Are you a researcher?
Create a profile to get free access to personal recommendations for colleagues and new articles.
SCImago
Q1
SJR
0.990
CiteScore
4.4
Categories
Earth and Planetary Sciences (miscellaneous)
Geochemistry and Petrology
Geology
Geophysics
Areas
Earth and Planetary Sciences
Years of issue
2018-2023
journal names
Volcanica
Top-3 citing journals
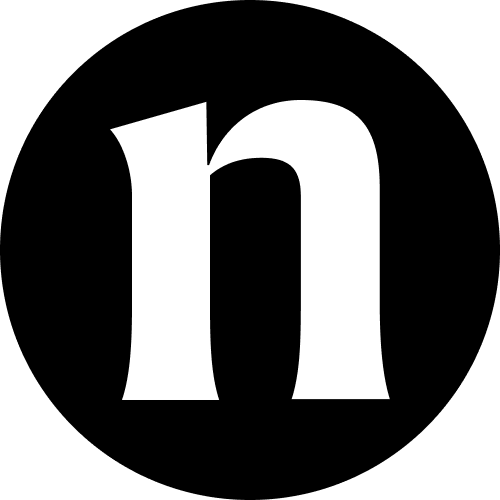
Bulletin of Volcanology
(92 citations)
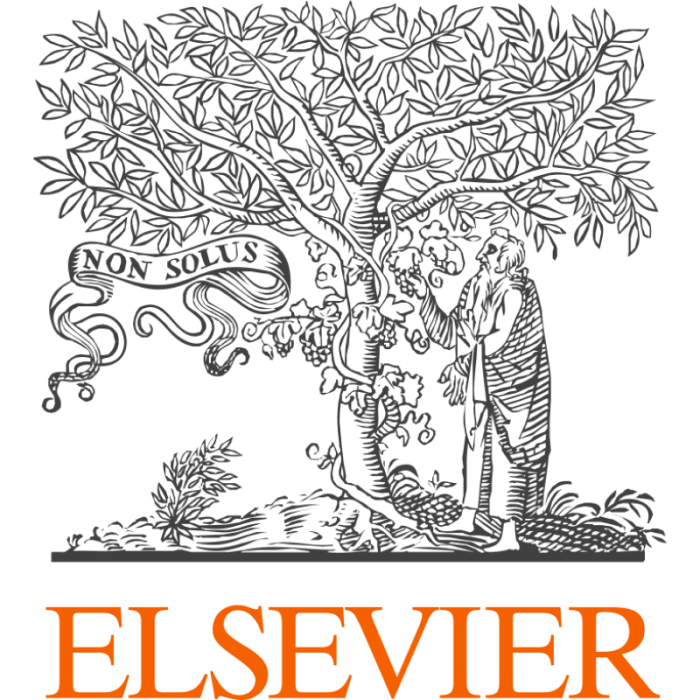
Journal of Volcanology and Geothermal Research
(79 citations)
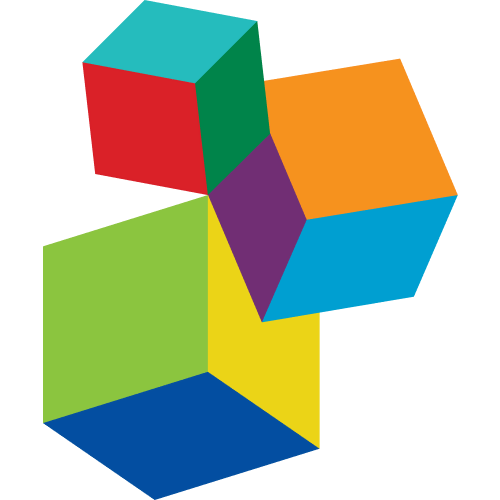
Frontiers in Earth Science
(51 citations)
Most cited in 5 years
Found
Publications found: 217
Erratum. Context Matters: The Importance of Physical Activity Domains for Public Health
Journal for the Measurement of Phys
Q2
Journal for the Measurement of Physical Behaviour
,
2024
,
citations by CoLab: 0
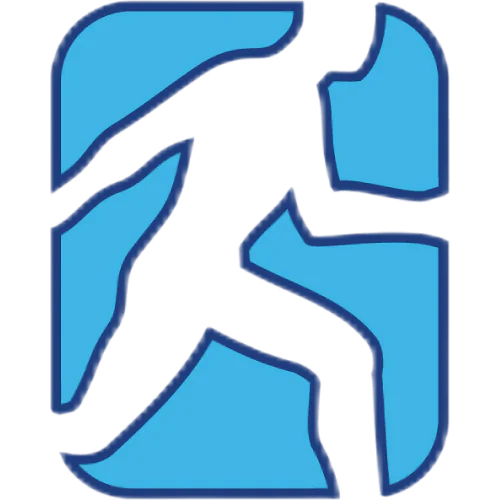
Criterion Validity of Accelerometers in Determining Knee-Flexion Angles During Sitting in a Laboratory Setting
Wu Y., O’Brien M.W., Peddle A., Daley W.S., Schwartz B.D., Kimmerly D.S., Frayne R.J.
