Are you a researcher?
Create a profile to get free access to personal recommendations for colleagues and new articles.
SCImago
Q1
Impact factor
1
SJR
0.500
CiteScore
2.2
Categories
Law
Sociology and Political Science
Areas
Social Sciences
Years of issue
1999-2025
journal names
The Journal of Adult Protection
J ADULT PROT
Top-3 citing journals
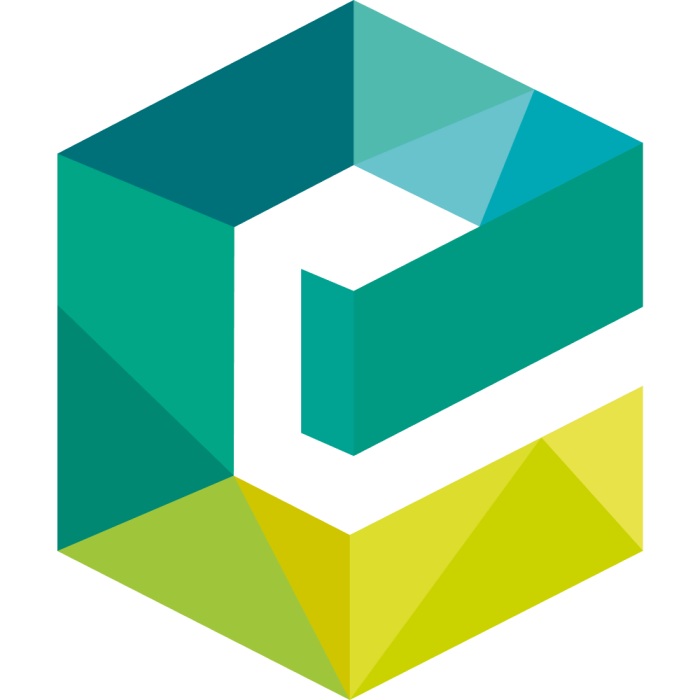
The Journal of Adult Protection
(1007 citations)
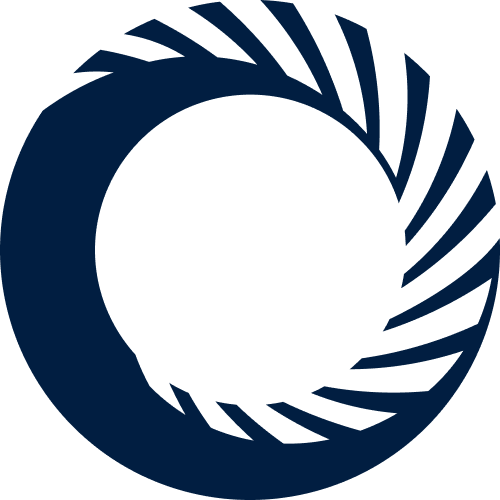
British Journal of Social Work
(131 citations)
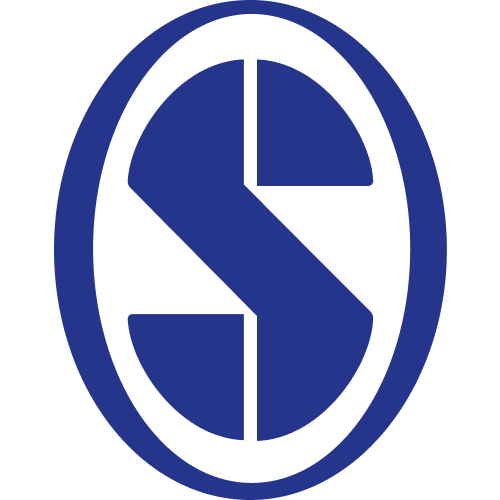
Journal of Social Work
(90 citations)
Top-3 organizations
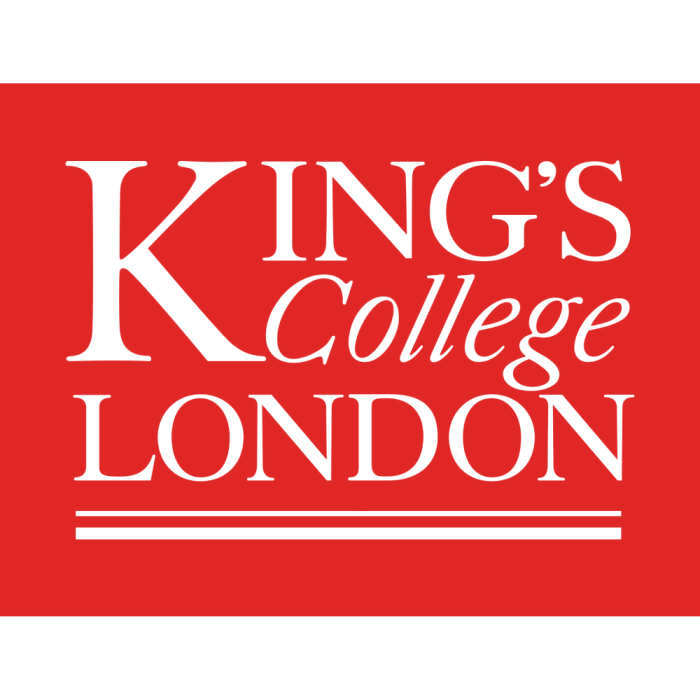
King's College London
(31 publications)
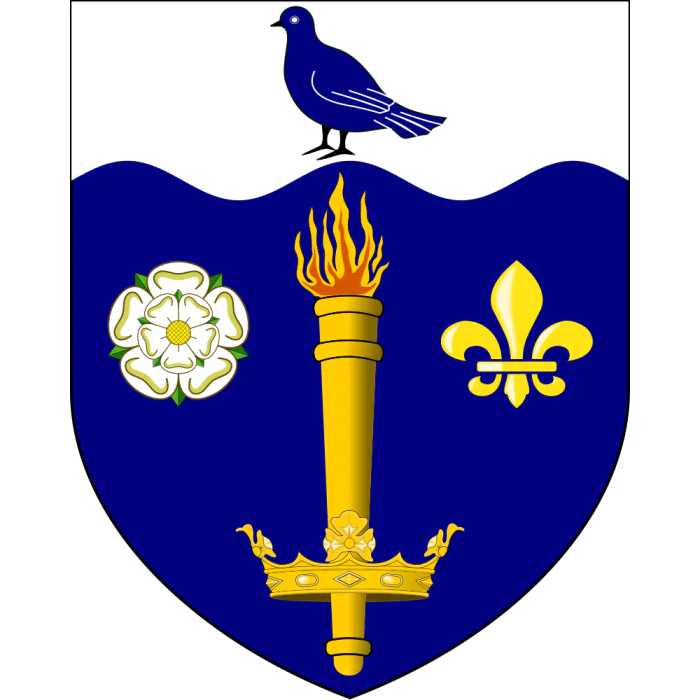
University of Hull
(18 publications)
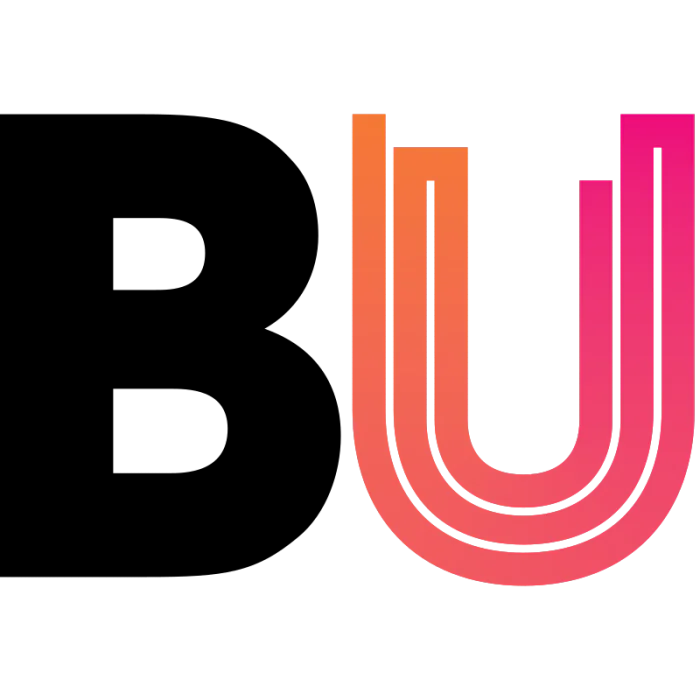
Bournemouth University
(14 publications)
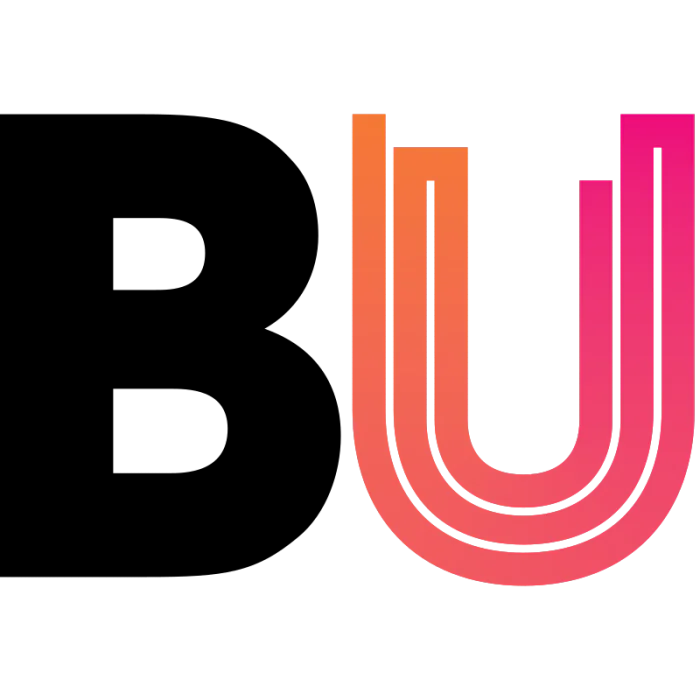
Bournemouth University
(5 publications)
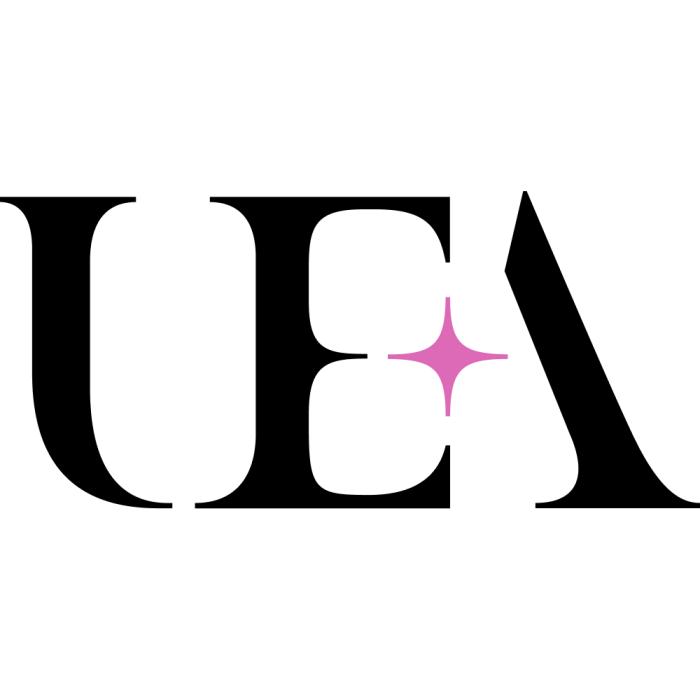
University of East Anglia
(4 publications)
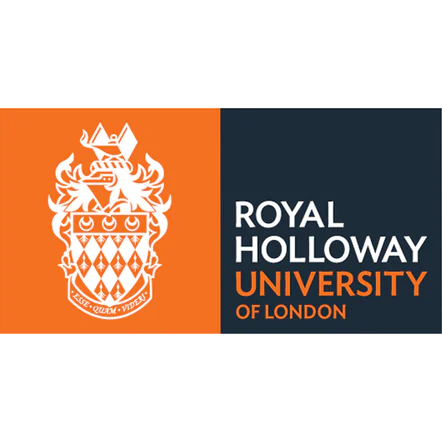
Royal Holloway University of London
(3 publications)
Top-3 countries
Most cited in 5 years
Top-100
Citing journals
200
400
600
800
1000
1200
|
|
The Journal of Adult Protection
1007 citations, 29.9%
|
|
British Journal of Social Work
131 citations, 3.89%
|
|
Journal of Social Work
90 citations, 2.67%
|
|
Journal of Elder Abuse and Neglect
70 citations, 2.08%
|
|
Health and Social Care in the Community
62 citations, 1.84%
|
|
Disability and Society
56 citations, 1.66%
|
|
Journal of Applied Research in Intellectual Disabilities
55 citations, 1.63%
|
|
European Journal of Social Work
35 citations, 1.04%
|
|
Social Work Education
35 citations, 1.04%
|
|
Working with Older People
30 citations, 0.89%
|
|
Ethics and Social Welfare
