Macao Polytechnic University
Are you a researcher?
Create a profile to get free access to personal recommendations for colleagues and new articles.
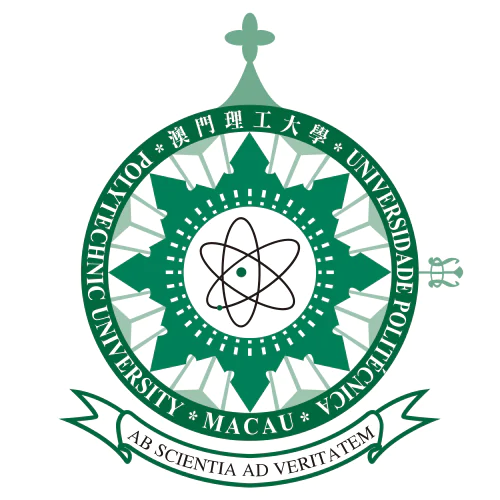
Publications
1 535
Citations
15 588
h-index
56
Top-3 journals
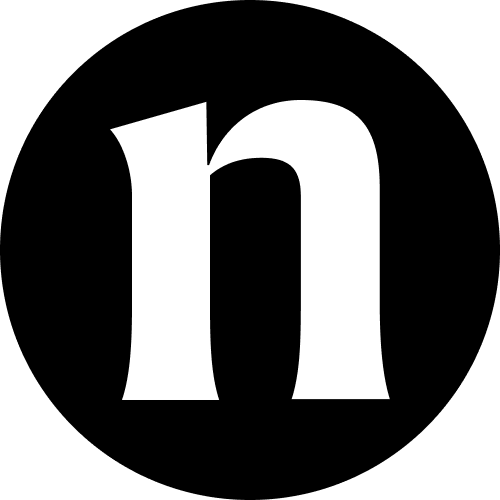
Lecture Notes in Computer Science
(73 publications)
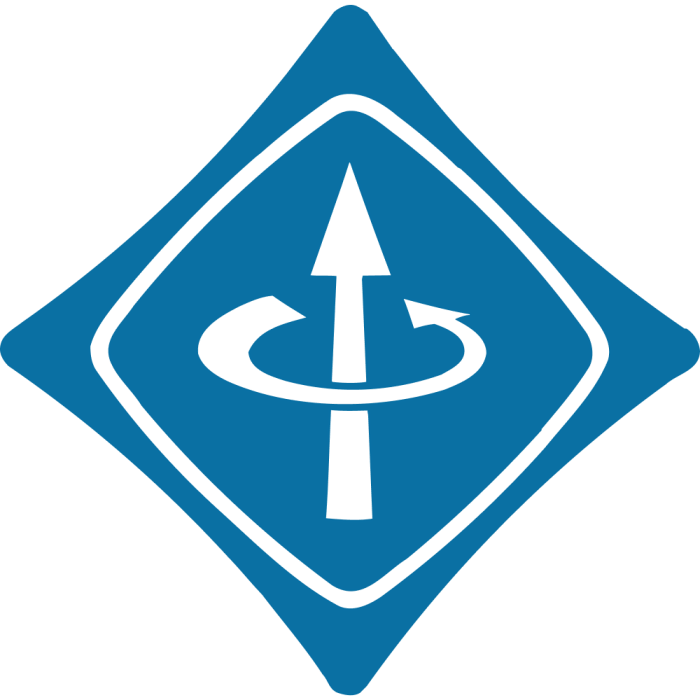
