Mohamed bin Zayed University of Artificial Intelligence
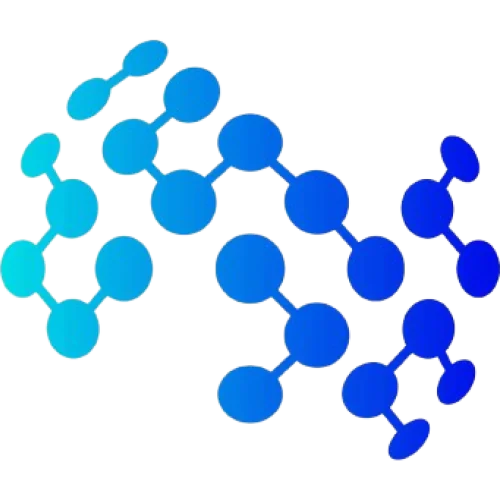
Since 2020
Total publications
643
Total citations
7798
Citations per publication
12.13
Average publications per year
128.6
Average authors per publication
5.38
h-index
43
Metrics description
h-index
A scientist has an h-index if h of his N publications are cited at least h times each, while the remaining (N - h) publications are cited no more than h times each.
Top-30
Fields of science
50
100
150
200
250
|
|
Computer Networks and Communications
|
Computer Networks and Communications, 203, 31.57%
Computer Networks and Communications
203 publications, 31.57%
|
Computer Science Applications
|
Computer Science Applications, 177, 27.53%
Computer Science Applications
177 publications, 27.53%
|
Electrical and Electronic Engineering
|
Electrical and Electronic Engineering, 143, 22.24%
Electrical and Electronic Engineering
143 publications, 22.24%
|
Software
|
Software, 126, 19.6%
Software
126 publications, 19.6%
|
Hardware and Architecture
|
Hardware and Architecture, 123, 19.13%
Hardware and Architecture
123 publications, 19.13%
|
Information Systems
|
Information Systems, 113, 17.57%
Information Systems
113 publications, 17.57%
|
Signal Processing
|
Signal Processing, 87, 13.53%
Signal Processing
87 publications, 13.53%
|
Artificial Intelligence
|
Artificial Intelligence, 78, 12.13%
Artificial Intelligence
78 publications, 12.13%
|
Computer Vision and Pattern Recognition
|
Computer Vision and Pattern Recognition, 39, 6.07%
Computer Vision and Pattern Recognition
39 publications, 6.07%
|
Applied Mathematics
|
Applied Mathematics, 37, 5.75%
Applied Mathematics
37 publications, 5.75%
|
Automotive Engineering
|
Automotive Engineering, 33, 5.13%
Automotive Engineering
33 publications, 5.13%
|
Control and Systems Engineering
|
Control and Systems Engineering, 30, 4.67%
Control and Systems Engineering
30 publications, 4.67%
|
Aerospace Engineering
|
Aerospace Engineering, 26, 4.04%
Aerospace Engineering
26 publications, 4.04%
|
Computational Theory and Mathematics
|
Computational Theory and Mathematics, 25, 3.89%
Computational Theory and Mathematics
25 publications, 3.89%
|
General Medicine
|
General Medicine, 23, 3.58%
General Medicine
23 publications, 3.58%
|
General Engineering
|
General Engineering, 23, 3.58%
General Engineering
23 publications, 3.58%
|
General Computer Science
|
General Computer Science, 22, 3.42%
General Computer Science
22 publications, 3.42%
|
General Materials Science
|
General Materials Science, 19, 2.95%
General Materials Science
19 publications, 2.95%
|
Human-Computer Interaction
|
Human-Computer Interaction, 14, 2.18%
Human-Computer Interaction
14 publications, 2.18%
|
Computer Graphics and Computer-Aided Design
|
Computer Graphics and Computer-Aided Design, 13, 2.02%
Computer Graphics and Computer-Aided Design
13 publications, 2.02%
|
Cognitive Neuroscience
|
Cognitive Neuroscience, 13, 2.02%
Cognitive Neuroscience
13 publications, 2.02%
|
Renewable Energy, Sustainability and the Environment
|
Renewable Energy, Sustainability and the Environment, 11, 1.71%
Renewable Energy, Sustainability and the Environment
11 publications, 1.71%
|
Media Technology
|
Media Technology, 11, 1.71%
Media Technology
11 publications, 1.71%
|
Control and Optimization
|
Control and Optimization, 9, 1.4%
Control and Optimization
9 publications, 1.4%
|
Modeling and Simulation
|
Modeling and Simulation, 9, 1.4%
Modeling and Simulation
9 publications, 1.4%
|
Management Science and Operations Research
|
Management Science and Operations Research, 9, 1.4%
Management Science and Operations Research
9 publications, 1.4%
|
Library and Information Sciences
|
Library and Information Sciences, 8, 1.24%
Library and Information Sciences
8 publications, 1.24%
|
Mechanical Engineering
|
Mechanical Engineering, 7, 1.09%
Mechanical Engineering
7 publications, 1.09%
|
Management Information Systems
|
Management Information Systems, 7, 1.09%
Management Information Systems
7 publications, 1.09%
|
Statistics, Probability and Uncertainty
|
Statistics, Probability and Uncertainty, 6, 0.93%
Statistics, Probability and Uncertainty
6 publications, 0.93%
|
50
100
150
200
250
|
Journals
10
20
30
40
50
60
70
80
90
|
|
Lecture Notes in Computer Science
84 publications, 13.06%
|
|
IEEE Internet of Things Journal
62 publications, 9.64%
|
|
IEEE Transactions on Vehicular Technology
27 publications, 4.2%
|
|
IEEE Network
20 publications, 3.11%
|
|
Proceedings of the AAAI Conference on Artificial Intelligence
20 publications, 3.11%
|
|
IEEE Transactions on Pattern Analysis and Machine Intelligence
19 publications, 2.95%
|
|
IEEE Access
16 publications, 2.49%
|
|
IEEE Transactions on Network and Service Management
12 publications, 1.87%
|
|
IEEE Wireless Communications
11 publications, 1.71%
|
|
IEEE Transactions on Network Science and Engineering
10 publications, 1.56%
|
|
IEEE Transactions on Image Processing
10 publications, 1.56%
|
|
IEEE Transactions on Mobile Computing
10 publications, 1.56%
|
|
IEEE Transactions on Wireless Communications
10 publications, 1.56%
|
|
IEEE Transactions on Neural Networks and Learning Systems
9 publications, 1.4%
|
|
Signals and Communication Technology
9 publications, 1.4%
|
|
Neurocomputing
8 publications, 1.24%
|
|
Wireless Networks
8 publications, 1.24%
|
|
IEEE Transactions on Intelligent Transportation Systems
7 publications, 1.09%
|
|
Synthesis Lectures on Human Language Technologies
7 publications, 1.09%
|
|
Pattern Recognition
6 publications, 0.93%
|
|
IEEE Consumer Electronics Magazine
6 publications, 0.93%
|
|
IEEE Communications Surveys & Tutorials
6 publications, 0.93%
|
|
IEEE Transactions on Multimedia
5 publications, 0.78%
|
|
IEEE Transactions on Industrial Informatics
5 publications, 0.78%
|
|
IEEE Transactions on Communications
5 publications, 0.78%
|
|
IEEE Transactions on Knowledge and Data Engineering
5 publications, 0.78%
|
|
Information Fusion
4 publications, 0.62%
|
|
IEEE Communications Magazine
4 publications, 0.62%
|
|
Neural Computing and Applications
4 publications, 0.62%
|
|
Knowledge-Based Systems
4 publications, 0.62%
|
|
10
20
30
40
50
60
70
80
90
|
Publishers
50
100
150
200
250
300
350
|
|
IEEE
316 publications, 49.14%
|
|
Springer Nature
161 publications, 25.04%
|
|
Elsevier
73 publications, 11.35%
|
|
Multidisciplinary Digital Publishing Institute (MDPI)
21 publications, 3.27%
|
|
Association for the Advancement of Artificial Intelligence (AAAI)
20 publications, 3.11%
|
|
Association for Computing Machinery (ACM)
14 publications, 2.18%
|
|
Taylor & Francis
7 publications, 1.09%
|
|
Wiley
4 publications, 0.62%
|
|
IOS Press
3 publications, 0.47%
|
|
Social Science Electronic Publishing
3 publications, 0.47%
|
|
Cambridge University Press
2 publications, 0.31%
|
|
Oxford University Press
2 publications, 0.31%
|
|
KeAi Communications Co.