29 citations, 0.86%
|
|
PLoS ONE
29 citations, 0.86%
|
|
International Journal of Law and Psychiatry
26 citations, 0.77%
|
|
Practice
23 citations, 0.68%
|
|
Trauma, Violence, and Abuse
23 citations, 0.68%
|
|
Journal of Interpersonal Violence
21 citations, 0.62%
|
|
Journal of Intellectual Disabilities
20 citations, 0.59%
|
|
Journal of Advanced Nursing
20 citations, 0.59%
|
|
BMC Health Services Research
19 citations, 0.56%
|
|
Policing (Oxford)
19 citations, 0.56%
|
|
International Journal of Environmental Research and Public Health
16 citations, 0.48%
|
|
Ageing International
16 citations, 0.48%
|
|
British Journal of Learning Disabilities
16 citations, 0.48%
|
|
British Journal of Nursing
16 citations, 0.48%
|
|
Social Policy and Society
16 citations, 0.48%
|
|
Ageing and Society
15 citations, 0.45%
|
|
Journal of Family Violence
14 citations, 0.42%
|
|
Tizard Learning Disability Review
14 citations, 0.42%
|
|
Canadian Journal on Aging
13 citations, 0.39%
|
|
International journal of older people nursing
13 citations, 0.39%
|
|
Social Policy and Administration
13 citations, 0.39%
|
|
Journal of Clinical Nursing
13 citations, 0.39%
|
|
Critical Social Policy
12 citations, 0.36%
|
|
Journal of Gerontological Social Work
12 citations, 0.36%
|
|
Journal of Social Welfare and Family Law
12 citations, 0.36%
|
|
Journal of Policy and Practice in Intellectual Disabilities
11 citations, 0.33%
|
|
British Journal of Community Nursing
11 citations, 0.33%
|
|
The Gerontologist
11 citations, 0.33%
|
|
The Police Journal Theory Practice and Principles
11 citations, 0.33%
|
|
Australian Social Work
10 citations, 0.3%
|
|
BMC Geriatrics
10 citations, 0.3%
|
|
Policing and Society
10 citations, 0.3%
|
|
Journal of Social Work Practice
10 citations, 0.3%
|
|
Nursing Ethics
9 citations, 0.27%
|
|
