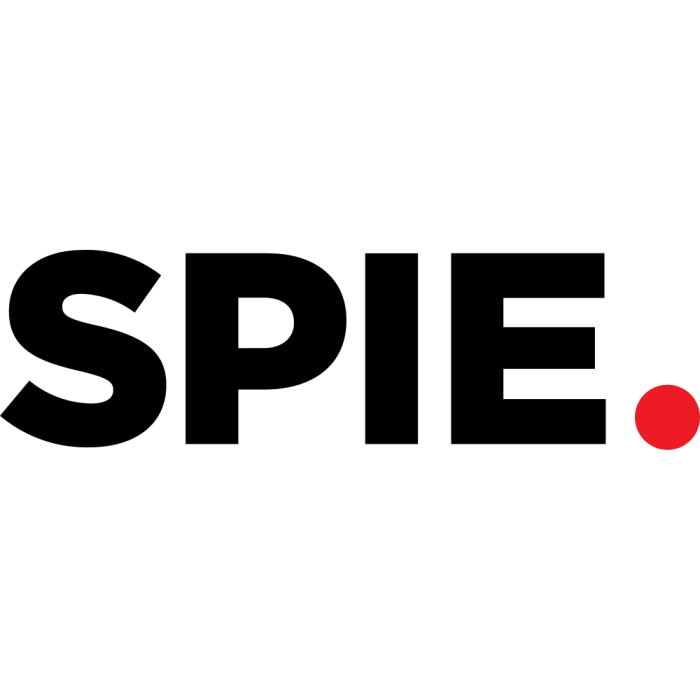
Proceedings of SPIE - The International Society for Optical Engineering
(67 publications)
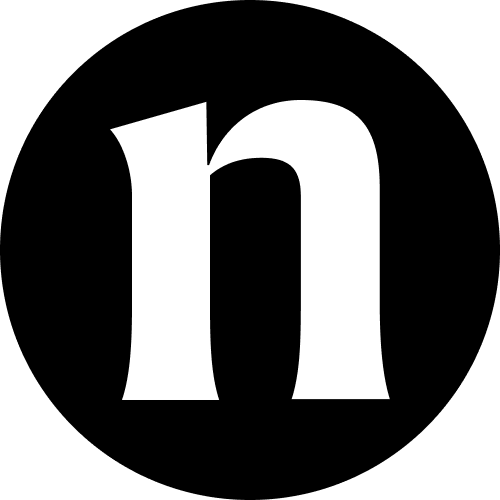
Lecture Notes in Computer Science
(57 publications)
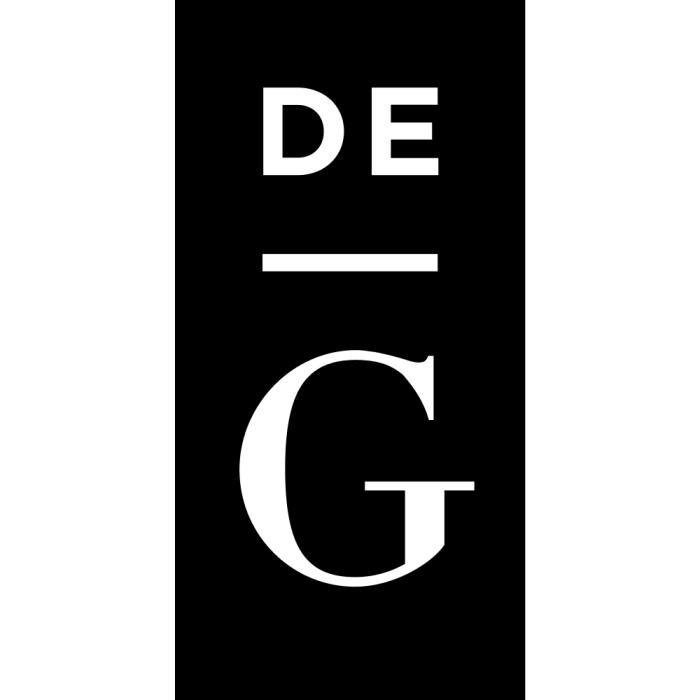
Current Directions in Biomedical Engineering
(37 publications)
Top-3 organizations
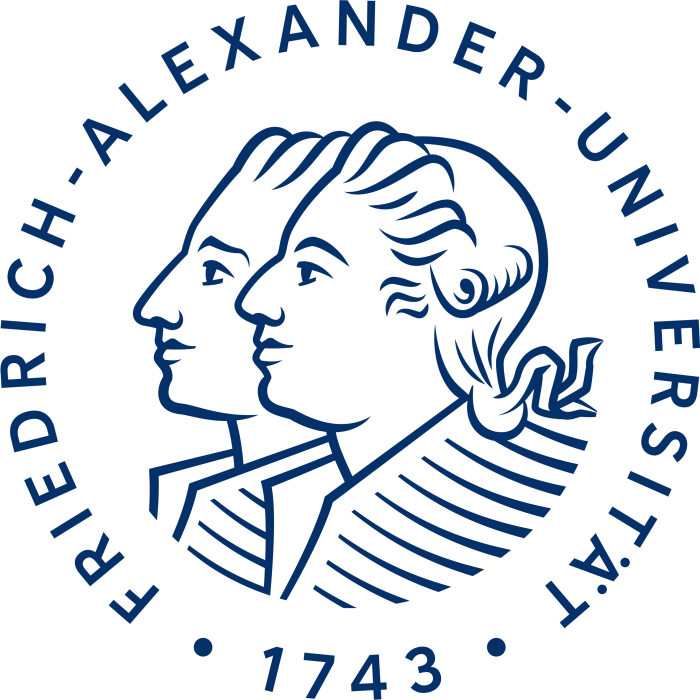
University of Erlangen–Nuremberg