2 publications, 0.31%
|
|
MIT Press
2 publications, 0.31%
|
|
IOP Publishing
2 publications, 0.31%
|
|
IGI Global
2 publications, 0.31%
|
|
Institution of Engineering and Technology (IET)
1 publication, 0.16%
|
|
Proceedings of the National Academy of Sciences (PNAS)
1 publication, 0.16%
|
|
Frontiers Media S.A.
1 publication, 0.16%
|
|
Mary Ann Liebert
1 publication, 0.16%
|
|
American Institute of Physics (AIP)
1 publication, 0.16%
|
|
Institute of Mathematical Statistics
1 publication, 0.16%
|
|
EPI SCP
1 publication, 0.16%
|
|
American Association for Artificial Intelligence (AAAI) Press
1 publication, 0.16%
|
|
JMIR Publications
1 publication, 0.16%
|
|
50
100
150
200
250
300
350
|
With other organizations
5
10
15
20
25
30
35
40
45
|
|
Inception Institute of Artificial Intelligence
41 publications, 6.38%
|
|
Hamad Bin Khalifa University
29 publications, 4.51%
|
|
University of Electronic Science and Technology of China
29 publications, 4.51%
|
|
Qatar University
21 publications, 3.27%
|
|
Sun Yat-sen University
21 publications, 3.27%
|
|
Xidian University
21 publications, 3.27%
|
|
Beijing University of Posts and Telecommunications
20 publications, 3.11%
|
|
Carnegie Mellon University
20 publications, 3.11%
|
|
Khalifa University
17 publications, 2.64%
|
|
Thapar Institute of Engineering and Technology
17 publications, 2.64%
|
|
Nanyang Technological University
16 publications, 2.49%
|
|
Guangdong University of Technology
15 publications, 2.33%
|
|
City University of Hong Kong
15 publications, 2.33%
|
|
Northwestern Polytechnical University
14 publications, 2.18%
|
|
Shenyang Aerospace University
14 publications, 2.18%
|
|
King Saud University
13 publications, 2.02%
|
|
Birla Institute of Technology and Science, Pilani
13 publications, 2.02%
|
|
Zayed University
12 publications, 1.87%
|
|
Harbin Institute of Technology
12 publications, 1.87%
|
|
University of Glasgow
12 publications, 1.87%
|
|
Moscow Institute of Physics and Technology
11 publications, 1.71%
|
|
China University of Petroleum (East China)
11 publications, 1.71%
|
|
Ajman University of Science and Technology
10 publications, 1.56%
|
|
Zhejiang University
10 publications, 1.56%
|
|
East China Normal University
10 publications, 1.56%
|
|
Linköping University
9 publications, 1.4%
|
|
Shenzhen University
9 publications, 1.4%
|
|
Liverpool John Moores University
9 publications, 1.4%
|
|
George Mason University
9 publications, 1.4%
|
|
Technology Innovation Institute
8 publications, 1.24%
|
|
5
10
15
20
25
30
35
40
45
|
With foreign organizations
5
10
15
20
25
30
|
|
Hamad Bin Khalifa University
29 publications, 4.51%
|
|
University of Electronic Science and Technology of China
29 publications, 4.51%
|
|
Qatar University
21 publications, 3.27%
|
|
Sun Yat-sen University
21 publications, 3.27%
|
|
Xidian University
21 publications, 3.27%
|
|
Beijing University of Posts and Telecommunications
20 publications, 3.11%
|
|
Carnegie Mellon University
20 publications, 3.11%
|
|
Thapar Institute of Engineering and Technology
17 publications, 2.64%
|
|
Nanyang Technological University
16 publications, 2.49%
|
|
Guangdong University of Technology
15 publications, 2.33%
|
|
City University of Hong Kong
15 publications, 2.33%
|
|
Northwestern Polytechnical University
14 publications, 2.18%
|
|
Shenyang Aerospace University
14 publications, 2.18%
|
|
King Saud University
13 publications, 2.02%
|
|
Birla Institute of Technology and Science, Pilani
13 publications, 2.02%
|
|
Harbin Institute of Technology
12 publications, 1.87%
|
|
University of Glasgow
12 publications, 1.87%
|
|
Moscow Institute of Physics and Technology
11 publications, 1.71%
|
|
China University of Petroleum (East China)
11 publications, 1.71%
|
|
Zhejiang University
10 publications, 1.56%
|
|
East China Normal University
10 publications, 1.56%
|
|
Linköping University
9 publications, 1.4%
|
|
Shenzhen University
9 publications, 1.4%
|
|
Liverpool John Moores University
9 publications, 1.4%
|
|
George Mason University
9 publications, 1.4%
|
|
University of Kufa
8 publications, 1.24%
|
|
Beihang University
8 publications, 1.24%
|
|
Nanjing University of Aeronautics and Astronautics
8 publications, 1.24%
|
|
Nanjing University of Information Science and Technology
8 publications, 1.24%
|
|
Nankai University
8 publications, 1.24%
|
|
5
10
15
20
25
30
|
With other countries
50
100
150
200
250
300
350
|
|
China
|
China, 312, 48.52%
China
312 publications, 48.52%
|
USA
|
USA, 135, 21%
USA
135 publications, 21%
|
United Kingdom
|
United Kingdom, 66, 10.26%
United Kingdom
66 publications, 10.26%
|
Canada
|
Canada, 61, 9.49%
Canada
61 publications, 9.49%
|
India
|
India, 51, 7.93%
India
51 publications, 7.93%
|
Australia
|
Australia, 38, 5.91%
Australia
38 publications, 5.91%
|
Qatar
|
Qatar, 35, 5.44%
Qatar
35 publications, 5.44%
|
Saudi Arabia
|
Saudi Arabia, 31, 4.82%
Saudi Arabia
31 publications, 4.82%
|
Japan
|
Japan, 28, 4.35%
Japan
28 publications, 4.35%
|
Singapore
|
Singapore, 23, 3.58%
Singapore
23 publications, 3.58%
|
Republic of Korea
|
Republic of Korea, 22, 3.42%
Republic of Korea
22 publications, 3.42%
|
Russia
|
Russia, 18, 2.8%
Russia
18 publications, 2.8%
|
Pakistan
|
Pakistan, 17, 2.64%
Pakistan
17 publications, 2.64%
|
Sweden
|
Sweden, 17, 2.64%
Sweden
17 publications, 2.64%
|
Germany
|
Germany, 15, 2.33%
Germany
15 publications, 2.33%
|
Jordan
|
Jordan, 14, 2.18%
Jordan
14 publications, 2.18%
|
Netherlands
|
Netherlands, 13, 2.02%
Netherlands
13 publications, 2.02%
|
Kuwait
|
Kuwait, 12, 1.87%
Kuwait
12 publications, 1.87%
|
Portugal
|
Portugal, 11, 1.71%
Portugal
11 publications, 1.71%
|
Iraq
|
Iraq, 11, 1.71%
Iraq
11 publications, 1.71%
|
Finland
|
Finland, 11, 1.71%
Finland
11 publications, 1.71%
|
France
|
France, 10, 1.56%
France
10 publications, 1.56%
|
Italy
|
Italy, 10, 1.56%
Italy
10 publications, 1.56%
|
Switzerland
|
Switzerland, 9, 1.4%
Switzerland
9 publications, 1.4%
|
Lebanon
|
Lebanon, 8, 1.24%
Lebanon
8 publications, 1.24%
|
Poland
|
Poland, 7, 1.09%
Poland
7 publications, 1.09%
|
Egypt
|
Egypt, 6, 0.93%
Egypt
6 publications, 0.93%
|
Spain
|
Spain, 6, 0.93%
Spain
6 publications, 0.93%
|
Palestine
|
Palestine, 6, 0.93%
Palestine
6 publications, 0.93%
|
Turkey
|
Turkey, 6, 0.93%
Turkey
6 publications, 0.93%
|
50
100
150
200
250
300
350
|
- We do not take into account publications that without a DOI.