Issues in Mental Health Nursing
9 citations, 0.27%
|
|
BMJ Open
9 citations, 0.27%
|
|
Frontiers in Public Health
9 citations, 0.27%
|
|
International Psychogeriatrics
9 citations, 0.27%
|
|
BMC Public Health
9 citations, 0.27%
|
|
Dementia
9 citations, 0.27%
|
|
Journal of Psychiatric and Mental Health Nursing
9 citations, 0.27%
|
|
Research and Practice in Intellectual and Developmental Disabilities
9 citations, 0.27%
|
|
Educational Gerontology
8 citations, 0.24%
|
|
International Journal of Mental Health Nursing
8 citations, 0.24%
|
|
Frontiers in Psychiatry
8 citations, 0.24%
|
|
Violence Against Women
8 citations, 0.24%
|
|
Health Expectations
8 citations, 0.24%
|
|
Journal of Intellectual Disability Research
7 citations, 0.21%
|
|
Advances in Mental Health and Intellectual Disabilities
7 citations, 0.21%
|
|
Health, Risk and Society
7 citations, 0.21%
|
|
Research in Developmental Disabilities
7 citations, 0.21%
|
|
Journal of Applied Gerontology
7 citations, 0.21%
|
|
Journal of Integrated Care
7 citations, 0.21%
|
|
Cambridge Prisms Global Mental Health
7 citations, 0.21%
|
|
Sexuality and Disability
6 citations, 0.18%
|
|
Campbell Systematic Reviews
6 citations, 0.18%
|
|
Australasian Journal on Ageing
6 citations, 0.18%
|
|
Child Abuse and Neglect
6 citations, 0.18%
|
|
Journal of Intellectual and Developmental Disability
6 citations, 0.18%
|
|
Child Abuse Review
6 citations, 0.18%
|
|
Children and Youth Services Review
6 citations, 0.18%
|
|
Journal of Intellectual Disabilities and Offending Behaviour
6 citations, 0.18%
|
|
Aggression and Violent Behavior
6 citations, 0.18%
|
|
Disability and Rehabilitation
6 citations, 0.18%
|
|
Sociology of Health and Illness
6 citations, 0.18%
|
|
Journal of Aging and Social Policy
6 citations, 0.18%
|
|
BMC Psychiatry
5 citations, 0.15%