(347 publications)
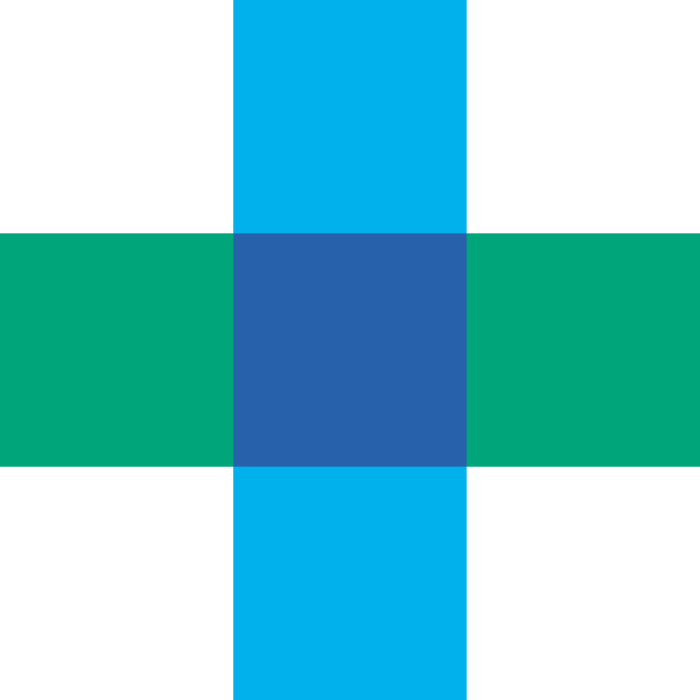
University Hospital Erlangen
(69 publications)
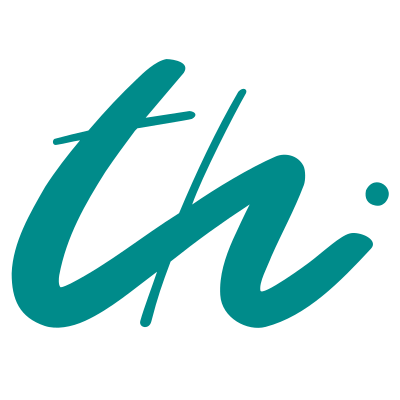
Ilmenau University of Technology
(47 publications)
Top-3 foreign organizations
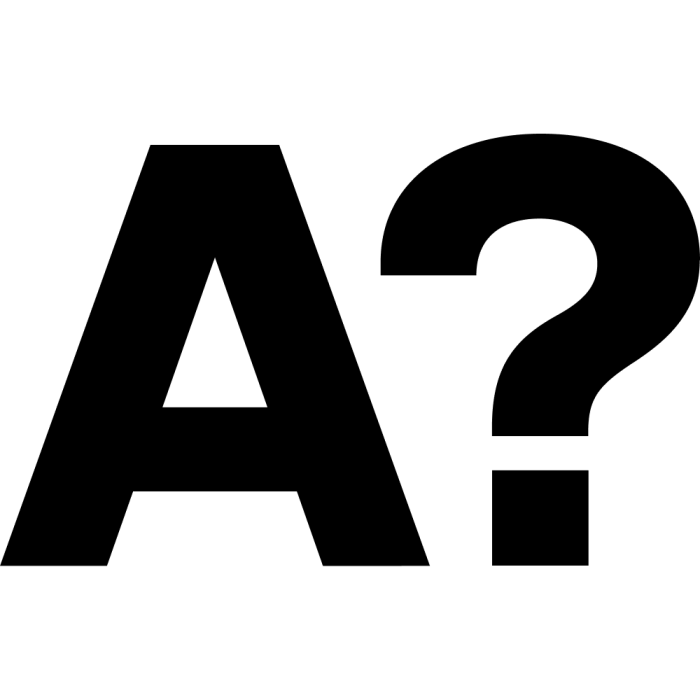
Aalto University
(10 publications)
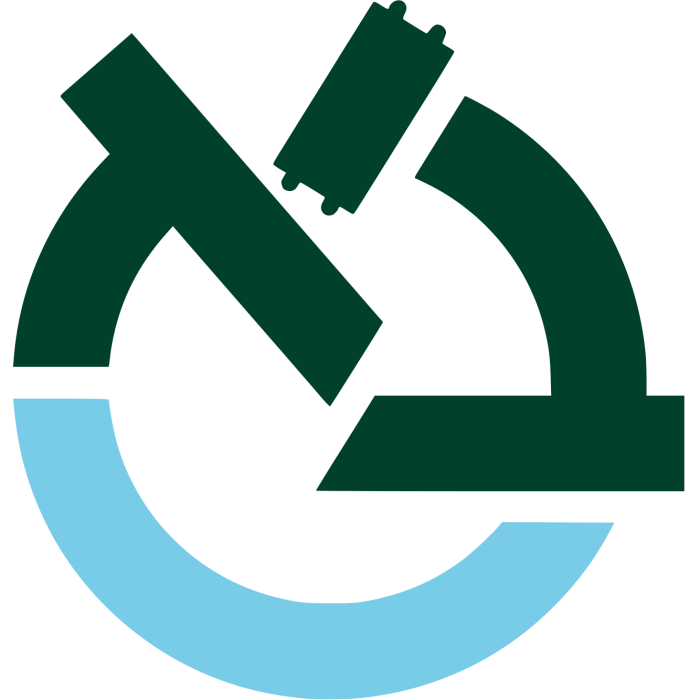
Bar-Ilan University
(10 publications)
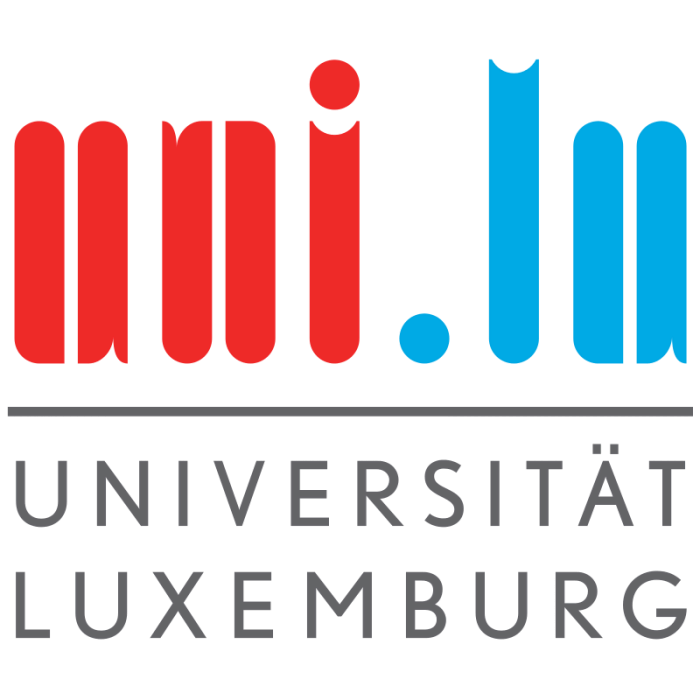
University of Luxembourg
(9 publications)
Since 1991
Total publications
1065
Total citations
12294
Citations per publication
11.54
Average publications per year
31.32
Average authors per publication
6.38
h-index
47
Metrics description
h-index
A scientist has an h-index if h of his N publications are cited at least h times each, while the remaining (N - h) publications are cited no more than h times each.