- Statistics recalculated daily.
- Publications published earlier than 2020 are ignored in the statistics.
- The horizontal charts show the 30 top positions.
- Journals quartiles values are relevant at the moment.
{"yearsFrom":2020,"yearsTo":2024,"articlesYearsTotal":5,"articlesYearsFrom":2020,"articlesYearsTo":2024,"citationsYearsTotal":5,"citationsYearsFrom":0,"citationsYearsTo":0,"totalCitations":7798,"totalPublications":643,"averageCitations":"12.13","averagePublicationsPerYear":"128.6","averageAuthors":"5.38","hIndex":43,"iTenIndex":146,"mIndex":"8.6","oIndex":"177.05","gIndex":75,"wIndex":10,"journals":{"type":"bar","data":{"show":true,"labels":["Lecture Notes in Computer Science","IEEE Internet of Things Journal","IEEE Transactions on Vehicular Technology","IEEE Network","Proceedings of the AAAI Conference on Artificial Intelligence","IEEE Transactions on Pattern Analysis and Machine Intelligence","IEEE Access","IEEE Transactions on Network and Service Management","IEEE Wireless Communications","IEEE Transactions on Network Science and Engineering","IEEE Transactions on Image Processing","IEEE Transactions on Mobile Computing","IEEE Transactions on Wireless Communications","IEEE Transactions on Neural Networks and Learning Systems","Signals and Communication Technology","Neurocomputing","Wireless Networks","IEEE Transactions on Intelligent Transportation Systems","Synthesis Lectures on Human Language Technologies","Pattern Recognition","IEEE Consumer Electronics Magazine","IEEE Communications Surveys & Tutorials","IEEE Transactions on Multimedia","IEEE Transactions on Industrial Informatics","IEEE Transactions on Communications","IEEE Transactions on Knowledge and Data Engineering","Information Fusion","IEEE Communications Magazine","Neural Computing and Applications","Knowledge-Based Systems"],"ids":[1022,8752,7575,17451,26599,6856,25260,7986,18533,5236,18162,20462,22533,1084,6072,4817,7693,5270,23141,12523,23122,62789,7691,8342,9193,16548,2828,2921,5072,5293],"codes":[0,0,0,0,0,0,0,0,0,0,0,0,0,0,0,0,0,0,0,0,0,0,0,0,0,0,0,0,0,0],"imageUrls":["\/storage\/images\/resized\/voXLqlsvTwv5p3iMQ8Dhs95nqB4AXOG7Taj7G4ra_medium.webp","\/storage\/images\/resized\/6scCJegesojp2jubwY3uKCzTAmgsaH2GIFlg6Hfk_medium.webp","\/storage\/images\/resized\/6scCJegesojp2jubwY3uKCzTAmgsaH2GIFlg6Hfk_medium.webp","\/storage\/images\/resized\/6scCJegesojp2jubwY3uKCzTAmgsaH2GIFlg6Hfk_medium.webp","\/storage\/images\/resized\/tl1HPxf9fROJhpcIdh2hoKw0ud2lMABjjcEHvVa5_medium.webp","\/storage\/images\/resized\/6scCJegesojp2jubwY3uKCzTAmgsaH2GIFlg6Hfk_medium.webp","\/storage\/images\/resized\/6scCJegesojp2jubwY3uKCzTAmgsaH2GIFlg6Hfk_medium.webp","\/storage\/images\/resized\/6scCJegesojp2jubwY3uKCzTAmgsaH2GIFlg6Hfk_medium.webp","\/storage\/images\/resized\/6scCJegesojp2jubwY3uKCzTAmgsaH2GIFlg6Hfk_medium.webp","\/storage\/images\/resized\/6scCJegesojp2jubwY3uKCzTAmgsaH2GIFlg6Hfk_medium.webp","\/storage\/images\/resized\/6scCJegesojp2jubwY3uKCzTAmgsaH2GIFlg6Hfk_medium.webp","\/storage\/images\/resized\/6scCJegesojp2jubwY3uKCzTAmgsaH2GIFlg6Hfk_medium.webp","\/storage\/images\/resized\/6scCJegesojp2jubwY3uKCzTAmgsaH2GIFlg6Hfk_medium.webp","\/storage\/images\/resized\/6scCJegesojp2jubwY3uKCzTAmgsaH2GIFlg6Hfk_medium.webp","\/storage\/images\/resized\/voXLqlsvTwv5p3iMQ8Dhs95nqB4AXOG7Taj7G4ra_medium.webp","\/storage\/images\/resized\/GDnYOu1UpMMfMMRV6Aqle4H0YLLsraeD9IP9qScG_medium.webp","\/storage\/images\/resized\/voXLqlsvTwv5p3iMQ8Dhs95nqB4AXOG7Taj7G4ra_medium.webp","\/storage\/images\/resized\/6scCJegesojp2jubwY3uKCzTAmgsaH2GIFlg6Hfk_medium.webp","","\/storage\/images\/resized\/GDnYOu1UpMMfMMRV6Aqle4H0YLLsraeD9IP9qScG_medium.webp","\/storage\/images\/resized\/6scCJegesojp2jubwY3uKCzTAmgsaH2GIFlg6Hfk_medium.webp","\/storage\/images\/resized\/6scCJegesojp2jubwY3uKCzTAmgsaH2GIFlg6Hfk_medium.webp","\/storage\/images\/resized\/6scCJegesojp2jubwY3uKCzTAmgsaH2GIFlg6Hfk_medium.webp","\/storage\/images\/resized\/6scCJegesojp2jubwY3uKCzTAmgsaH2GIFlg6Hfk_medium.webp","\/storage\/images\/resized\/6scCJegesojp2jubwY3uKCzTAmgsaH2GIFlg6Hfk_medium.webp","\/storage\/images\/resized\/6scCJegesojp2jubwY3uKCzTAmgsaH2GIFlg6Hfk_medium.webp","\/storage\/images\/resized\/GDnYOu1UpMMfMMRV6Aqle4H0YLLsraeD9IP9qScG_medium.webp","\/storage\/images\/resized\/6scCJegesojp2jubwY3uKCzTAmgsaH2GIFlg6Hfk_medium.webp","\/storage\/images\/resized\/voXLqlsvTwv5p3iMQ8Dhs95nqB4AXOG7Taj7G4ra_medium.webp","\/storage\/images\/resized\/GDnYOu1UpMMfMMRV6Aqle4H0YLLsraeD9IP9qScG_medium.webp"],"datasets":[{"label":"","data":[84,62,27,20,20,19,16,12,11,10,10,10,10,9,9,8,8,7,7,6,6,6,5,5,5,5,4,4,4,4],"backgroundColor":["#3B82F6","#3B82F6","#3B82F6","#3B82F6","#3B82F6","#3B82F6","#3B82F6","#3B82F6","#3B82F6","#3B82F6","#3B82F6","#3B82F6","#3B82F6","#3B82F6","#3B82F6","#3B82F6","#3B82F6","#3B82F6","#3B82F6","#3B82F6","#3B82F6","#3B82F6","#3B82F6","#3B82F6","#3B82F6","#3B82F6","#3B82F6","#3B82F6","#3B82F6","#3B82F6"],"percentage":[13.06,9.64,4.2,3.11,3.11,2.95,2.49,1.87,1.71,1.56,1.56,1.56,1.56,1.4,1.4,1.24,1.24,1.09,1.09,0.93,0.93,0.93,0.78,0.78,0.78,0.78,0.62,0.62,0.62,0.62],"barThickness":13}]},"options":{"indexAxis":"y","maintainAspectRatio":false,"scales":{"y":{"ticks":{"precision":0,"autoSkip":false,"font":{"family":"Montserrat"},"color":"#000000"},"stacked":false},"x":{"ticks":{"stepSize":null,"precision":0,"font":{"family":"Montserrat"},"color":"#000000"},"stacked":false}},"plugins":{"legend":{"position":"top","labels":{"font":{"family":"Montserrat"},"color":"#000000"}},"title":{"display":true,"text":"Journals","font":{"size":24,"family":"Montserrat","weight":600},"color":"#000000"}}}},"openAccess":{"type":"pie","data":{"show":true,"labels":["Closed