Tecnalia Research & Innovation
Are you a researcher?
Create a profile to get free access to personal recommendations for colleagues and new articles.
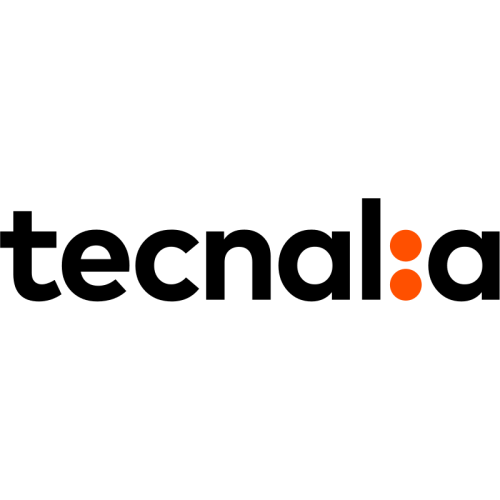
Publications
3 514
Citations
103 613
h-index
129
Top-3 journals
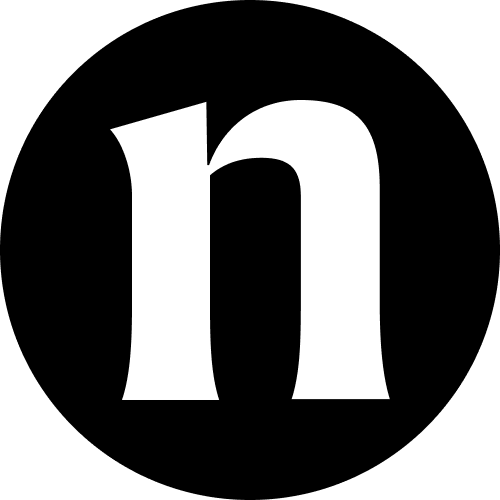
Lecture Notes in Computer Science
(114 publications)
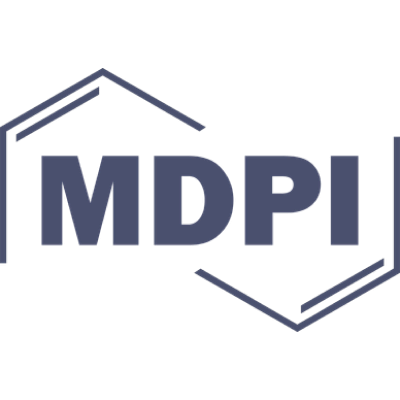
Sensors
(48 publications)
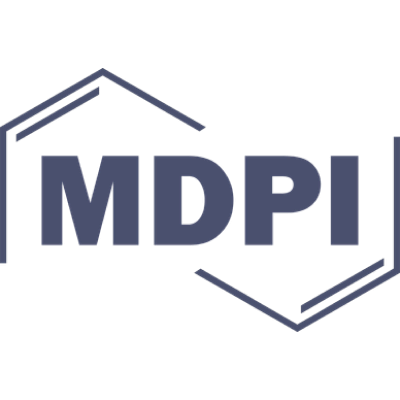
Energies
(44 publications)
Top-3 organizations
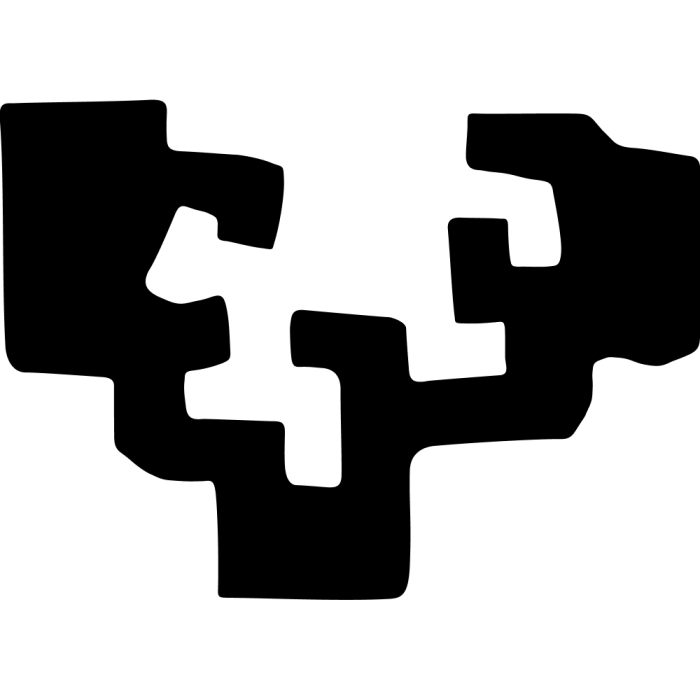