Introduction: Device-based monitors often classify all sedentary positions as the sitting posture, but sitting with bent or straight legs may exhibit unique physiological and biomechanical effects. The classifications of the specific nuances of sitting have not been understood. The purpose of this study was to validate a dual-monitor approach from a trimonitor configuration measuring knee-flexion angles compared to motion capture (criterion) during sitting in laboratory setting. Methods: Nineteen adults (12♀, 24 ± 4 years) wore three activPALs (torso, thigh, tibia) while 14 motion capture cameras simultaneously tracked 15 markers located on bony landmarks. Each participant completed a 45-s supine resting period and eight, 45-s seated trials at different knee flexion angles (15° increment between 0° and 105°, determined via goniometry), followed by 15 s of standing. Validity was assessed via Friedman’s test (adjusted p value = .006), mean absolute error, Bland–Altman analyses, equivalence testing, and intraclass correlation. Results: Compared to motion capture, the calculated angles from activPALs were not different during 15°–90° (all, p ≥ .009), underestimated at 105° (p = .002) and overestimated at 0°, as well as the supine position (both, p < .001). Knee angles between 15° and 105° exhibited a mean absolute error of ∼5°, but knee angles <15° exhibited larger degrees of error (∼10°). A proportional (β = −0.12, p < .001) bias was observed, but a fixed (0.5° ± 1.7°, p = .405) bias did not exist. In equivalence testing, the activPALs were statistically equivalent to motion capture across 30°–105°. Strong agreement between the activPALs and motion capture was observed (intraclass correlation = .97, p < .001). Conclusions: The usage of a three-activPAL configuration detecting seated knee-flexion angles in free-living conditions is promising.
Influence of Accelerometer Calibration on the Estimation of Objectively Measured Physical Activity: The Tromsø Study
Weitz M., Morseth B., Hopstock L.A., Horsch A.
Accelerometers are increasingly used to observe human behavior such as physical activity under free-living conditions. An important prerequisite to obtain reliable results is the correct calibration of the sensors. However, accurate calibration is often neglected, leading to potentially biased results. Here, we demonstrate and quantify the effect of accelerometer miscalibration on the estimation of objectively measured physical activity under free-living conditions. The total volume of moderate to vigorous physical activity (MVPA) was significantly reduced after post hoc auto-calibration for uniaxial and triaxial count data, as well as for Euclidean Norm Minus One and mean amplitude deviation raw data. Weekly estimates of MVPA were reduced on average by 5.5, 9.2, 45.8, and 4.8 min, respectively, when compared to the original uncalibrated estimates. Our results indicate a general trend of overestimating physical activity when using factory-calibrated sensors. In particular, the accuracy of estimates derived from the Euclidean Norm Minus One feature suffered from uncalibrated sensors. For all modalities, the more uncalibrated the sensor was, the more MVPA was overestimated. This might especially affect studies with lower sample sizes.
Comparative Analysis and Conversion Between Actiwatch and ActiGraph Open-Source Counts
Lee P.H., Neishabouri A., Tse A.C., Guo C.C.
Body-worn sensors have contributed to a rich and growing body of literature in public health and clinical research in the last decades. A major challenge in sensor research is the lack of consistency and standardization of the collection and reporting of the sensor data. The algorithms used to derive these activity counts can be vastly different between manufactures and not always transparent to the researchers. With Philips, one of the major research-grade wearable device manufacturers, discontinuing this product line, many researchers are left in need of alternative solutions and at the risk of not being able to relate their historical data using the Philips Actiwatch 2 devices to future findings with other devices. We herein provide a comparison analysis and conversion method that can be used to convert activity counts from Philips to those from ActiGraph, another major manufacturer who provide both raw acceleration data and count data based on their open-source algorithm to the research community. This work provides an approach to maximize the scientific value of historical actigraphy data collected by the Actiwatch devices to support research continuity in this community. The conversion, however, is not perfect and only offers an approximation, due to the intrinsic difference in the count algorithms between the two accelerometers, and the permanent information loss during data reduction. We encourage future research using body-worn sensors to retain the raw sensor data to ensure data consistency, comparability, and the ability to leverage future algorithm improvement.