|
|
Frontiers in Psychology
5 citations, 0.15%
|
|
Social Work and Social Sciences Review
5 citations, 0.15%
|
|
Cochrane Database of Systematic Reviews
5 citations, 0.15%
|
|
Revista Espanola de Geriatria y Gerontologia
5 citations, 0.15%
|
|
Journal of Mental Health
5 citations, 0.15%
|
|
Critical Criminology
5 citations, 0.15%
|
|
Nurse Education Today
5 citations, 0.15%
|
|
Geriatric Nursing
5 citations, 0.15%
|
|
International Journal of Developmental Disabilities
5 citations, 0.15%
|
|
International Review of Victimology
5 citations, 0.15%
|
|
Nordic Social Work Research
5 citations, 0.15%
|
|
Public Policy & Aging Report
5 citations, 0.15%
|
|
Journal of Financial Crime
4 citations, 0.12%
|
|
Violence and Victims
4 citations, 0.12%
|
|
Nursing standard (Royal College of Nursing (Great Britain) : 1987)
4 citations, 0.12%
|
|
Brain Injury
4 citations, 0.12%
|
|
International Journal of Social Welfare
4 citations, 0.12%
|
|
Family Journal
4 citations, 0.12%
|
|
Policing
4 citations, 0.12%
|
|
Journal of Mental Health Training, Education and Practice
4 citations, 0.12%
|
|
BMC Medical Education
4 citations, 0.12%
|
|
Journal of Social Policy
4 citations, 0.12%
|
|
Psychiatry, Psychology and Law
4 citations, 0.12%
|
|
Show all (70 more) | |
200
400
600
800
1000
1200
|
Citing publishers
200
400
600
800
1000
1200
|
|
Emerald
1117 citations, 33.17%
|
|
Taylor & Francis
460 citations, 13.66%
|
|
Wiley
326 citations, 9.68%
|
|
SAGE
298 citations, 8.85%
|
|
Springer Nature
185 citations, 5.49%
|
|
Oxford University Press
181 citations, 5.37%
|
|
Elsevier
123 citations, 3.65%
|
|
Cambridge University Press
76 citations, 2.26%
|
|
Mark Allen Group
42 citations, 1.25%
|
|
MDPI
31 citations, 0.92%
|
|
Public Library of Science (PLoS)
31 citations, 0.92%
|
|
Frontiers Media S.A.
