Cannarile, Francesco
Publications
18
Citations
176
h-index
5
- Aerospace Science and Technology (1)
- Annals of Nuclear Energy (1)
- Engineering Applications of Artificial Intelligence (1)
- Fuzzy Sets and Systems (1)
- International Journal of Prognostics and Health Management (1)
- Lecture Notes in Computer Science (1)
- Machines (1)
- Proceedings of the Institution of Mechanical Engineers, Part O: Journal of Risk and Reliability (2)
Nothing found, try to update filter.
Suardi M., Cannarile F., Guastone G., Fidanzi A., Millini R., Testa D.
Abstract
The energy sector plays a pivotal role in the journey towards achieving net-zero emissions. As one of the largest contributors to greenhouse gas emissions, transforming the energy sector is essential for the climate change goals. The transition involves a shift from fossil fuel-based energy sources to renewable and low-carbon alternatives. Embracing renewable energy technologies such as solar, wind, hydro, and geothermal power is vital for reducing carbon emissions. Carbon capture, utilization, and storage (CCUS) technologies also play a crucial role in mitigating emissions from fossil fuel-based power plants and industrial processes. Collaborative efforts, innovative solutions, and supportive policies are needed to drive this transformation and ensure a sustainable, net-zero emissions future for the energy sector. Artificial Intelligence (AI) is currently playing a primary role in accelerating and de-risking the development of novel technologies for achieving these goals.
Artificial intelligence can be strategic to accelerate energy industry towards the achievement of net zero emissions with a transversal and integrated approach starting from the introduction of new digital solutions up to their industrialization [1]. In this context, we propose a framework which aims to develop AI solutions that align with the level of awareness and data availability on the basis of the maturity of the development of novel R&D technology. These levels are commonly classified as experimental, prototypal, and demonstrative according to the typically used Technology Readiness Level (TRL) taxonomy [2]. The experimental phase focuses on conducting experiments and gathering data to explore the feasibility and potential of a new technology. The emphasis is on the proof of concept, testing hypotheses, and assessing the fundamental principles underlying the technology. Once the experimental phase has shown to be successful, the technology progresses to the prototypal phase. Here, a functional prototype is developed to validate the design and functionality of the technology. The prototype serves as a tangible representation of the envisioned solution and allows for further testing, refinement, and optimization. Finally, the demonstration phase is characterized by the construction and commissioning of a demonstration plant or system that showcases the technology capabilities on a larger scale. This phase aims to provide evidence of the technology performance, its reliability and potentiality for its commercialization. It involves demonstrating the technology functionality, efficiency, and suitability for real-world applications.
In this context, this research work proposes a framework to develop data science and AI solutions complying for the TRL of maturity of an R&D technology The proposed approach has been integrated as an established framework accompanying the full lifecycle of the development of novel R&D technologies for achieving the net zero emissions target. The application of this framework has shown to lead to important benefits in R&D initiatives in terms of data enrichment, speed-up, efficiency, and de-risking; the most tangible results were achieved on projects related to the development of new renewable energies, biofuel production and CO2 capture and utilization.
Corneo A., Suardi M., Lancia L., Cannarile F., Fidanzi A.
Abstract
Objectives/Scope
To ensure that predictive models based on Machine Learning (ML) techniques can be fully exploited by energy plant operators, these should be trained on "actionable" features, i.e., a set of features that are explainable and on which operators can directly act to take mitigative and/or preventive actions. To this aim, we have developed a business-oriented feature selection approach which identifies actionable features while optimizing the model predictive capability at the same time.
Methods, Procedures, Process
We have developed a solution which frames the feature selection step as a Multi-Objective Optimization (MOO) problem which relies on genetic algorithms as search engine. The MOO returns a set of optimal feature subsets referred to as Pareto optimal front on which the best compromise solution in terms of model performance and ‘actionability’ must be chosen. For selecting an optimal solution from the Pareto optimal front, the TOPSIS method has been embraced which automatically ranks the candidate solutions based on their relative importance that the users assign to the two objectives to be optimized.