access","Open access"],"datasets":[{"label":"Open access","data":[499,144],"backgroundColor":["#E5E7EB","#f68212"],"hoverOffset":4,"percentage":[77.6,22.4]}]},"options":{"plugins":{"legend":{"position":"top","labels":{"font":{"family":"Montserrat"},"color":"#000000"}},"title":{"display":true,"text":"Open access","font":{"size":24,"family":"Montserrat","weight":600},"color":"#000000"}}}},"publishers":{"type":"bar","data":{"show":true,"labels":["IEEE","Springer Nature","Elsevier","Multidisciplinary Digital Publishing Institute (MDPI)","Association for the Advancement of Artificial Intelligence (AAAI)","Association for Computing Machinery (ACM)","Taylor & Francis","Wiley","IOS Press","Social Science Electronic Publishing","Cambridge University Press","Oxford University Press","KeAi Communications Co.","MIT Press","IOP Publishing","IGI Global","Institution of Engineering and Technology (IET)","Proceedings of the National Academy of Sciences (PNAS)","Frontiers Media S.A.","Mary Ann Liebert","American Institute of Physics (AIP)","Institute of Mathematical Statistics","EPI SCP","American Association for Artificial Intelligence (AAAI) Press","JMIR Publications"],"ids":[6953,8,17,202,7114,1141,18,11,29,7090,1,19,326,625,2075,7845,117,162,208,230,250,312,1435,3881,7126],"codes":[0,0,0,0,0,0,0,0,0,0,0,0,0,0,0,0,0,0,0,0,0,0,0,0,0],"imageUrls":["\/storage\/images\/resized\/6scCJegesojp2jubwY3uKCzTAmgsaH2GIFlg6Hfk_medium.webp","\/storage\/images\/resized\/voXLqlsvTwv5p3iMQ8Dhs95nqB4AXOG7Taj7G4ra_medium.webp","\/storage\/images\/resized\/GDnYOu1UpMMfMMRV6Aqle4H0YLLsraeD9IP9qScG_medium.webp","\/storage\/images\/resized\/MjH1ITP7lMYGxeqUZfkt2BnVLgjkk413jwBV97XX_medium.webp","\/storage\/images\/resized\/tl1HPxf9fROJhpcIdh2hoKw0ud2lMABjjcEHvVa5_medium.webp","\/storage\/images\/resized\/XZDD1UbkaHV0BImS1Dm7kQfvovjiljgbqNi7vyqK_medium.webp","\/storage\/images\/resized\/5YZtvLvkPZuc2JHOaZsjCvGSHFCuC3drUwN3YAc5_medium.webp","\/storage\/images\/resized\/bRyGpdm98BkAUYiK1YFNpl5Z7hPu6Gd87gbIeuG3_medium.webp","\/storage\/images\/resized\/KqWdDIHwzps3KNMz2kSZBC4SgMnkL3bwEKJqtZ2u_medium.webp","\/storage\/images\/resized\/VB6UIgqsyDBgrsTtqT4C2ImAHGDidVrMrprJV4LS_medium.webp","\/storage\/images\/resized\/cF81zWlLdkmYekymGuDcSdvgBGNKaIDoMoeJtHS1_medium.webp","\/storage\/images\/resized\/yNSijlgQghQF53VZuyFLA30CKDe4j3HK74Vtpnxa_medium.webp","\/storage\/images\/resized\/jeWDR7NTA9Ab5MVz8Uqjl2RPCndzFjSyl0FaRAHg_medium.webp","\/storage\/images\/resized\/FBYohhLEm0mZo5SN1CXXUS2IkhaacOEqLilV21TH_medium.webp","\/storage\/images\/resized\/LsKy6OnmmmRGcAU6CZgWQvNiP1polbaSLNrN7zqj_medium.webp","\/storage\/images\/resized\/7FMNeB9SnnAZoNUlPrVuDf3sVkEkt74weJA9emBp_medium.webp","\/storage\/images\/resized\/aK6yGjkv01aMMh16lIwFe0Z7wTx4ksfzsYuogiOY_medium.webp","\/storage\/images\/resized\/mxFdPe9qujsfvfYfcN0QOclAiYORFb0xrRlwV8gs_medium.webp","\/storage\/images\/resized\/4QWA67eqfcfyOiA8Wk7YnqroHFqQbTsmDJUYTCTg_medium.webp","\/storage\/images\/resized\/Zy5YST233rzRbZP24I24MzZm64hz20ZV9qQopmMn_medium.webp","\/storage\/images\/resized\/ARM4e6URKRsbRZvIF0vFis9DjxGloBjnBYJXbHmZ_medium.webp","\/storage\/images\/resized\/RPoHbLn4QABHbHcI0L0bNeyoyNlynV20iMXUD1m9_medium.webp","","","\/storage\/images\/resized\/cRMehae9HOl9IXrL0Y5aSxEgqjYPaMefZzmyZtW8_medium.webp"],"datasets":[{"label":"","data":[316,161,73,21,20,14,7,4,3,3,2,2,2,2,2,2,1,1,1,1,1,1,1,1,1],"backgroundColor":["#3B82F6","#3B82F6","#3B82F6","#3B82F6","#3B82F6","#3B82F6","#3B82F6","#3B82F6","#3B82F6","#3B82F6","#3B82F6","#3B82F6","#3B82F6","#3B82F6","#3B82F6","#3B82F6","#3B82F6","#3B82F6","#3B82F6","#3B82F6","#3B82F6","#3B82F6","#3B82F6","#3B82F6","#3B82F6"],"percentage":[49.14,25.04,11.35,3.27,3.11,2.18,1.09,0.62,0.47,0.47,0.31,0.31,0.31,0.31,0.31,0.31,0.16,0.16,0.16,0.16,0.16,0.16,0.16,0.16,0.16],"barThickness":13}]},"options":{"indexAxis":"y","maintainAspectRatio":false,"scales":{"y":{"ticks":{"precision":0,"autoSkip":false,"font":{"family":"Montserrat"},"color":"#000000"},"stacked":false},"x":{"ticks":{"stepSize":null,"precision":0,"font":{"family":"Montserrat"},"color":"#000000"},"stacked":false}},"plugins":{"legend":{"position":"top","labels":{"font":{"family":"Montserrat"},"color":"#000000"}},"title":{"display":true,"text":"Publishers","font":{"size":24,"family":"Montserrat","weight":600},"color":"#000000"}}}},"quartiles":{"type":"pie","data":{"show":true,"labels":["Quartile not defined","Q1","Q2","Q3","Q4"],"datasets":[{"label":"Quartiles by SCImago","data":[43,439,59,85,17],"backgroundColor":["#E5E7EB","rgb(164, 207, 99)","rgb(232, 213, 89)","rgb(251, 