26 citations, 0.77%
|
|
BMJ
17 citations, 0.5%
|
|
Ovid Technologies (Wolters Kluwer Health)
14 citations, 0.42%
|
|
RCNi
13 citations, 0.39%
|
|
Bristol University Press
11 citations, 0.33%
|
|
American Association on Intellectual and Developmental Disabilities
6 citations, 0.18%
|
|
Scandinavian University Press / Universitetsforlaget AS
6 citations, 0.18%
|
|
Mary Ann Liebert
5 citations, 0.15%
|
|
Whiting & Birch Ltd.
5 citations, 0.15%
|
|
5 citations, 0.15%
|
|
Royal College of Psychiatrists
5 citations, 0.15%
|
|
Springer Publishing Company
4 citations, 0.12%
|
|
SciELO
4 citations, 0.12%
|
|
National Institute for Health and Care Research (NIHR)
4 citations, 0.12%
|
|
IGI Global
4 citations, 0.12%
|
|
Georg Thieme Verlag KG
3 citations, 0.09%
|
|
Stockholm University Press
3 citations, 0.09%
|
|
IOS Press
2 citations, 0.06%
|
|
2 citations, 0.06%
|
|
2 citations, 0.06%
|
|
Fondation Nationale de Gerontologie
2 citations, 0.06%
|
|
European Society of Traumatic Stress Studies (ESTSS)
2 citations, 0.06%
|
|
Cold Spring Harbor Laboratory
2 citations, 0.06%
|
|
Institute of Electrical and Electronics Engineers (IEEE)
2 citations, 0.06%
|
|
JMIR Publications
2 citations, 0.06%
|
|
Virtus Interpress
2 citations, 0.06%
|
|
OpenEdition
2 citations, 0.06%
|
|
Consortium Erudit
2 citations, 0.06%
|
|
IntechOpen
2 citations, 0.06%
|
|
Walter de Gruyter
1 citation, 0.03%
|
|
1 citation, 0.03%
|
|
EDP Sciences
1 citation, 0.03%
|
|
Ediciones Universidad de Salamanca
1 citation, 0.03%
|
|
Universiti Putra Malaysia
1 citation, 0.03%
|
|
American Speech Language Hearing Association
1 citation, 0.03%
|
|
1 citation, 0.03%
|
|
Linkoping University Electronic Press
1 citation, 0.03%
|
|
IOP Publishing
1 citation, 0.03%
|
|
1 citation, 0.03%
|
|
American Medical Association (AMA)
1 citation, 0.03%
|
|
University of Nebraska Press
1 citation, 0.03%
|
|
Borsa Istanbul Anonim Sirketi
1 citation, 0.03%
|
|
Unisa Press
1 citation, 0.03%
|
|
Open Access Macedonian Journal of Medical Sciences
1 citation, 0.03%
|
|
Royal College of General Practitioners
1 citation, 0.03%
|
|
Kemerovo State University
1 citation, 0.03%
|
|
Wilfrid Laurier University Press
1 citation, 0.03%
|
|
Hindawi Limited
1 citation, 0.03%
|
|
Peoples' Friendship University of Russia
1 citation, 0.03%
|
|
American Society of Civil Engineers (ASCE)
1 citation, 0.03%
|
|
Social Science Electronic Publishing
1 citation, 0.03%
|
|
National Library of Serbia
1 citation, 0.03%
|
|
Vandenhoeck & Ruprecht GmbH & Co, KG
1 citation, 0.03%
|
|
AOSIS
1 citation, 0.03%
|
|
SLACK
1 citation, 0.03%
|
|
The Japanese Association of Rural Medicine
1 citation, 0.03%
|
|
Research Square Platform LLC
1 citation, 0.03%
|
|
Show all (38 more) | |
200
400
600
800
1000
1200
|
Publishing organizations
5
10
15
20
25
30
35
|
|
King's College London
31 publications, 4.43%
|
|
University of Hull
18 publications, 2.57%
|
|
Bournemouth University
14 publications, 2%
|
|
Keele University
12 publications, 1.71%
|
|
University of Nottingham
11 publications, 1.57%
|
|
University of Salford
11 publications, 1.57%
|
|
University of East Anglia
10 publications, 1.43%
|
|
University of Stirling
10 publications, 1.43%
|
|
University of Sheffield
8 publications, 1.14%
|
|
University of Plymouth
7 publications, 1%
|
|
De Montfort University
6 publications, 0.86%
|
|
University of Sussex
6 publications, 0.86%
|
|