IEEE Access
(35 publications)
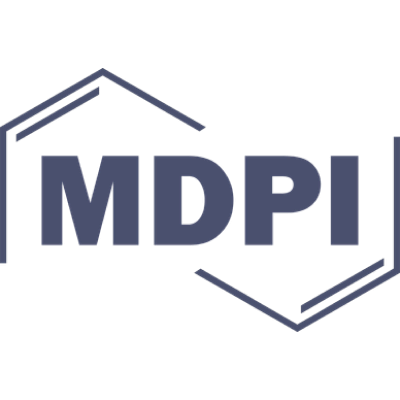
Sustainability
(28 publications)
Top-3 organizations
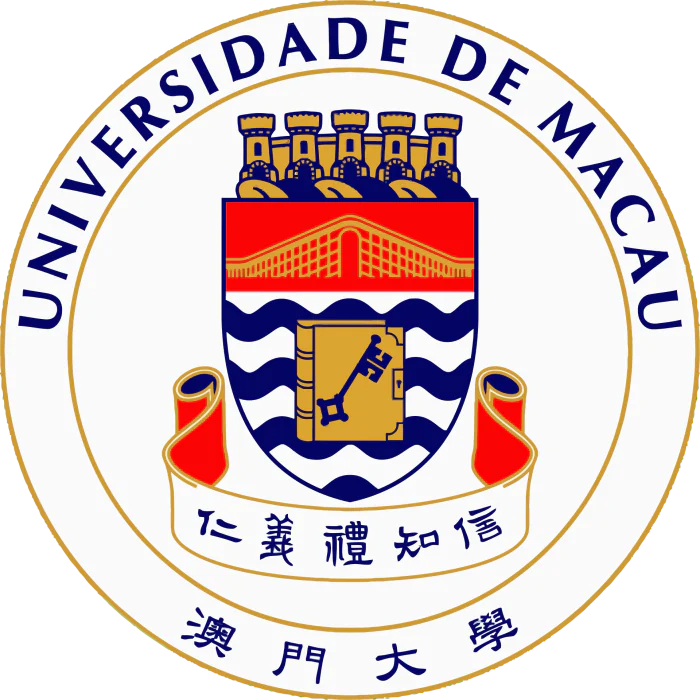
University of Macau
(143 publications)
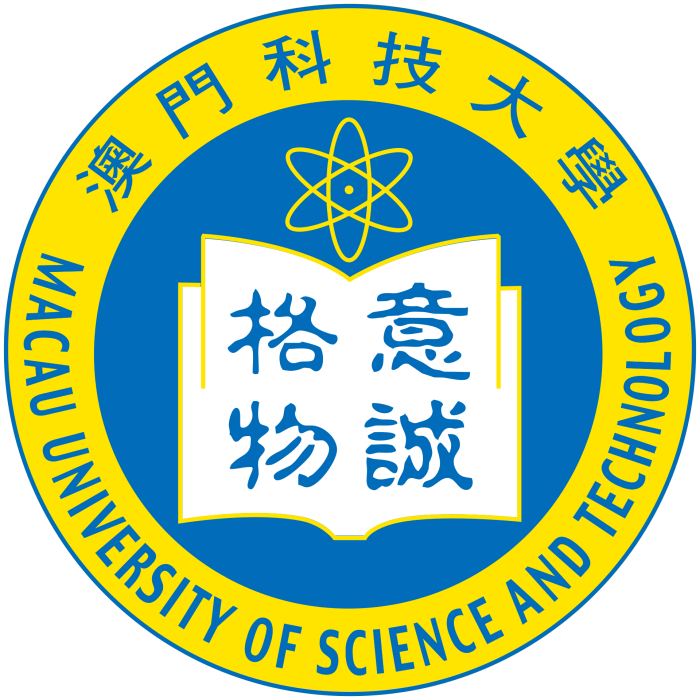
Macau University of Science and Technology
(83 publications)
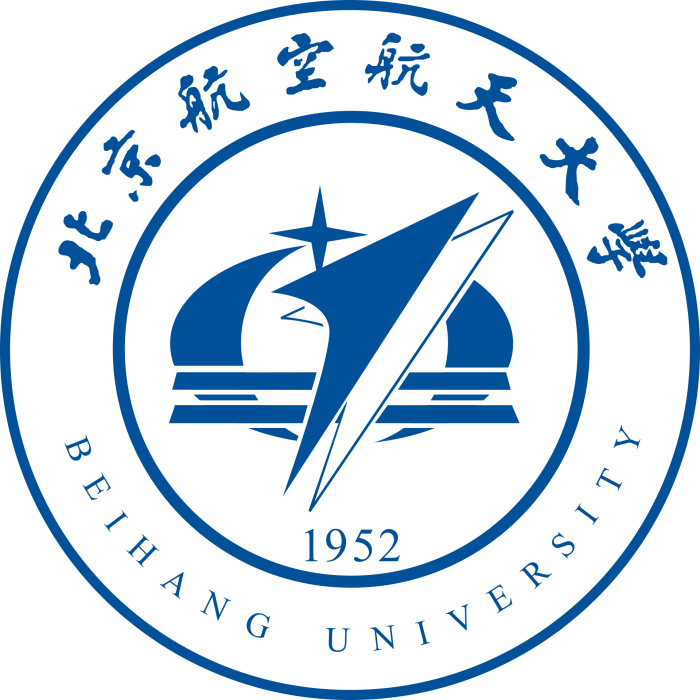
Beihang University