Comparison of Sleep and Physical Activity Metrics From Wrist-Worn ActiGraph wGT3X-BT and GT9X Accelerometers During Free-Living in Adults
Buchan D.S.
Background: ActiGraph accelerometers can monitor sleep and physical activity (PA) during free-living, but there is a need to confirm agreement in outcomes between different models. Methods: Sleep and PA metrics from two ActiGraphs were compared after participants (N = 30) wore a GT9X and wGT3X-BT on their nondominant wrist for 7 days during free-living. PA metrics including total steps, counts, average acceleration—Euclidean Norm Minus One (ENMO) and Mean Amplitude Deviation, intensity gradient, the minimum acceleration value of the most active 10 and 30 min (M10, M30), time spent in activity intensities from vector magnitude (VM) counts, and ENMO cut points and sleep metrics (sleep period time window, sleep duration, sleep onset, and waking time) were compared. Results: Excellent agreement was evident for average acceleration-Mean Amplitude Deviation, counts, total steps, M10, and light PA (VM counts) with good agreement evident from the remaining PA metrics apart from moderate–vigorous PA (VM counts) which demonstrated moderate agreement. Mean bias for all PA metrics were low, as were the limits of agreement for the intensity gradient, average acceleration-Mean Amplitude Deviation, and inactive time (ENMO and VM counts). The limits of agreement for all other PA metrics were >10%. Excellent agreement, low mean bias, and narrow limits of agreement were evident for all sleep metrics. All sleep and PA metrics demonstrated equivalence (equivalence zone of ≤10%) apart from moderate–vigorous PA (ENMO) which needed an equivalence zone of 16%. Conclusions: Equivalent estimates of almost all PA and sleep metrics are provided from the GT9X and wGT3X-BT worn on the nondominant wrist.
A Self-Paced Walk Test for Individual Calibration of Heart Rate to Energy Expenditure
Westgate K., Gonzales T.I., Hollidge S., Lindsay T., Wareham N., Brage S.
Introduction: Estimating free-living physical activity (PA) with continuous heart rate (HR) monitoring is challenging due to individual variation in the relationship between HR and energy expenditure. This variation can be captured through individual calibration with graded exercise tests, but structured tests with prescribed load require medical screening and are not always feasible in population settings. We present and evaluate an individual calibration method using HR response to a less demanding self-paced walk test. Methods: Six hundred and forty-three participants from the Fenland Study (Cambridgeshire, the United Kingdom) completed a 200-m self-paced walk test, a treadmill test, and 1 week of continuous HR and accelerometry monitoring. Mixed-effects regression was used to derive a walk test calibration model from HR response to the walk using treadmill-based parameters as criterion. Free-living PA estimates from the calibration model were compared with treadmill-calibrated and non-exercise-calibrated estimates. Results: Walk calibration captured 57% of the variance in the HR–energy expenditure relationship determined by the treadmill test. Applying walk calibration to data from free-living yielded similar PA estimates to those using treadmill calibration (52.7 vs. 52.0 kJ·kg−1·day−1; mean difference: 0.7 kJ·kg−1·day−1, 95% confidence interval [−0.0, 1.5]) and high correlation (r = .89). Individual differences were observed (root mean square error: 10.0 kJ·kg−1·day−1; 95% limits of agreement: −20.6, 19.1 kJ·kg−1·day−1). Walk calibration improved precision by 29% compared with nonexercise group calibration (root mean square error: 14.0 kJ·kg−1·day−1; 95% limits of agreement: −30.4, 24.5 kJ·kg−1·day−1). Conclusions: A 200-m self-paced walk test captures between-individual variation in the HR–energy expenditure relationship and facilitates estimation of free-living PA in population settings.
Comparability of 24-hr Activity Cycle Outputs From ActiGraph Counts Generated in ActiLife and RStudio
Montoye A.H., Clevenger K.A., Boudreaux B.D., Schmidt M.D.