Top-30
Fields of science
50
100
150
200
250
300
|
|
Electrical and Electronic Engineering
|
Electrical and Electronic Engineering, 278, 26.1%
Electrical and Electronic Engineering
278 publications, 26.1%
|
Condensed Matter Physics
|
Condensed Matter Physics, 96, 9.01%
Condensed Matter Physics
96 publications, 9.01%
|
Instrumentation
|
Instrumentation, 91, 8.54%
Instrumentation
91 publications, 8.54%
|
Electronic, Optical and Magnetic Materials
|
Electronic, Optical and Magnetic Materials, 67, 6.29%
Electronic, Optical and Magnetic Materials
67 publications, 6.29%
|
General Materials Science
|
General Materials Science, 66, 6.2%
General Materials Science
66 publications, 6.2%
|
Signal Processing
|
Signal Processing, 61, 5.73%
Signal Processing
61 publications, 5.73%
|
Applied Mathematics
|
Applied Mathematics, 57, 5.35%
Applied Mathematics
57 publications, 5.35%
|
Acoustics and Ultrasonics
|
Acoustics and Ultrasonics, 57, 5.35%
Acoustics and Ultrasonics
57 publications, 5.35%
|
Computer Science Applications
|
Computer Science Applications, 55, 5.16%
Computer Science Applications
55 publications, 5.16%
|
Atomic and Molecular Physics, and Optics
|
Atomic and Molecular Physics, and Optics, 55, 5.16%
Atomic and Molecular Physics, and Optics
55 publications, 5.16%
|
Biomedical Engineering
|
Biomedical Engineering, 51, 4.79%
Biomedical Engineering
51 publications, 4.79%
|
General Engineering
|
General Engineering, 49, 4.6%
General Engineering
49 publications, 4.6%
|
Surfaces, Coatings and Films
|
Surfaces, Coatings and Films, 45, 4.23%
Surfaces, Coatings and Films
45 publications, 4.23%
|
Software
|
Software, 39, 3.66%
Software
39 publications, 3.66%
|
Materials Chemistry
|
Materials Chemistry, 37, 3.47%
Materials Chemistry
37 publications, 3.47%
|
Computational Mathematics
|
Computational Mathematics, 37, 3.47%
Computational Mathematics
37 publications, 3.47%
|
General Medicine
|
General Medicine, 36, 3.38%
General Medicine
36 publications, 3.38%
|
Nuclear and High Energy Physics
|
Nuclear and High Energy Physics, 36, 3.38%
Nuclear and High Energy Physics
36 publications, 3.38%
|
Computer Science (miscellaneous)
|
Computer Science (miscellaneous), 34, 3.19%
Computer Science (miscellaneous)
34 publications, 3.19%
|
Mechanical Engineering
|
Mechanical Engineering, 34, 3.19%
Mechanical Engineering
34 publications, 3.19%
|
Hardware and Architecture
|
Hardware and Architecture, 31, 2.91%
Hardware and Architecture
31 publications, 2.91%
|
Computer Networks and Communications
|
Computer Networks and Communications, 29, 2.72%
Computer Networks and Communications
29 publications, 2.72%
|
Biochemistry
|
Biochemistry, 28, 2.63%
Biochemistry
28 publications, 2.63%
|
Analytical Chemistry
|
Analytical Chemistry, 27, 2.54%
Analytical Chemistry
27 publications, 2.54%
|
Mechanics of Materials
|
Mechanics of Materials, 27, 2.54%
Mechanics of Materials
27 publications, 2.54%
|
Media Technology
|
Media Technology, 27, 2.54%
Media Technology
27 publications, 2.54%
|
Control and Systems Engineering
|
Control and Systems Engineering, 24, 2.25%
Control and Systems Engineering
24 publications, 2.25%
|
General Computer Science