163,83)","rgb(221,90,78)"],"hoverOffset":4,"percentage":[6.69,68.27,9.18,13.22,2.64]}]},"options":{"plugins":{"legend":{"position":"top","labels":{"font":{"family":"Montserrat"},"color":"#000000"}},"title":{"display":true,"text":"Quartiles by SCImago","font":{"size":24,"family":"Montserrat","weight":600},"color":"#000000"}}}},"quartilesWs":{"type":"pie","data":{"show":true,"labels":["Quartile not defined","Q1","Q2","Q3","Q4"],"datasets":[{"label":"Quartiles by WoS","data":[166,352,107,11,7],"backgroundColor":["#E5E7EB","rgb(164, 207, 99)","rgb(232, 213, 89)","rgb(251, 163,83)","rgb(221,90,78)"],"hoverOffset":4,"percentage":[25.82,54.74,16.64,1.71,1.09]}]},"options":{"plugins":{"legend":{"position":"top","labels":{"font":{"family":"Montserrat"},"color":"#000000"}},"title":{"display":true,"text":"Quartiles by WoS","font":{"size":24,"family":"Montserrat","weight":600},"color":"#000000"}}}},"quartilesByYears":{"type":"bar","data":{"show":true,"labels":[2020,2021,2022,2023,2024],"ids":[],"codes":[],"imageUrls":[],"datasets":[{"label":"Q4","backgroundColor":"rgb(221,90,78)","data":[0,0,2,13,2],"percentage":[0,0,1.37,5.35,1.09]},{"label":"Q3","backgroundColor":"rgb(251, 163,83)","data":[22,3,25,28,7],"percentage":[68.75,7.89,17.12,11.52,3.8]},{"label":"Q2","backgroundColor":"rgb(232, 213, 89)","data":[1,0,18,24,16],"percentage":[3.13,0,12.33,9.88,8.7]},{"label":"Q1","backgroundColor":"rgb(164, 207, 99)","data":[9,32,90,157,151],"percentage":[28.13,84.21,61.64,64.61,82.07]},{"label":"Quartile not defined","backgroundColor":"#E5E7EB","data":[0,3,11,21,8],"percentage":[0,7.89,7.53,8.64,4.35]}]},"options":{"indexAxis":"x","maintainAspectRatio":true,"scales":{"y":{"ticks":{"precision":0,"autoSkip":false,"font":{"family":"Montserrat"},"color":"#000000"},"stacked":true},"x":{"ticks":{"stepSize":1,"precision":0,"font":{"family":"Montserrat"},"color":"#000000"},"stacked":true}},"plugins":{"legend":{"position":"top","labels":{"font":{"family":"Montserrat"},"color":"#000000"}},"title":{"display":true,"text":"Quartiles by SCImago per year","font":{"size":24,"family":"Montserrat","weight":600},"color":"#000000"}}}},"quartilesWsByYears":{"type":"bar","data":{"show":true,"labels":[2020,2021,2022,2023,2024],"ids":[],"codes":[],"imageUrls":[],"datasets":[{"label":"Q4","backgroundColor":"rgb(221,90,78)","data":[0,0,1,4,2],"percentage":[0,0,0.68,1.65,1.09]},{"label":"Q3","backgroundColor":"rgb(251, 163,83)","data":[0,0,3,6,2],"percentage":[0,0,2.05,2.47,1.09]},{"label":"Q2","backgroundColor":"rgb(232, 213, 89)","data":[1,11,18,51,26],"percentage":[3.13,28.95,12.33,20.99,14.13]},{"label":"Q1","backgroundColor":"rgb(164, 207, 99)","data":[9,19,85,110,129],"percentage":[28.13,50,58.22,45.27,70.11]},{"label":"Quartile not defined","backgroundColor":"#E5E7EB","data":[22,8,39,72,25],"percentage":[68.75,21.05,26.71,29.63,13.59]}]},"options":{"indexAxis":"x","maintainAspectRatio":true,"scales":{"y":{"ticks":{"precision":0,"autoSkip":false,"font":{"family":"Montserrat"},"color":"#000000"},"stacked":true},"x":{"ticks":{"stepSize":1,"precision":0,"font":{"family":"Montserrat"},"color":"#000000"},"stacked":true}},"plugins":{"legend":{"position":"top","labels":{"font":{"family":"Montserrat"},"color":"#000000"}},"title":{"display":true,"text":"Quartiles by WoS per year","font":{"size":24,"family":"Montserrat","weight":600},"color":"#000000"}}}},"subjects":{"type":"bar","data":{"show":true,"labels":["Computer Networks and Communications","Computer Science Applications","Electrical and Electronic Engineering","Software","Hardware and Architecture","Information Systems","Signal Processing","Artificial Intelligence","Computer Vision and Pattern Recognition","Applied Mathematics","Automotive Engineering","Control and Systems Engineering","Aerospace Engineering","Computational Theory and Mathematics","General Medicine","General Engineering","General Computer Science","General Materials Science","Human-Computer Interaction","Computer Graphics and Computer-Aided Design","Cognitive Neuroscience","Renewable Energy, Sustainability and the Environment","Media Technology","Control and Optimization","Modeling and Simulation","Management Science and Operations Research","Library and Information Sciences","Mechanical Engineering","Management Information Systems","Statistics, Probability and Uncertainty"],"ids":[128,17,53,133,68,92,156,131,233,132,173,135,174,91,20,70,179,50,161,89,197,107,268,143,243,253,88,60,230,181],"codes":[0,0,0,0,0,0,0,0,0,0,0,0,0,0,0,0,0,0,0,0,0,0,0,0,0,0,0,0,0,0],"imageUrls":["","","","","","","","","","","","","","","","","","","","","","","","","","","","","",""],"datasets":[{"label":"","data":[203,177,143,126,123,113,87,78,39,37,33,30,26,25,23,23,22,19,14,13,13,11,11,9,9,9,8,7,7,6],"backgroundColor":["#3B82F6","#3B82F6","#3B82F6","#3B82F6","#3B82F6","#3B82F6","#3B82F6","#3B82F6","#3B82F6","#3B82F6","#3B82F6","#3B82F6","#3B82F6","#3B82F6","#3B82F6","#3B82F6","#3B82F6","#3B82F6","#3B82F6","#3B82F6","#3B82F6","#3B82F6","#3B82F6","#3B82F6","#3B82F6","#3B82F6","#3B82F6","#3B82F6","#3B82F6","#3B82F6"],"percentage":[31.57,27.53,22.24,19.6,19.13,17.57,13.53,12.13,6.07,5.75,5.13,4.67,4.04,3.89,3.58,3.58,3.42,2.95,2.18,2.02,2.02,1.71,1.71,1.4,1.4,1.4,1.24,1.09,1.09,0.93],"barThickness":13}]},"options":{"indexAxis":"y","maintainAspectRatio":false,"scales":{"y":{"ticks":{"precision":0,"autoSkip":false,"font":{"family":"Montserrat"},"color":"#000000"},"stacked":false},"x":{"ticks":{"stepSize":null,"precision":0,"font":{"family":"Montserrat"},"color":"#000000"},"stacked":false}},"plugins":{"legend":{"position":"top","labels":{"font":{"family":"Montserrat"},"color":"#000000"}},"title":{"display":true,"text":"Fields