Results, Observations, Conclusions
The proposed methodology has been successfully applied to a real case study concerning cracking furnaces of a petrochemical plant. The optimized predictive model resulting from the application of the developed feature selection strategy has shown to be properly suited for plant operators allowing them to easily derive insights and properly take actions based on the model output. Further, due to the agnostic nature of the proposed methodology, this can be easily extended and applied to other Energy case studies where "actionability" is a driving key for the successful adoption of digital tools based on ML models.
Novel/Additive Information
To the best of Authors knowledge, the proposed approach which embeds the "actionability" property of a feature as criterium in the feature selection step and automates the process of selecting an optimal solution through the TOPSIS method, is novel from a methodological point of view in the field of machine learning applied to energy transition use cases.
Baraldi P., Compare M., Zio E., Cannarile F., Yang Z.
Proceedings of the Institution of Mechanical Engineers, Part O: Journal of Risk and Reliability
scimago Q2
wos Q3
,
,
2022-07-06,
citations by CoLab: 1
,
PDF,
Abstract
Abstract
Cites
A recurrent difficulty for the effective application of Prognostics and Health Management (PHM) methods is related to the “evolving environments” in which industrial components typically operate. Several factors render the operational environments evolving, including deterioration of components, effects of maintenance activities and changes in working conditions. The issue of evolving environments is even more complicated for multi-component systems, where the degradation of one component can affect the degradation processes of other components, thus modifying their lifetime distributions and the statistical properties of the monitored signals. In an effort to convey research toward practical PHM solutions capable of dealing with evolving environments, the “Aramis challenge on degradation state assessment in evolving environments,” has been launched for the ESREL2020-PSAM15 conference. This work describes the Aramis Data Challenge and associated public dataset, illustrates the methods proposed for its solution and the related results obtained. For the evaluation of the goodness of the fault detection methods, an original metric is introduced, which is a variant of the timeliness metric that has been used in the PHM08 data challenge.
Cannarile F., Montoli S., Giliberto G., Suardi M., Di Bari B., Formato G., Farina D., Magni G., Mutidieri L., Prospero A., Fidanzi A., Dal Forno L., Ricci Maccarini G.
Abstract
Lost circulation is a challenging aspect during drilling operations as uncontrolled flow of wellbore fluids into formation can expose rig personnel and environment to risks. Further, the time required to regain the circulation of drilling fluid typically results in unplanned Non-Productive Time (NPT) causing undesired amplified drilling cost. Thus, it is of primary importance to support drilling supervisors with accurate and effective detection tools for safe and economic drilling operations.
In this framework, a novel lost circulation intelligent detection system is proposed which relies on the simultaneous identification of decreasing trends in the paddle mud flow-out and standpipe pressure signals, at constant mud flow-in rate. First, mud flow-out and standpipe pressure signals underlie cubic-spline-based smoothing step to remove background noise caused by the measurement instrument and the intrinsic variability of the drilling environment. To identify structural changes in the considered signals, a nonparametric kernel-based change point detection algorithm is employed. Finally, an alarm is raised if flow-out and standpipe pressure decreasing trends have been detected and their negative variations are below prefixed threshold values.
The proposed intelligent lost circulation detection system has been verified with respect to historical field data recorded from several Eni wells located in different countries. Results show that the proposed system satisfactorily and reliably detects both partial and total lost circulation events. Further, its integration with already existing Eni NPT prediction models has led to a significant improvement in terms of events correctly triggered.
Cannarile F., Baraldi P., Colombo P., Zio E.
Sensor data validation has become an important issue in the operation and control of energy production plants. An undetected sensor malfunction may convey inaccurate or misleading information about the actual plant state, possibility leading to unnecessary downtimes and, consequently, large financial losses. The objective of this work is the development of a novel sensor data validation method to promptly detect sensor malfunctions. The proposed method is based on the analysis of data regularity properties, through the joint use of Continuous Wavelet Transform and image analysis techniques. Differently from the typical sensor data validation techniques which detect a sensor malfunction by observing variations in the relationships among measurements provided by different sensors, the proposed method validates the data collected by a given sensor only using historical data collected from the sensor itself. The proposed method is shown able to correctly detect different types and intensities of sensor malfunctions from energy production plants.