|
General Computer Science, 23, 2.16%
General Computer Science
23 publications, 2.16%
|
General Physics and Astronomy
|
General Physics and Astronomy, 20, 1.88%
General Physics and Astronomy
20 publications, 1.88%
|
Computer Vision and Pattern Recognition
|
Computer Vision and Pattern Recognition, 20, 1.88%
Computer Vision and Pattern Recognition
20 publications, 1.88%
|
50
100
150
200
250
300
|
Journals
10
20
30
40
50
60
70
|
|
Proceedings of SPIE - The International Society for Optical Engineering
67 publications, 6.29%
|
|
Lecture Notes in Computer Science
57 publications, 5.35%
|
|
Current Directions in Biomedical Engineering
37 publications, 3.47%
|
|
IEEE/ACM Transactions on Audio Speech and Language Processing
33 publications, 3.1%
|
|
Nuclear Instruments and Methods in Physics Research, Section B: Beam Interactions with Materials and Atoms
22 publications, 2.07%
|
|
Sensors
22 publications, 2.07%
|
|
Informatik aktuell
18 publications, 1.69%
|
|
IEEE Signal Processing Letters
16 publications, 1.5%
|
|
Journal of the Acoustical Society of America
16 publications, 1.5%
|
|
Materials Science Forum
13 publications, 1.22%
|
|
Journal of Applied Physics
13 publications, 1.22%
|
|
Microelectronic Engineering
13 publications, 1.22%
|
|
Communications in Computer and Information Science
12 publications, 1.13%
|
|
Nuclear Instruments and Methods in Physics Research, Section A: Accelerators, Spectrometers, Detectors and Associated Equipment
11 publications, 1.03%
|
|
Laryngo- Rhino- Otologie
11 publications, 1.03%
|
|
IEEE Access
11 publications, 1.03%
|
|
IFMBE Proceedings
10 publications, 0.94%
|
|
IEEE Transactions on Broadcasting
10 publications, 0.94%
|
|
Journal of Crystal Growth
10 publications, 0.94%
|
|
International Journal of Satellite Communications and Networking
9 publications, 0.85%
|
|
TM. Technisches Messen
9 publications, 0.85%
|
|
Microelectronics Journal
8 publications, 0.75%
|
|
IEEE Transactions on Audio Speech and Language Processing
8 publications, 0.75%
|
|
Microelectronics Reliability
7 publications, 0.66%
|
|
Applied Sciences (Switzerland)
7 publications, 0.66%
|
|
Thin Solid Films
7 publications, 0.66%
|
|
IEEE Transactions on Antennas and Propagation
6 publications, 0.56%
|
|
Solid-State Electronics
6 publications, 0.56%
|
|
Biomedizinische Technik
6 publications, 0.56%
|
|
International Journal of Antennas and Propagation
6 publications, 0.56%
|
|
10
20
30
40
50
60
70
|
Publishers
20
40
60
80
100
120
140
160
180
200
|
|
Springer Nature
199 publications, 18.69%
|
|
Elsevier
193 publications, 18.12%
|
|
Institute of Electrical and Electronics Engineers (IEEE)
183 publications, 17.18%
|
|
SPIE-Intl Soc Optical Eng
72 publications, 6.76%
|
|
Wiley
61 publications, 5.73%
|
|
Walter de Gruyter
59 publications, 5.54%
|
|
MDPI
54 publications, 5.07%
|
|
Georg Thieme Verlag KG
21 publications, 1.97%
|
|
AIP Publishing
20 publications, 1.88%
|
|
Acoustical Society of America (ASA)
17 publications, 1.6%
|
|
Taylor & Francis
15 publications, 1.41%
|
|
Trans Tech Publications
14 publications, 1.31%
|
|
Hindawi Limited
11 publications, 1.03%
|
|
Frontiers Media S.A.