of science","font":{"size":24,"family":"Montserrat","weight":600},"color":"#000000"}}}},"yearsArticles":{"type":"bar","data":{"show":true,"labels":[2020,2021,2022,2023,2024],"ids":[0,0,0,0,0],"codes":[0,0,0,0,0],"imageUrls":["","","","",""],"datasets":[{"label":"Articles number","data":[32,38,146,243,184],"backgroundColor":["#3B82F6","#3B82F6","#3B82F6","#3B82F6","#3B82F6"],"percentage":[4.98,5.91,22.71,37.79,28.62],"barThickness":null}]},"options":{"indexAxis":"x","maintainAspectRatio":true,"scales":{"y":{"ticks":{"precision":0,"autoSkip":false,"font":{"family":"Montserrat"},"color":"#000000"},"stacked":false},"x":{"ticks":{"stepSize":1,"precision":0,"font":{"family":"Montserrat"},"color":"#000000"},"stacked":false}},"plugins":{"legend":{"position":"top","labels":{"font":{"family":"Montserrat"},"color":"#000000"}},"title":{"display":true,"text":"Articles per year","font":{"size":24,"family":"Montserrat","weight":600},"color":"#000000"}}}},"yearsCitations":{"type":"bar","data":{"show":true,"labels":[2020,2021,2022,2023,2024],"ids":[0,0,0,0,0],"codes":[0,0,0,0,0],"imageUrls":["","","","",""],"datasets":[{"label":"Citations number","data":[62,536,1479,3216,2494],"backgroundColor":["#3B82F6","#3B82F6","#3B82F6","#3B82F6","#3B82F6"],"percentage":[0.8,6.87,18.97,41.24,31.98],"barThickness":null}]},"options":{"indexAxis":"x","maintainAspectRatio":true,"scales":{"y":{"ticks":{"precision":0,"autoSkip":false,"font":{"family":"Montserrat"},"color":"#000000"},"stacked":false},"x":{"ticks":{"stepSize":1,"precision":0,"font":{"family":"Montserrat"},"color":"#000000"},"stacked":false}},"plugins":{"legend":{"position":"top","labels":{"font":{"family":"Montserrat"},"color":"#000000"}},"title":{"display":true,"text":"Citations per year","font":{"size":24,"family":"Montserrat","weight":600},"color":"#000000"}}}},"countries":{"type":"bar","data":{"show":true,"labels":["China","USA","United Kingdom","Canada","India","Australia","Qatar","Saudi Arabia","Japan","Singapore","Republic of Korea","Russia","Pakistan","Sweden","Germany","Jordan","Netherlands","Kuwait","Portugal","Iraq","Finland","France","Italy","Switzerland","Lebanon","Poland","Egypt","Spain","Palestine","Turkey"],"ids":[9,7,48,97,84,11,98,183,250,195,175,1,166,238,2,86,148,112,10,87,226,3,92,237,118,173,79,91,168,217],"codes":["cn","us","gb","ca","in","au","qa","sa","jp","sg","kr","ru","pk","se","de","jo","nl","kw","pt","iq","fi","fr","it","ch","lb","pl","eg","es","ps","tr"],"imageUrls":["","","","","","","","","","","","","","","","","","","","","","","","","","","","","",""],"datasets":[{"label":"","data":[312,135,66,61,51,38,35,31,28,23,22,18,17,17,15,14,13,12,11,11,11,10,10,9,8,7,6,6,6,6],"backgroundColor":["#3B82F6","#3B82F6","#3B82F6","#3B82F6","#3B82F6","#3B82F6","#3B82F6","#3B82F6","#3B82F6","#3B82F6","#3B82F6","#3B82F6","#3B82F6","#3B82F6","#3B82F6","#3B82F6","#3B82F6","#3B82F6","#3B82F6","#3B82F6","#3B82F6","#3B82F6","#3B82F6","#3B82F6","#3B82F6","#3B82F6","#3B82F6","#3B82F6","#3B82F6","#3B82F6"],"percentage":[48.52,21,10.26,9.49,7.93,5.91,5.44,4.82,4.35,3.58,3.42,2.8,2.64,2.64,2.33,2.18,2.02,1.87,1.71,1.71,1.71,1.56,1.56,1.4,1.24,1.09,0.93,0.93,0.93,0.93],"barThickness":13}]},"options":{"indexAxis":"y","maintainAspectRatio":false,"scales":{"y":{"ticks":{"precision":0,"autoSkip":false,"font":{"family":"Montserrat"},"color":"#000000"},"stacked":false},"x":{"ticks":{"stepSize":null,"precision":0,"font":{"family":"Montserrat"},"color":"#000000"},"stacked":false}},"plugins":{"legend":{"position":"top","labels":{"font":{"family":"Montserrat"},"color":"#000000"}},"title":{"display":true,"text":"With other countries","font":{"size":24,"family":"Montserrat","weight":600},"color":"#000000"}}}},"organizations":{"type":"bar","data":{"show":true,"labels":["Inception Institute of Artificial Intelligence","Hamad Bin Khalifa University","University of Electronic Science and Technology of China","Qatar University","Sun Yat-sen University","Xidian University","Beijing University of Posts and Telecommunications","Carnegie Mellon University","Khalifa University","Thapar Institute of Engineering and Technology","Nanyang Technological University","Guangdong University of Technology","City University of Hong Kong","Northwestern Polytechnical University","Shenyang Aerospace University","King Saud University","Birla Institute of Technology and Science, Pilani","Zayed University","Harbin Institute of Technology","University of Glasgow","Moscow Institute of Physics and Technology","China University of Petroleum (East China)","Ajman