(62 publications)
Top-3 foreign organizations
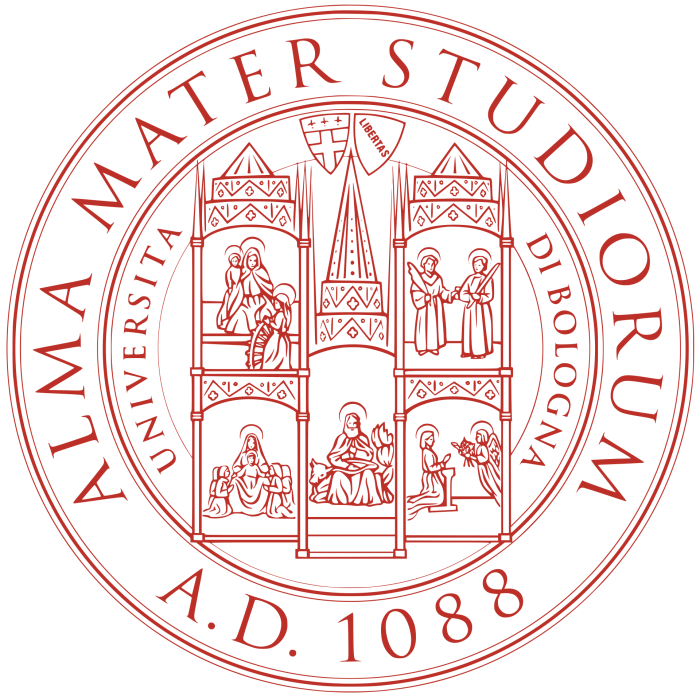
University of Bologna
(29 publications)
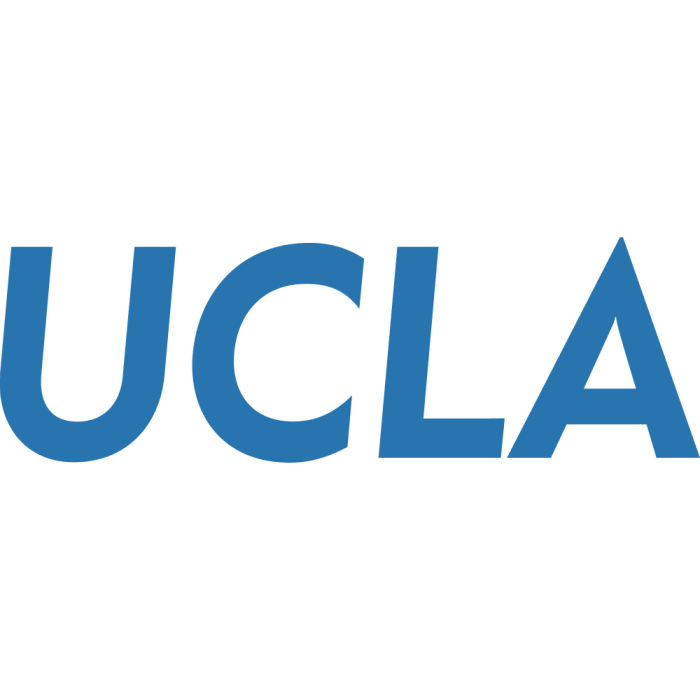
University of California, Los Angeles
(18 publications)
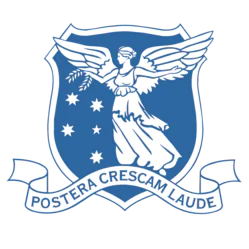
University of Melbourne
(17 publications)
Most cited in 5 years
Nothing found, try to update filter.