10 publications, 0.94%
|
|
ifmbe proceedings
10 publications, 0.94%
|
|
Association for Computing Machinery (ACM)
8 publications, 0.75%
|
|
IOP Publishing
8 publications, 0.75%
|
|
American Vacuum Society
7 publications, 0.66%
|
|
Cambridge University Press
6 publications, 0.56%
|
|
Institution of Engineering and Technology (IET)
6 publications, 0.56%
|
|
Materials Research Society
6 publications, 0.56%
|
|
Oxford University Press
5 publications, 0.47%
|
|
IOS Press
5 publications, 0.47%
|
|
American Chemical Society (ACS)
5 publications, 0.47%
|
|
SAE International
5 publications, 0.47%
|
|
Emerald
4 publications, 0.38%
|
|
Ovid Technologies (Wolters Kluwer Health)
4 publications, 0.38%
|
|
Institute for Operations Research and the Management Sciences (INFORMS)
4 publications, 0.38%
|
|
World Scientific
3 publications, 0.28%
|
|
Public Library of Science (PLoS)
3 publications, 0.28%
|
|
20
40
60
80
100
120
140
160
180
200
|
With other organizations
50
100
150
200
250
300
350
|
|
University of Erlangen–Nuremberg
347 publications, 32.58%
|
|
University Hospital Erlangen
69 publications, 6.48%
|
|
Ilmenau University of Technology
47 publications, 4.41%
|
|
Julius Maximilian University of Würzburg
31 publications, 2.91%
|
|
Ludwig Maximilian University of Munich
30 publications, 2.82%
|
|
University of Bamberg
18 publications, 1.69%
|
|
Technical University of Munich
16 publications, 1.5%
|
|
Friedrich Schiller University Jena
13 publications, 1.22%
|
|
Fraunhofer Institute for Nondestructive Testing
13 publications, 1.22%
|
|
Fraunhofer Center for Applied Research on Supply Chain Services
12 publications, 1.13%
|
|
University of Regensburg
12 publications, 1.13%
|
|
Technische Universität Dresden
11 publications, 1.03%
|
|
RWTH Aachen University
11 publications, 1.03%
|
|
Bar-Ilan University
10 publications, 0.94%
|
|
Aalto University
10 publications, 0.94%
|
|
University Hospital Würzburg
10 publications, 0.94%
|
|
University of Passau
10 publications, 0.94%
|
|
University of Luxembourg
9 publications, 0.85%
|
|
Ulm University
9 publications, 0.85%
|
|
Autonomous University of Barcelona
8 publications, 0.75%
|
|
Ruhr University Bochum
8 publications, 0.75%
|
|
Albert Ludwig University of Freiburg
8 publications, 0.75%
|
|
Infineon Technologies AG
8 publications, 0.75%
|
|
Ghent University
7 publications, 0.66%
|
|
Paul Scherrer Institute
7 publications, 0.66%
|
|
University College London
7 publications, 0.66%
|
|
Institute for Technical Physics and Materials Science
7 publications, 0.66%
|
|
Interuniversity Microelectronics Centre
7 publications, 0.66%
|
|
Technical University of Dortmund
7 publications, 0.66%
|
|
Fraunhofer Institute for Silicate Research
7 publications, 0.66%
|
|
50
100
150
200
250
300
350
|
With foreign organizations
2
4
6
8
10
|
|
Bar-Ilan University
10 publications, 0.94%
|
|
Aalto University
10 publications, 0.94%
|
|
University of Luxembourg
9 publications, 0.85%
|
|
Autonomous University of Barcelona
8 publications, 0.75%
|
|
Ghent University
7 publications, 0.66%
|
|
Paul Scherrer Institute
7 publications, 0.66%
|
|
University College London
7 publications, 0.66%
|
|
Institute for Technical Physics and Materials Science
7 publications, 0.66%
|
|
Interuniversity Microelectronics Centre
7 publications, 0.66%
|
|
Imperial College London