Data from ActiGraph accelerometers have long been imported into ActiLife software, where the company’s proprietary “activity counts” were generated in order to understand physical behavior metrics. In 2022, ActiGraph released an open-source method to generate activity counts from any raw, triaxial accelerometer data using Python, which has been translated into RStudio packages. However, it is unclear if outcomes are comparable when generated in ActiLife and RStudio. Therefore, the authors’ technical note systematically compared activity counts and related physical behavior metrics generated from ActiGraph accelerometer data using ActiLife or available packages in RStudio and provides example code to ease implementation of such analyses in RStudio. In addition to comparing triaxial activity counts, physical behavior outputs (sleep, sedentary behavior, light-intensity physical activity, and moderate- to vigorous-intensity physical activity) were compared using multiple nonwear algorithms, epochs, cut points, sleep scoring algorithms, and accelerometer placement sites. Activity counts and physical behavior outcomes were largely the same between ActiLife and the tested packages in RStudio. However, peculiarities in the application of nonwear algorithms to the first and last portions of a data file (that occurred on partial, first or last days of data collection), differences in rounding, and handling of counts values on the borderline of activity intensities resulted in small but inconsequential differences in some files. The hope is that researchers and both hardware and software manufacturers continue to push efforts toward transparency in data analysis and interpretation, which will enhance comparability across devices and studies and help to advance fields examining links between physical behavior and health.
Pre- Versus Postmeal Sedentary Duration—Impact on Postprandial Glucose in Older Adults With Overweight or Obesity
Chun E., Gaynanova I., Melanson E.L., Lyden K.
Introduction: Reducing sedentary time is associated with improved postprandial glucose regulation. However, it is not known if the timing of sedentary behavior (i.e., pre- vs. postmeal) differentially impacts postprandial glucose in older adults with overweight or obesity. Methods: In this secondary analysis, older adults (≥65 years) with overweight and obesity (body mass index ≥ 25 kg/m2) wore a continuous glucose monitor and a sedentary behavior monitor continuously in their real-world environments for four consecutive days on four separate occasions. Throughout each 4-day measurement period, participants followed a standardized eucaloric diet and recorded mealtimes in a diary. Glucose, sedentary behavior, and meal intake data were fused using sensor and diary timestamps. Mixed-effect linear regression models were used to evaluate the impact of sedentary timing relative to meal intake. Results: Premeal sedentary time was significantly associated with both the increase from premeal glucose to the postmeal peak (ΔG) and the percent of premeal glucose increase that was recovered 1-hr postmeal glucose peak (%Baseline Recovery; p < .05), with higher levels of premeal sedentary time leading to both a larger ΔG and a smaller %Baseline Recovery. Postmeal sedentary time was significantly associated with the time from meal intake to glucose peak (ΔT; p < .05), with higher levels of postmeal sedentary time leading to a longer time to peak. Conclusions: Pre- versus postmeal sedentary behavior differentially impacts postprandial glucose response in older adults with overweight or obesity, suggesting that the timing of sedentary behavior reductions might play an influential role on long-term glycemic control.
The KID Study (Kids Interacting With Dogs): Piloting a Novel Approach for Measuring Dog-Facilitated Youth Physical Activity
Chase C.J., Burkart S., Potter K.
Background: Two-thirds of children in the United States do not meet the National Physical Activity Guidelines, leaving a majority at higher risk for negative health outcomes. Novel, effective children’s physical activity (PA) interventions are urgently needed. Dog-facilitated PA (e.g., dog walking and active play) is a promising intervention target, as dogs support many of the known correlates of children’s PA. There is a need for accurate methods of quantifying dog-facilitated PA. Purpose: The study purpose was to determine the feasibility and acceptability of a novel method for quantifying the volume and intensity of dog-facilitated PA among dog-owning children. Methods: Children and their dog(s) wore ActiGraph accelerometers with a Bluetooth proximity feature for 7 days. Additionally, parents logged child PA with the family dog(s). Total minutes of dog-facilitated PA and percentage of overall daily moderate to vigorous PA performed with the dog were calculated. Results: Twelve children (mean age = 7.8 ± 2.9 years) participated. There was high feasibility, with 100% retention, valid device data (at least 4 days ≥6-hr wear time), and completion of daily parent log and questionnaire packets. On average, dog-facilitated PA contributed 22.9% (9.2 min) and 15.1% (7.3 min) of the overall daily moderate to vigorous PA for children according to Bluetooth proximity data and parent report, respectively. Conclusions: This pilot study demonstrated the feasibility of utilizing an accelerometer with a proximity feature to quantify dog-facilitated PA. Future research should use this protocol with a larger, more diverse sample to determine whether dog-facilitated PA contributes a clinically significant amount toward overall PA in dog-owning youth.