University of Science and Technology","Zhejiang University","East China Normal University","Link\u00f6ping University","Shenzhen University","Liverpool John Moores University","George Mason University","Technology Innovation Institute"],"ids":[3356,2424,2454,2434,2966,2989,2795,3447,1323,1679,3106,3326,4416,2453,3408,1260,1362,1339,2252,3741,10,2801,1352,2236,3012,2671,3065,3249,4467,1360],"codes":[0,0,0,0,0,0,0,0,0,0,0,0,0,0,0,0,0,0,0,0,0,0,0,0,0,0,0,0,0,0],"imageUrls":["\/storage\/images\/resized\/kwiELnIFJeUqWpnh8N47TVO1GXuqIWk4jPEji9iq_medium.webp","\/storage\/images\/resized\/nFNLC5V7pQW5kMG0bS5yD4RWBuZ0oxoPvyKHj6vL_medium.webp","\/storage\/images\/resized\/LcCanHnZPh0mBIzCPZ79rcwQuiKwpNh24pD6TOtd_medium.webp","\/storage\/images\/resized\/Vs9svwplpO16ogA4Qa1NBM55QdLHni2FJBdn63Fj_medium.webp","\/storage\/images\/resized\/YnpZacG3TRTWCTE9ip17nwyzaWfGybATOPIThnax_medium.webp","\/storage\/images\/resized\/QygBwxzSppJCzVvOK3Fg19SniIQzppUvA2EXmdFx_medium.webp","\/storage\/images\/resized\/lJDx0bDyacHZ3yQ2jbu9irCg0U4Vk7lSKsv3zxgr_medium.webp","\/storage\/images\/resized\/dKZBswy2nhqQGDOoOUCdbRPFUc3PhBe22beB45Zm_medium.webp","\/storage\/images\/resized\/F2NVMXW4IPBpOueWASh8U0fGJOKv0OhqB07ou41y_medium.webp","\/storage\/images\/resized\/EjTepN4kQIJYDquIUDbpWJfYiMBhsgMzarmvZKZl_medium.webp","\/storage\/images\/resized\/60UStnu57GUgll5jUkTVA2bxGRRg137okz8zSTg4_medium.webp","\/storage\/images\/resized\/NzhrCa2w7pJQzsCmgIwtZhWp4A9ZORO948UQn1fs_medium.webp","\/storage\/images\/resized\/vESpJswoEKmwJViYTWjYRGq0xXvfC7ciA2I9FmVt_medium.webp","\/storage\/images\/resized\/nJOyvuZSRGQ34sf5eyhxCVIw20k4iANyoiB7ioVs_medium.webp","\/storage\/images\/resized\/FEszLEqUL91kz1kgcrjRRbfPzbiYgKkMLiMmNSrs_medium.webp","\/storage\/images\/resized\/VTt7oUh54NOig9cDAnITBNj1g8FW5jDsi2XWu83e_medium.webp","\/storage\/images\/resized\/YAra9EhmCjWo9g1v9XhF3SoD8VoMAwDhr4GkUefp_medium.webp","\/storage\/images\/resized\/lcgW9fBKm1j95zFbPV0WOFJCRr8vQ3LOhzQapd3O_medium.webp","\/storage\/images\/resized\/fhOenyx1lXuX9jKhaY1DDPIpDd336b6Vn9rBoavM_medium.webp","\/storage\/images\/resized\/Ccw7gq73OYvxX9UUK12ZISkBTXhsScOI9fjKjLXW_medium.webp","\/storage\/images\/resized\/3EK9xVAuufiiSo3DJo3Ts3t1uhLnt9ZqPWb6nN4m_medium.webp","\/storage\/images\/resized\/xPz9bdn99qbO0L4NlGDKDb8jxqGpRGxv4hcHVyts_medium.webp","\/storage\/images\/resized\/hNEOheoCndpqnd1oewa2Z7JRd1XO5KxNCW3absAn_medium.webp","\/storage\/images\/resized\/4yDtWKIEFTVGhJOThWb977epaUjHut4mabibT36h_medium.webp","\/storage\/images\/resized\/HdjQ63rYr5HJLMHtdN4O2SaC7Rm9alX3P5n0n2yv_medium.webp","\/storage\/images\/resized\/B3JG2bUPCazfzNsdMA8UZpd4aYJdCsLTTaRlIbtg_medium.webp","\/storage\/images\/resized\/XMFHNgoRSIEzQne6v4YH4y1Z5FnuhDVnOfxkywmc_medium.webp","\/storage\/images\/resized\/6Hanu3MtT3LommcCD6N6X2WzvS8Y4D17Y6sqw2Xk_medium.webp","\/storage\/images\/resized\/PBq9F4J7XM2VrhSAHLVcFyMLED6wg5mThM6eT1tu_medium.webp","\/storage\/images\/resized\/VxFfiRY0AJdI9iYfprOinardwDs0PiEtFb7MXj4B_medium.webp"],"datasets":[{"label":"","data":[41,29,29,21,21,21,20,20,17,17,16,15,15,14,14,13,13,12,12,12,11,11,10,10,10,9,9,9,9,8],"backgroundColor":["#3B82F6","#3B82F6","#3B82F6","#3B82F6","#3B82F6","#3B82F6","#3B82F6","#3B82F6","#3B82F6","#3B82F6","#3B82F6","#3B82F6","#3B82F6","#3B82F6","#3B82F6","#3B82F6","#3B82F6","#3B82F6","#3B82F6","#3B82F6","#3B82F6","#3B82F6","#3B82F6","#3B82F6","#3B82F6","#3B82F6","#3B82F6","#3B82F6","#3B82F6","#3B82F6"],"percentage":[6.38,4.51,4.51,3.27,3.27,3.27,3.11,3.11,2.64,2.64,2.49,2.33,2.33,2.18,2.18,2.02,2.02,1.87,1.87,1.87,1.71,1.71,1.56,1.56,1.56,1.4,1.4,1.4,1.4,1.24],"barThickness":13}],"rorIds":{"3356":"02664zk40","2424":"03eyq4y97","2454":"04qr3zq92","2434":"00yhnba62","2966":"0064kty71","2989":"05s92vm98","2795":"04w9fbh59","3447":"05x2bcf33","1323":"05hffr360","1679":"00wdq3744","3106":"02e7b5302","3326":"04azbjn80","4416":"03q8dnn23","2453":"01y0j0j86","3408":"02423gm04","1260":"02f81g417","1362":"001p3jz28","1339":"03snqfa66","2252":"01yqg2h08","3741":"00vtgdb53","10":"00v0z9322","2801":"05gbn2817","1352":"01j1rma10","2236":"00a2xv884","3012":"02n96ep67","2671":"05ynxx418","3065":"01vy4gh70","3249":"04zfme737","4467":"02jqj7156","1360":"001kv2y39"}},"options":{"indexAxis":"y","maintainAspectRatio":false,"scales":{"y":{"ticks":{"precision":0,"autoSkip":false,"font":{"family":"Montserrat"},"color":"#000000"},"stacked":false},"x":{"ticks":{"stepSize":null,"precision":0,"font":{"family":"Montserrat"},"color":"#000000"},"stacked":false}},"plugins":{"legend":{"position":"top","labels":{"font":{"family":"Montserrat"},"color":"#000000"}},"title":{"display":true,"text":"With other organizations","font":{"size":24,"family":"Montserrat","weight":600},"color":"#000000"}}}},"foreignOrganizations":{"type":"bar","data":{"show":true,"labels":["Hamad Bin Khalifa University","University of Electronic Science and Technology of China","Qatar University","Sun Yat-sen University","Xidian University","Beijing University of Posts and Telecommunications","Carnegie Mellon University","Thapar Institute of Engineering and Technology","Nanyang Technological University","Guangdong University of Technology","City