6 publications, 0.56%
|
|
Johns Hopkins University
6 publications, 0.56%
|
|
University of California, Los Angeles
6 publications, 0.56%
|
|
Institute of Microelectronics of Barcelona
6 publications, 0.56%
|
|
Katholieke Universiteit Leuven
5 publications, 0.47%
|
|
University of Bordeaux
5 publications, 0.47%
|
|
Vienna University of Technology
5 publications, 0.47%
|
|
Osaka University
5 publications, 0.47%
|
|
Luxembourg Institute of Health
5 publications, 0.47%
|
|
European Space Research and Technology Centre
5 publications, 0.47%
|
|
University of Ottawa
5 publications, 0.47%
|
|
University of Leicester
5 publications, 0.47%
|
|
ETH Zurich
4 publications, 0.38%
|
|
Brunel University London
4 publications, 0.38%
|
|
University of Cambridge
4 publications, 0.38%
|
|
Sorbonne University
4 publications, 0.38%
|
|
Joint Institute for Nuclear Research
3 publications, 0.28%
|
|
École Polytechnique Fédérale de Lausanne
3 publications, 0.28%
|
|
KTH Royal Institute of Technology
3 publications, 0.28%
|
|
Tampere University
3 publications, 0.28%
|
|
University of Bologna
3 publications, 0.28%
|
|
2
4
6
8
10
|
With other countries
5
10
15
20
25
30
35
40
45
50
|
|
France
|
France, 49, 4.6%
France
49 publications, 4.6%
|
USA
|
USA, 47, 4.41%
USA
47 publications, 4.41%
|
United Kingdom
|
United Kingdom, 39, 3.66%
United Kingdom
39 publications, 3.66%
|
Spain
|
Spain, 38, 3.57%
Spain
38 publications, 3.57%
|
Austria
|
Austria, 31, 2.91%
Austria
31 publications, 2.91%
|
Italy
|
Italy, 29, 2.72%
Italy
29 publications, 2.72%
|
Netherlands
|
Netherlands, 27, 2.54%
Netherlands
27 publications, 2.54%
|
Switzerland
|
Switzerland, 26, 2.44%
Switzerland
26 publications, 2.44%
|
China
|
China, 21, 1.97%
China
21 publications, 1.97%
|
Hungary
|
Hungary, 19, 1.78%
Hungary
19 publications, 1.78%
|
Belgium
|
Belgium, 18, 1.69%
Belgium
18 publications, 1.69%
|
Brazil
|
Brazil, 17, 1.6%
Brazil
17 publications, 1.6%
|
Canada
|
Canada, 17, 1.6%
Canada
17 publications, 1.6%
|
Finland
|
Finland, 17, 1.6%
Finland
17 publications, 1.6%
|
Israel
|
Israel, 15, 1.41%
Israel
15 publications, 1.41%
|
Sweden
|
Sweden, 15, 1.41%
Sweden
15 publications, 1.41%
|
Japan
|
Japan, 12, 1.13%
Japan
12 publications, 1.13%
|
Russia
|
Russia, 11, 1.03%
Russia
11 publications, 1.03%
|
India
|
India, 11, 1.03%
India
11 publications, 1.03%
|
Czech Republic
|
Czech Republic, 10, 0.94%
Czech Republic
10 publications, 0.94%
|
Luxembourg
|
Luxembourg, 9, 0.85%
Luxembourg
9 publications, 0.85%
|
Norway
|
Norway, 9, 0.85%
Norway
9 publications, 0.85%
|
Portugal
|
Portugal, 7, 0.66%
Portugal
7 publications, 0.66%
|
Greece
|
Greece, 7, 0.66%
Greece
7 publications, 0.66%
|
Australia
|
Australia, 6, 0.56%
Australia
6 publications, 0.56%
|
Denmark
|
Denmark, 5, 0.47%
Denmark
5 publications, 0.47%
|
Egypt
|
Egypt, 5, 0.47%
Egypt
5 publications, 0.47%
|
Iran
|
Iran, 5, 0.47%
Iran
5 publications, 0.47%
|
Ireland
|
Ireland, 5, 0.47%
Ireland
5 publications, 0.47%
|
Poland
|
Poland, 5, 0.47%
Poland
5 publications, 0.47%
|
5
10
15
20
25
30
35
40
45
50
|
- We do not take into account publications without a DOI.
- Statistics recalculated daily.
- Publications published earlier than 1991 are ignored in the statistics.
- The horizontal charts show the 30 top positions.
- Journals quartiles values are relevant at the moment.