Manchester Metropolitan University
5 publications, 0.71%
|
|
University of Birmingham
4 publications, 0.57%
|
|
Sheffield Hallam University
4 publications, 0.57%
|
|
Brunel University London
3 publications, 0.43%
|
|
Royal Holloway University of London
3 publications, 0.43%
|
|
University of Manchester
3 publications, 0.43%
|
|
University of Queensland
3 publications, 0.43%
|
|
University of St Andrews
3 publications, 0.43%
|
|
University of Leeds
3 publications, 0.43%
|
|
University College Dublin
3 publications, 0.43%
|
|
Saratov State University
2 publications, 0.29%
|
|
Liverpool John Moores University
2 publications, 0.29%
|
|
University of Oslo
2 publications, 0.29%
|
|
City, University of London
2 publications, 0.29%
|
|
Nottingham Trent University
2 publications, 0.29%
|
|
Griffith University
2 publications, 0.29%
|
|
State University of Padang
2 publications, 0.29%
|
|
University of Hong Kong
2 publications, 0.29%
|
|
Queen's University Belfast
2 publications, 0.29%
|
|
University of the West of England
2 publications, 0.29%
|
|
Northumbria University
2 publications, 0.29%
|
|
University of Porto
2 publications, 0.29%
|
|
University of York
2 publications, 0.29%
|
|
University of Ulster
2 publications, 0.29%
|
|
Cardiff and Vale University Health Board
2 publications, 0.29%
|
|
University of Bradford
2 publications, 0.29%
|
|
Russian Presidential Academy of National Economy and Public Administration
1 publication, 0.14%
|
|
Birla Institute of Technology and Science, Pilani
1 publication, 0.14%
|
|
University of Lahore
1 publication, 0.14%
|
|
Symbiosis International University
1 publication, 0.14%
|
|
Muğla Sıtkı Koçman University
1 publication, 0.14%
|
|
Arak University of Medical Sciences
1 publication, 0.14%
|
|
Amity University, Noida
1 publication, 0.14%
|
|
Frederick University
1 publication, 0.14%
|
|
Sichuan University
1 publication, 0.14%
|
|
University of Haifa
1 publication, 0.14%
|
|
University of Helsinki
1 publication, 0.14%
|
|
Mid Sweden University
1 publication, 0.14%
|
|
Hashemite University
1 publication, 0.14%
|
|
Sun Yat-sen University
1 publication, 0.14%
|
|
University of Eastern Finland
1 publication, 0.14%
|
|
University College London
1 publication, 0.14%
|
|
University of Dundee
1 publication, 0.14%
|
|
University of Warwick
1 publication, 0.14%
|
|
University of Cambridge
1 publication, 0.14%
|
|
Finnish Institute for Health and Welfare
1 publication, 0.14%
|
|
University of Liverpool
1 publication, 0.14%
|
|
St George's, University of London
1 publication, 0.14%
|
|
Edinburgh Napier University
1 publication, 0.14%
|
|
University of Edinburgh
1 publication, 0.14%
|
|
University of Southern California
1 publication, 0.14%
|
|
University of Nicosia
1 publication, 0.14%
|
|
University of Glasgow
1 publication, 0.14%
|
|
Pennsylvania State University
1 publication, 0.14%
|
|
Lincoln University
1 publication, 0.14%
|
|
University of Johannesburg
1 publication, 0.14%
|
|
George Washington University
1 publication, 0.14%
|
|
Makerere University
1 publication, 0.14%
|
|
Northwestern University
1 publication, 0.14%
|
|
Hong Kong Polytechnic University
1 publication, 0.14%
|
|
Brigham and Women's Hospital
1 publication, 0.14%
|
|
Aberystwyth University
1 publication, 0.14%
|
|
Rush University Medical Center
1 publication, 0.14%
|
|
Northeastern University
1 publication, 0.14%
|
|
Federal University of São Carlos