Nothing found, try to update filter.
Guo J., Chong C.F., Liang X., Mao C., Li J., Lam C., Shen J., Ng B.K.


















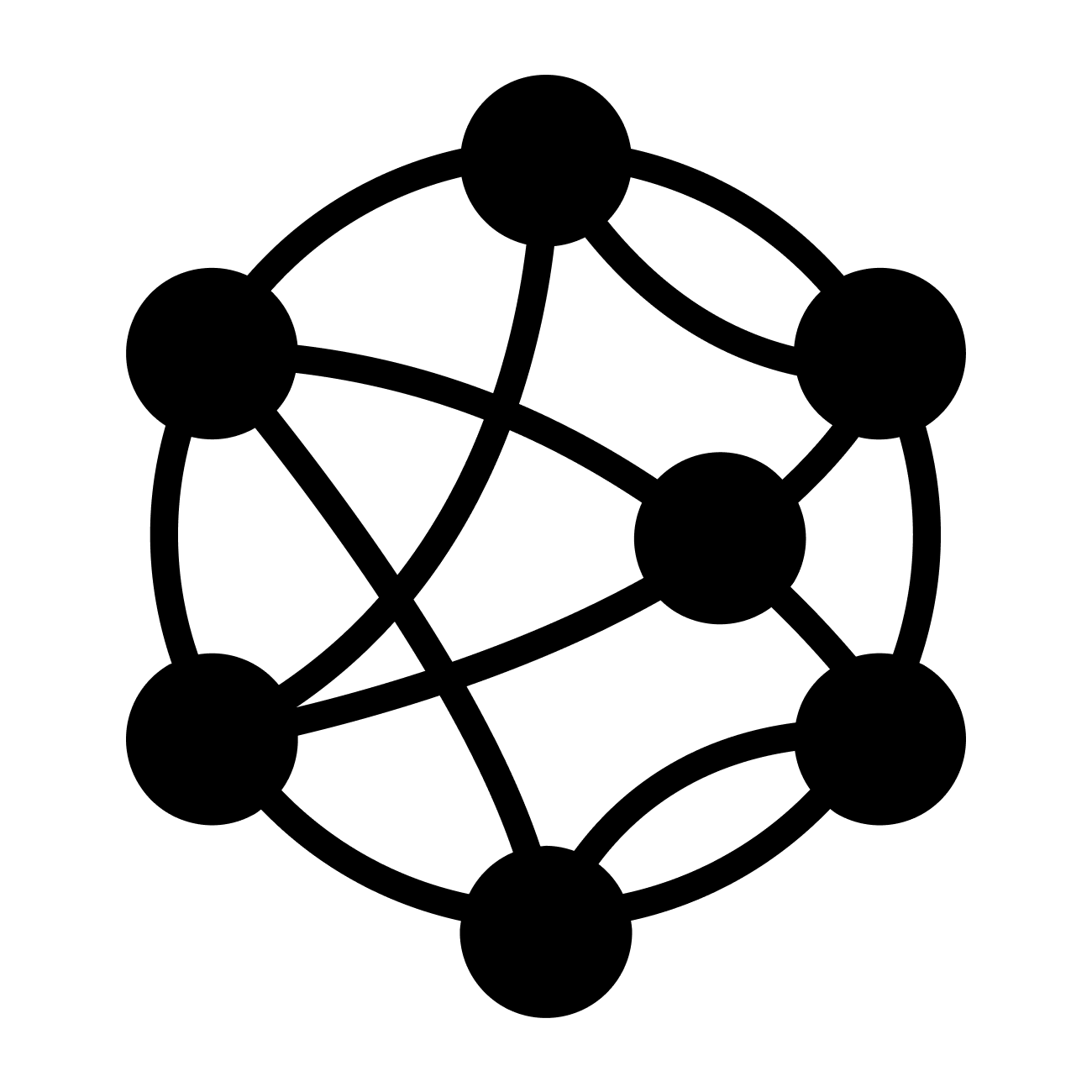