Context Matters: The Importance of Physical Activity Domains for Public Health
Quinn T.D., Barone Gibbs B.
Physical activity can be performed across several domains, including leisure, occupation, household, and transportation, but physical activity research, measurement, and surveillance have historically been focused on leisure-time physical activity. Emerging evidence suggests differential health effects across these domains. In particular, occupational physical activity may be associated with adverse health outcomes. We argue that to adequately consider and evaluate such impacts, physical activity researchers and public health practitioners engaging in measurement, surveillance, and guideline creation should measure and consider all relevant physical activity domains where possible. We describe why physical activity science is often limited to the leisure-time domain and provide a rationale for expanding research and public health efforts to include all physical activity domains.
Evaluation of Physical Activity Assessment Using a Triaxial Activity Monitor in Community-Dwelling Older Japanese Adults With and Without Lifestyle-Related Diseases
Nagayoshi S., Yatsugi H., Liu X., Saito T., Yamatsu K., Kishimoto H.
Background: Several previous studies investigated physical activity of older adults using wearable devices, but more studies need to develop normative values for chronic disease conditions. This study aimed to investigate physical activity using a triaxial activity monitor in community-dwelling older Japanese adults with and without lifestyle-related diseases. Methods: Data from a total of 732 community-dwelling older Japanese men and women were collected and analyzed in a cross-sectional study. The participants’ physical activity was assessed for seven consecutive days by a triaxial accelerometer. Physical activity was assessed by number of lifestyle-related diseases and six lifestyle-related diseases categories by gender. Physical activity was assessed separately for total, locomotive, and nonlocomotive physical activity. Results: Participants with multiple (two or more) diseases had significantly lower total light-intensity physical activity (LPA; 278.5 ± 8.4 min/day) and nonlocomotive LPA (226.4 ± 7.0 min/day) versus without diseases in men. Compared in each disease category, total LPA and nonlocomotive LPA was significantly lower in men with hypertension and diabetes. Total sedentary time was significantly higher in men with hypertension, diabetes, and heart disease. Locomotive LPA was significantly lower in men with diabetes. In women, locomotive moderate- to vigorous-intensity physical activity was significantly higher in women with diabetes, and nonlocomotive moderate- to vigorous-intensity physical activity was significantly lower in women with heart disease. Conclusion: This study demonstrated that older Japanese men with multiple lifestyle-related diseases had lower physical activity. In each disease category, hypertension, diabetes, and heart disease affected lower physical activity, especially in men.
Reliability and Validity of the Repetition-Counting Feature of the Push Band 2.0 at Different Repetition Speeds
VanZant R., Erickson J., Dewar M., Williams D., Schmidt M.D.
Purpose: To assess the interdevice reliability and validity of the repetition-counting feature of the Push Band 2.0. Methods: Thirty college-aged participants (aged 18–24 years) simultaneously wore two Push Band 2.0 devices and performed 10 common resistance training exercises at four different tempos over the course of two testing sessions. Twelve repetitions were completed with visual confirmation for each set of exercises and compared with repetition estimates from the Push Band 2.0. Interdevice reliability was quantified using single measures intraclass correlation coefficients with 95% confidence intervals while validity was assessed via mean absolute percent error and mean percent error. Results: Interdevice reliability was found to be good to very good regardless of exercise type or tempo, as all intraclass correlation coefficients were >.770. Validity of the repetition-counting feature of the device was dependent on both exercise type and tempo, as exercises that did not involve rotation of the device throughout the movement demonstrated greater mean absolute percent error (31.0% average of all four tempos) and mean percent error (−29.9% average of all four tempos) than those that required such rotation (average mean absolute percent error of 3.5% and mean percent error of −1.6% across all four tempos). Conclusions: This study supports the reliability of the repetition-counting feature of the Push Band 2.0. However, device accuracy appears to be dependent on the type of movement and the speed at which the movement is performed, with greater accuracy observed during faster exercise tempos and exercises involving rotation of the device during movement execution.
Systematic Review of Accelerometer Responsiveness to Change for Measuring Physical Activity, Sedentary Behavior, or Sleep
Clevenger K.A., Montoye A.H.