1 publication, 0.14%
|
|
Trinity College Dublin
1 publication, 0.14%
|
|
Vrije Universiteit Brussel
1 publication, 0.14%
|
|
University of Bristol
1 publication, 0.14%
|
|
Vrije Universiteit Amsterdam
1 publication, 0.14%
|
|
University of Macau
1 publication, 0.14%
|
|
Lancaster University
1 publication, 0.14%
|
|
Université de Sherbrooke
1 publication, 0.14%
|
|
Dartmouth College
1 publication, 0.14%
|
|
Cardiff Metropolitan University
1 publication, 0.14%
|
|
Leeds Beckett University
1 publication, 0.14%
|
|
University of Vienna
1 publication, 0.14%
|
|
University of Maryland, Baltimore
1 publication, 0.14%
|
|
Anglia Ruskin University
1 publication, 0.14%
|
|
University of Toronto
1 publication, 0.14%
|
|
University of Calgary
1 publication, 0.14%
|
|
University of Granada
1 publication, 0.14%
|
|
University of North Carolina at Chapel Hill
1 publication, 0.14%
|
|
Indiana University Indianapolis
1 publication, 0.14%
|
|
Bahir Dar University
1 publication, 0.14%
|
|
National Health Service Lothian
1 publication, 0.14%
|
|
University of Essex
1 publication, 0.14%
|
|
University of Portsmouth
1 publication, 0.14%
|
|
Coventry University
1 publication, 0.14%
|
|
Show all (70 more) | |
5
10
15
20
25
30
35
|
Publishing organizations in 5 years
1
2
3
4
5
|
|
Bournemouth University
5 publications, 3.65%
|
|
University of East Anglia
4 publications, 2.92%
|
|
Royal Holloway University of London
3 publications, 2.19%
|
|
State University of Padang
2 publications, 1.46%
|
|
Keele University
2 publications, 1.46%
|
|
University of Stirling
2 publications, 1.46%
|
|
Russian Presidential Academy of National Economy and Public Administration
1 publication, 0.73%
|
|
Birla Institute of Technology and Science, Pilani
1 publication, 0.73%
|
|
University of Lahore
1 publication, 0.73%
|
|
Arak University of Medical Sciences
1 publication, 0.73%
|
|
Amity University, Noida
1 publication, 0.73%
|
|
Sichuan University
1 publication, 0.73%
|
|
Hashemite University
1 publication, 0.73%
|
|
Brunel University London
1 publication, 0.73%
|
|
University of Liverpool
1 publication, 0.73%
|
|
Edinburgh Napier University
1 publication, 0.73%
|
|
University of Southern California
1 publication, 0.73%
|
|
University of Johannesburg
1 publication, 0.73%
|
|
Makerere University
1 publication, 0.73%
|
|
Hong Kong Polytechnic University
1 publication, 0.73%
|
|
Aberystwyth University
1 publication, 0.73%
|
|
Federal University of São Carlos
1 publication, 0.73%
|
|
Queen's University Belfast
1 publication, 0.73%
|
|
Trinity College Dublin
1 publication, 0.73%
|
|
Université de Sherbrooke
1 publication, 0.73%
|
|
Dartmouth College
1 publication, 0.73%
|
|
University of Maryland, Baltimore
1 publication, 0.73%
|
|
University of Porto
1 publication, 0.73%
|
|
Sheffield Hallam University
1 publication, 0.73%
|
|
University of Toronto
1 publication, 0.73%
|
|
University of Sussex
1 publication, 0.73%
|
|
University of North Carolina at Chapel Hill
1 publication, 0.73%
|
|
University College Dublin
1 publication, 0.73%
|
|
University of Essex
1 publication, 0.73%
|
|
University of Plymouth
1 publication, 0.73%
|
|
University of Hull
1 publication, 0.73%
|
|
University of Salford
1 publication, 0.73%
|
|
Show all (7 more) | |
1
2
3
4
5
|
Publishing countries
50
100
150
200
250
300
|
|
United Kingdom
|
United Kingdom, 256, 36.57%
United Kingdom