University of the Basque Country
(1041 publications)
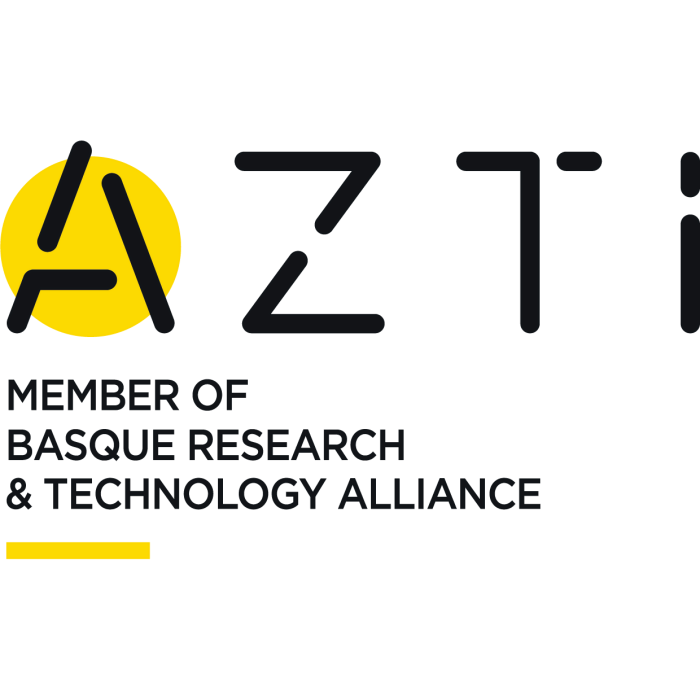
AZTI (Ciencia y tecnología marina y alimentaria)
(605 publications)
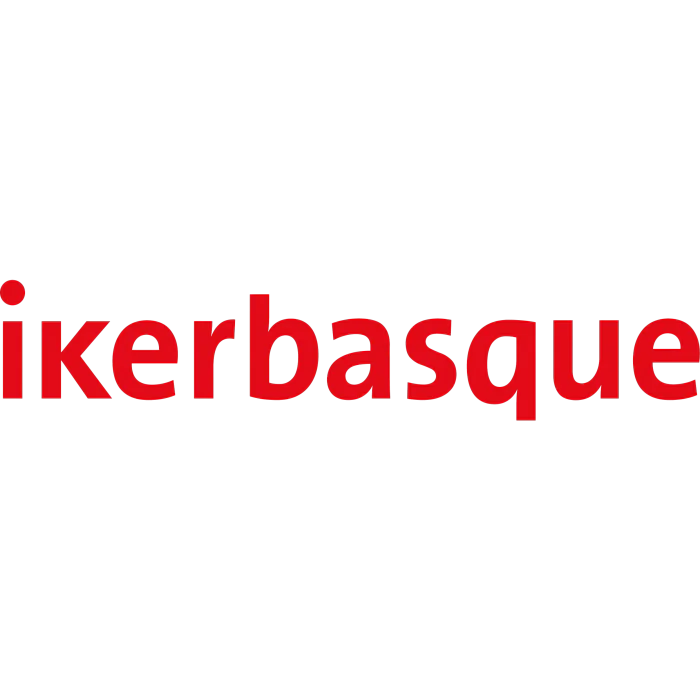
Basque Foundation for Science
(147 publications)
Top-3 foreign organizations

Eindhoven University of Technology
(50 publications)
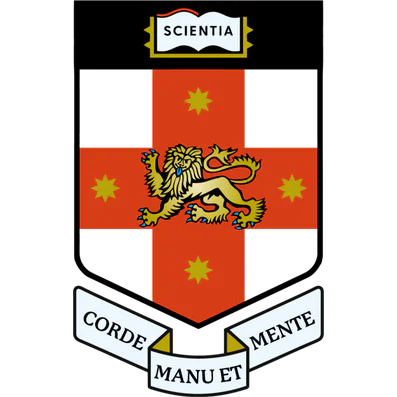
University of New South Wales
(46 publications)
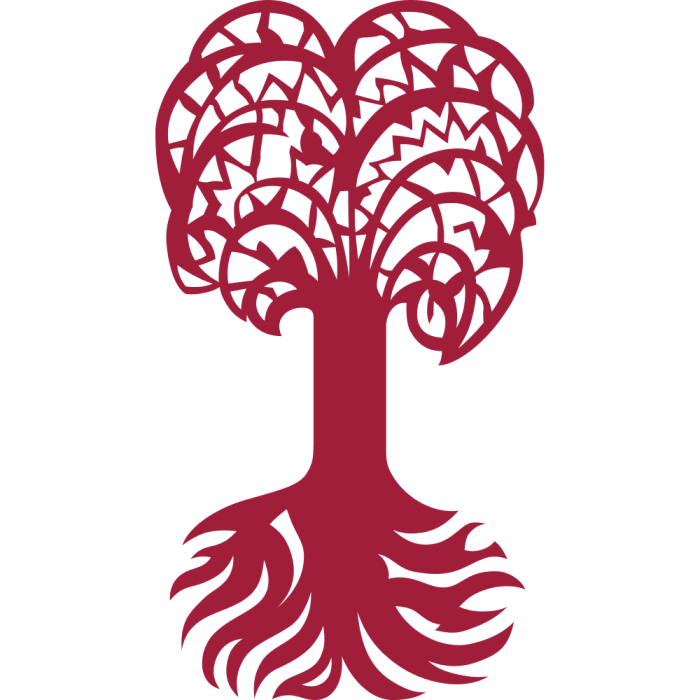
University of Tübingen
(41 publications)
Most cited in 5 years
Nothing found, try to update filter.