Measurement of 24-hr movement behaviors is important for assessing adherence to guidelines, participation trends over time, group differences, and whether health-promoting interventions are successful. For a measurement tool to be useful, it must be valid, reliable, and able to detect change, the latter being a measurement property called responsiveness, sensitivity to change, or longitudinal validity. We systematically reviewed literature on the responsiveness of accelerometers to detect change in 24-hr movement behaviors. Databases (PubMed, Scopus, and EBSCOHost) were searched for peer-reviewed papers published in English between 1998 and 2023. Quality/risk of bias was assessed using a customized tool. This study is registered at https://osf.io/qrn8a. Twenty-six papers met the inclusion/exclusion criteria with an overall sample of 1,939 participants. Narrative synthesis was used. Most studies focused on adults (n = 21), and almost half (n = 12) included individuals with specific medical conditions. Studies primarily took place in free-living settings (n = 21) and used research-grade accelerometers (n = 24) worn on the hip (n = 18), thigh (n = 7), or wrist (n = 9). Outcomes included physical activity (n = 19), sedentary time/behavior (n = 12), or sleep (n = 2) and were calculated using proprietary formulas (e.g., Fitbit algorithm), cut points, and/or count-based methods. Most studies calculated responsiveness by comparing before versus after an intervention (n = 16). Six studies included a criterion measure to confirm that changes occurred. Limited research is available on the responsiveness of accelerometers for detecting change in 24-hr movement behaviors, particularly in youth populations, for sleep outcomes, and for commercial and thigh- or wrist-worn devices. Lack of a criterion measure precludes conclusions about the responsiveness even in more frequently studied outcomes/populations.
Understanding Physical Behaviors During Periods of Accelerometer Wear and Nonwear in College Students
Montoye A.H., Clevenger K.A., Boudreaux B.D., Schmidt M.D.
Accelerometers are increasingly used to measure 24-hr movement behaviors but are sometimes removed intermittently (e.g., for sleep or bathing), resulting in missing data. This study compared physical behaviors between times a hip-placed accelerometer was worn versus not worn in a college student sample. Participants (n = 115) wore a hip-placed ActiGraph during waking times and a thigh-placed activPAL continuously for at least 7 days (mean ± SD 7.5 ± 1.1 days). Thirteen nonwear algorithms determined ActiGraph nonwear; days included in the analysis had to have at least 1 min where the ActiGraph classified nonwear while participant was classified as awake by the activPAL. activPAL data for steps, time in sedentary behaviors (SB), light-intensity physical activity (LPA), and moderate- to vigorous-intensity physical activity (MVPA) from ActiGraph wear times were then compared with activPAL data from ActiGraph nonwear times. Participants took more steps (10.2–11.8 steps/min) and had higher proportions of MVPA (5.0%–5.9%) during ActiGraph wear time than nonwear time (3.1–8.0 steps/min, 0.8%–1.3% in MVPA). Effects were variable for SB (62.6%–66.9% of wear, 45.5%–76.2% of nonwear) and LPA (28.2%–31.5% of wear, 23.0%–53.2% of nonwear) depending on nonwear algorithm. Rescaling to a 12-hr day reduced SB and LPA error but increased MVPA error. Requiring minimum wear time (e.g., 600 min/day) reduced error but resulted in 10%–22% of days removed as invalid. In conclusion, missing data had minimal effect on MVPA but resulted in underestimation of SB and LPA. Strategies like scaling SB and LPA, but not MVPA, may improve physical behavior estimates from incomplete accelerometer data.
Determinants of Consent in the SHARE Accelerometer Study
Franzese F., Schrank F., Bergmann M.
The eighth wave of the Survey of Health, Aging and Retirement in Europe comprises a subsample of respondents who were asked to participate in a measurement of physical activity using thigh-worn accelerometers. This paper describes the process for obtaining consent, identifies determinants of consent, and analyzes the aggregated results of the accelerometer measurements for bias due to sample selection. The overall consent rate in the Survey of Health, Aging and Retirement in Europe accelerometer study was 54%, with variations between countries ranging from 34% to 70%. Multivariate logistic regressions show that various factors are correlated with consent such as respondents’ age, self-reported moderate activity, computer literacy, willingness to answer questions, and the interviewers’ age. After introducing inverse probability weights, there appears to be only a small and insignificant influence of participant selection and consent.