256 publications, 36.57%
|
Italy
|
Italy, 31, 4.43%
Italy
31 publications, 4.43%
|
USA
|
USA, 28, 4%
USA
28 publications, 4%
|
Canada
|
Canada, 8, 1.14%
Canada
8 publications, 1.14%
|
Ireland
|
Ireland, 7, 1%
Ireland
7 publications, 1%
|
China
|
China, 5, 0.71%
China
5 publications, 0.71%
|
Portugal
|
Portugal, 5, 0.71%
Portugal
5 publications, 0.71%
|
Australia
|
Australia, 5, 0.71%
Australia
5 publications, 0.71%
|
Germany
|
Germany, 4, 0.57%
Germany
4 publications, 0.57%
|
India
|
India, 4, 0.57%
India
4 publications, 0.57%
|
Russia
|
Russia, 3, 0.43%
Russia
3 publications, 0.43%
|
Austria
|
Austria, 3, 0.43%
Austria
3 publications, 0.43%
|
Norway
|
Norway, 3, 0.43%
Norway
3 publications, 0.43%
|
Indonesia
|
Indonesia, 2, 0.29%
Indonesia
2 publications, 0.29%
|
Spain
|
Spain, 2, 0.29%
Spain
2 publications, 0.29%
|
Finland
|
Finland, 2, 0.29%
Finland
2 publications, 0.29%
|
Switzerland
|
Switzerland, 2, 0.29%
Switzerland
2 publications, 0.29%
|
Bangladesh
|
Bangladesh, 1, 0.14%
Bangladesh
1 publication, 0.14%
|
Belgium
|
Belgium, 1, 0.14%
Belgium
1 publication, 0.14%
|
Bosnia and Herzegovina
|
Bosnia and Herzegovina, 1, 0.14%
Bosnia and Herzegovina
1 publication, 0.14%
|
Brazil
|
Brazil, 1, 0.14%
Brazil
1 publication, 0.14%
|
Greece
|
Greece, 1, 0.14%
Greece
1 publication, 0.14%
|
Israel
|
Israel, 1, 0.14%
Israel
1 publication, 0.14%
|
Jordan
|
Jordan, 1, 0.14%
Jordan
1 publication, 0.14%
|
Iran
|
Iran, 1, 0.14%
Iran
1 publication, 0.14%
|
Cyprus
|
Cyprus, 1, 0.14%
Cyprus
1 publication, 0.14%
|
Lithuania
|
Lithuania, 1, 0.14%
Lithuania
1 publication, 0.14%
|
Netherlands
|
Netherlands, 1, 0.14%
Netherlands
1 publication, 0.14%
|
New Zealand
|
New Zealand, 1, 0.14%
New Zealand
1 publication, 0.14%
|
Pakistan
|
Pakistan, 1, 0.14%
Pakistan
1 publication, 0.14%
|
Palestine
|
Palestine, 1, 0.14%
Palestine
1 publication, 0.14%
|
Turkey
|
Turkey, 1, 0.14%
Turkey
1 publication, 0.14%
|
Uganda
|
Uganda, 1, 0.14%
Uganda
1 publication, 0.14%
|
Sweden
|
Sweden, 1, 0.14%
Sweden
1 publication, 0.14%
|
Ethiopia
|
Ethiopia, 1, 0.14%
Ethiopia
1 publication, 0.14%
|
South Africa
|
South Africa, 1, 0.14%
South Africa
1 publication, 0.14%
|
Show all (6 more) | |
50
100
150
200
250
300
|
Publishing countries in 5 years
5
10
15
20
25
30
35
|
|
United Kingdom
|
United Kingdom, 33, 24.09%
United Kingdom
33 publications, 24.09%
|
USA
|
USA, 2, 1.46%
USA
2 publications, 1.46%
|
China
|
China, 2, 1.46%
China
2 publications, 1.46%
|
Portugal
|
Portugal, 2, 1.46%
Portugal
2 publications, 1.46%
|
India
|
India, 2, 1.46%
India
2 publications, 1.46%
|
Indonesia
|
Indonesia, 2, 1.46%
Indonesia
2 publications, 1.46%
|
Ireland
|
Ireland, 2, 1.46%
Ireland
2 publications, 1.46%
|
Canada
|
Canada, 2, 1.46%
Canada
2 publications, 1.46%
|
Bangladesh
|
Bangladesh, 1, 0.73%
Bangladesh
1 publication, 0.73%
|
Brazil
|
Brazil, 1, 0.73%
Brazil
1 publication, 0.73%
|
Jordan
|
Jordan, 1, 0.73%
Jordan
1 publication, 0.73%
|
Iran
|
Iran, 1, 0.73%
Iran
1 publication, 0.73%
|
Italy
|
Italy, 1, 0.73%
Italy
1 publication, 0.73%
|
Pakistan
|
Pakistan, 1, 0.73%
Pakistan
1 publication, 0.73%
|
Palestine
|
Palestine, 1, 0.73%
Palestine
1 publication, 0.73%
|
Uganda
|
Uganda, 1, 0.73%
Uganda
1 publication, 0.73%
|
South Africa
|
South Africa, 1, 0.73%
South Africa
1 publication, 0.73%
|
5
10
15
20
25
30
35
|
1 profile journal article
Wen-Jung Chang
4 publications,
56 citations
h-index: 2