Nothing found, try to update filter.
Bidarte I., Galache J.M., Mellado I.
Herranz-Pascual K., Anchustegui P., Cantergiani C., Iraurgi I.
Lorenzo L., Pitacco W., Mattar N., Faye I., Maestro B., Ortiz P.
Xiong M., Chen H., Karaca Y., Jilani Saudagar A.K., Lee I.H., Del Ser J., Muhammad K.


















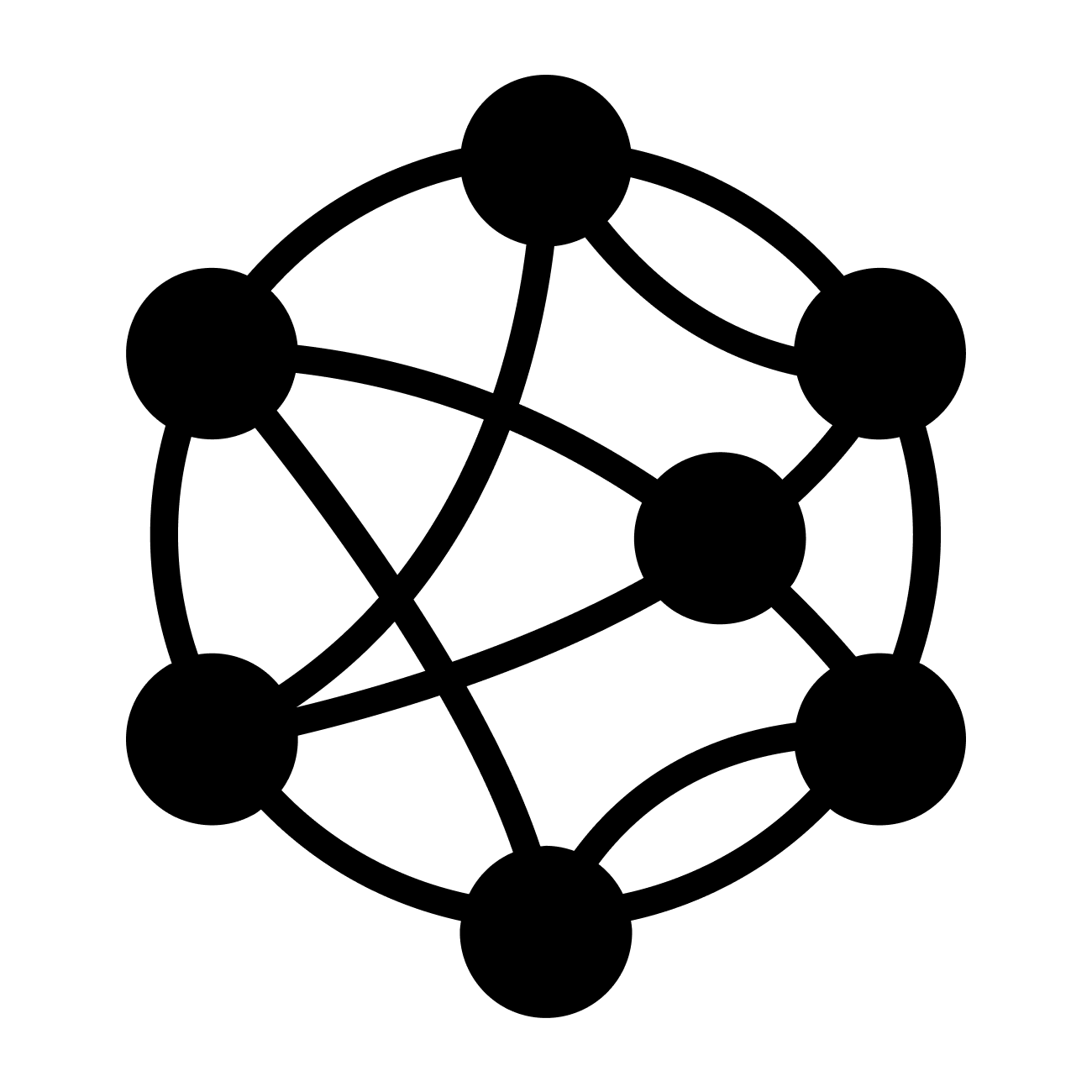