University of Hong Kong","Northwestern Polytechnical University","Shenyang Aerospace University","King Saud University","Birla Institute of Technology and Science, Pilani","Harbin Institute of Technology","University of Glasgow","Moscow Institute of Physics and Technology","China University of Petroleum (East China)","Zhejiang University","East China Normal University","Link\u00f6ping University","Shenzhen University","Liverpool John Moores University","George Mason University","University of Kufa","Beihang University","Nanjing University of Aeronautics and Astronautics","Nanjing University of Information Science and Technology","Nankai University"],"ids":[2424,2454,2434,2966,2989,2795,3447,1679,3106,3326,4416,2453,3408,1260,1362,2252,3741,10,2801,2236,3012,2671,3065,3249,4467,1861,2328,2739,2743,2895],"codes":[0,0,0,0,0,0,0,0,0,0,0,0,0,0,0,0,0,0,0,0,0,0,0,0,0,0,0,0,0,0],"imageUrls":["\/storage\/images\/resized\/nFNLC5V7pQW5kMG0bS5yD4RWBuZ0oxoPvyKHj6vL_medium.webp","\/storage\/images\/resized\/LcCanHnZPh0mBIzCPZ79rcwQuiKwpNh24pD6TOtd_medium.webp","\/storage\/images\/resized\/Vs9svwplpO16ogA4Qa1NBM55QdLHni2FJBdn63Fj_medium.webp","\/storage\/images\/resized\/YnpZacG3TRTWCTE9ip17nwyzaWfGybATOPIThnax_medium.webp","\/storage\/images\/resized\/QygBwxzSppJCzVvOK3Fg19SniIQzppUvA2EXmdFx_medium.webp","\/storage\/images\/resized\/lJDx0bDyacHZ3yQ2jbu9irCg0U4Vk7lSKsv3zxgr_medium.webp","\/storage\/images\/resized\/dKZBswy2nhqQGDOoOUCdbRPFUc3PhBe22beB45Zm_medium.webp","\/storage\/images\/resized\/EjTepN4kQIJYDquIUDbpWJfYiMBhsgMzarmvZKZl_medium.webp","\/storage\/images\/resized\/60UStnu57GUgll5jUkTVA2bxGRRg137okz8zSTg4_medium.webp","\/storage\/images\/resized\/NzhrCa2w7pJQzsCmgIwtZhWp4A9ZORO948UQn1fs_medium.webp","\/storage\/images\/resized\/vESpJswoEKmwJViYTWjYRGq0xXvfC7ciA2I9FmVt_medium.webp","\/storage\/images\/resized\/nJOyvuZSRGQ34sf5eyhxCVIw20k4iANyoiB7ioVs_medium.webp","\/storage\/images\/resized\/FEszLEqUL91kz1kgcrjRRbfPzbiYgKkMLiMmNSrs_medium.webp","\/storage\/images\/resized\/VTt7oUh54NOig9cDAnITBNj1g8FW5jDsi2XWu83e_medium.webp","\/storage\/images\/resized\/YAra9EhmCjWo9g1v9XhF3SoD8VoMAwDhr4GkUefp_medium.webp","\/storage\/images\/resized\/fhOenyx1lXuX9jKhaY1DDPIpDd336b6Vn9rBoavM_medium.webp","\/storage\/images\/resized\/Ccw7gq73OYvxX9UUK12ZISkBTXhsScOI9fjKjLXW_medium.webp","\/storage\/images\/resized\/3EK9xVAuufiiSo3DJo3Ts3t1uhLnt9ZqPWb6nN4m_medium.webp","\/storage\/images\/resized\/xPz9bdn99qbO0L4NlGDKDb8jxqGpRGxv4hcHVyts_medium.webp","\/storage\/images\/resized\/4yDtWKIEFTVGhJOThWb977epaUjHut4mabibT36h_medium.webp","\/storage\/images\/resized\/HdjQ63rYr5HJLMHtdN4O2SaC7Rm9alX3P5n0n2yv_medium.webp","\/storage\/images\/resized\/B3JG2bUPCazfzNsdMA8UZpd4aYJdCsLTTaRlIbtg_medium.webp","\/storage\/images\/resized\/XMFHNgoRSIEzQne6v4YH4y1Z5FnuhDVnOfxkywmc_medium.webp","\/storage\/images\/resized\/6Hanu3MtT3LommcCD6N6X2WzvS8Y4D17Y6sqw2Xk_medium.webp","\/storage\/images\/resized\/PBq9F4J7XM2VrhSAHLVcFyMLED6wg5mThM6eT1tu_medium.webp","\/storage\/images\/resized\/Zfp3eJods37jcplAEQgRXu7X71KXMez5N6lzfUXW_medium.webp","\/storage\/images\/resized\/CqMlJIjxp6Lm1cCVsVX8XFLZ9j1q2aZFnAnfP2jG_medium.webp","\/storage\/images\/resized\/TogL5D8OPWCYShhXyerf1z1Cq2f0flJFxuJmlFY2_medium.webp","\/storage\/images\/resized\/4eIJF7wlLKPiZ5eZR4kGVbhnDJ4LFeD1BimqMI90_medium.webp","\/storage\/images\/resized\/9ZbCfrCzBxSmmPzCFlCRNPtiPIze3RnT41Nvm4BE_medium.webp"],"datasets":[{"label":"","data":[29,29,21,21,21,20,20,17,16,15,15,14,14,13,13,12,12,11,11,10,10,9,9,9,9,8,8,8,8,8],"backgroundColor":["#3B82F6","#3B82F6","#3B82F6","#3B82F6","#3B82F6","#3B82F6","#3B82F6","#3B82F6","#3B82F6","#3B82F6","#3B82F6","#3B82F6","#3B82F6","#3B82F6","#3B82F6","#3B82F6","#3B82F6","#3B82F6","#3B82F6","#3B82F6","#3B82F6","#3B82F6","#3B82F6","#3B82F6","#3B82F6","#3B82F6","#3B82F6","#3B82F6","#3B82F6","#3B82F6"],"percentage":[4.51,4.51,3.27,3.27,3.27,3.11,3.11,2.64,2.49,2.33,2.33,2.18,2.18,2.02,2.02,1.87,1.87,1.71,1.71,1.56,1.56,1.4,1.4,1.4,1.4,1.24,1.24,1.24,1.24,1.24],"barThickness":13}],"rorIds":{"2424":"03eyq4y97","2454":"04qr3zq92","2434":"00yhnba62","2966":"0064kty71","2989":"05s92vm98","2795":"04w9fbh59","3447":"05x2bcf33","1679":"00wdq3744","3106":"02e7b5302","3326":"04azbjn80","4416":"03q8dnn23","2453":"01y0j0j86","3408":"02423gm04","1260":"02f81g417","1362":"001p3jz28","2252":"01yqg2h08","3741":"00vtgdb53","10":"00v0z9322","2801":"05gbn2817","2236":"00a2xv884","3012":"02n96ep67","2671":"05ynxx418","3065":"01vy4gh70","3249":"04zfme737","4467":"02jqj7156","1861":"02dwrdh81","2328":"00wk2mp56","2739":"01scyh794","2743":"02y0rxk19","2895":"01y1kjr75"}},"options":{"indexAxis":"y","maintainAspectRatio":false,"scales":{"y":{"ticks":{"precision":0,"autoSkip":false,"font":{"family":"Montserrat"},"color":"#000000"},"stacked":false},"x":{"ticks":{"stepSize":null,"precision":0,"font":{"family":"Montserrat"},"color":"#000000"},"stacked":false}},"plugins":{"legend":{"position":"top","labels":{"font":{"family":"Montserrat"},"color":"#000000"}},"title":{"display":true,"text":"With foreign organizations","font":{"size":24,"family":"Montserrat","weight":600},"color":"#000000"}}}}}