Cannarile F., Compare M., Zio E., Duca E., Febi I., Renzetti M., Platini M.
Cannarile F., Compare M., Baraldi P., Yang Z., Zio E.
Cannarile F., Compare M., Baraldi P., Diodati G., Quaranta V., Zio E.
The objective of this work is the development of a fault diagnostic system for a shaker blower used in on-board aeronautical systems. Features extracted from condition monitoring signals and selected by the ELastic NET (ELNET) algorithm, which combines l 1 -penalty with the squared l 2 -penalty on model parameters, are used as inputs of a Multinomial Logistic regression (MLR) model. For validation, the developed approach is applied to experimental data acquired on a shaker blower system (as representative of aeronautical on-board systems) and on three additional experimental datasets of literature. The satisfactory diagnostic performances obtained show the potential of the method for developing sound diagnostic classifiers from a very large set of features, even when only few training data are available.
Cannarile F., Baraldi P., Zio E.
Data-driven methods for direct prognostic map the relationship between monitored parameters and equipment Remaining Useful Life (RUL). They typically require the availability of a set of run-to-failure degradation trajectories for model training. Yet, in many industrial applications, equipment is often replaced before they fail to avoid catastrophic consequences on production and safety. Then also, incomplete degradation trajectories are available. In this work, we develop a method for predicting equipment RUL, and the related uncertainty based on both complete and incomplete degradation trajectories. The method is based on the combined use of a similarity measure and Evidence Theory (EvT). Application of the method on two case studies shows that it provides accurate RUL predictions, also in comparison with a similarity-based regression method of literature.
Cannarile F., Compare M., Baraldi P., Di Maio F., Zio E.
This work presents a method to improve the diagnostic performance of empirical classification system (ECS), which is used to estimate the degradation state of components based on measured signals. The ECS is embedded in a homogenous continuous-time, finite-state semi-Markov model (HCTFSSMM), which adjusts diagnoses based on the past history of components. The combination gives rise to a homogeneous continuous-time finite-state hidden semi-Markov model (HCTFSHSMM). In an application involving the degradation of bearings in automotive machines, the proposed method is shown to be superior in classification performance compared to the single-stage ECS.
Cannarile F., Baraldi P., Compare M., Borghi D., Capelli L., Zio E.
Cannarile F., Compare M., Di Maio F., Zio E.
Proceedings of the Institution of Mechanical Engineers, Part O: Journal of Risk and Reliability
scimago Q2
wos Q3
,
,
2018-03-28,
citations by CoLab: 2
,
PDF,
Abstract
Abstract
Cites
Big data from very large fleets of assets challenge the asset management, as the number of maintenance strategies to optimize and administrate may become very large. To address this issue, we exploit a clustering approach that identifies a small number of sets of assets with similar reliability behaviors. This enables addressing the maintenance strategy optimization issue once for all the assets belonging to the same cluster and, thus, introduces a strong simplification in the asset management. However, the clustering approach may lead to additional maintenance costs, due to the loss of refinement in the cluster reliability model. For this, we propose a cost model to support asset managers in trading off the simplification brought by the cluster-based approach against the related extra costs. The proposed approach is applied to a real case study concerning a set of more than 30,000 switch point machines.
Cannarile F., Compare M., Rossi E., Zio E.
Multi-State (MS) reliability models are used in practice to describe the evolution of degradation in industrial components and systems. To estimate the MS model parameters, we propose a method based on the Fuzzy Expectation-Maximization (FEM) algorithm, which integrates the evidence of the field inspection outcomes with information taken from the maintenance operators about the transition times from one state to another. Possibility distributions are used to describe the imprecision in the expert statements. A procedure for estimating the Remaining Useful Life (RUL) based on the MS model and conditional on such imprecise evidence is, then, developed. The proposed method is applied to a case study concerning the degradation of pipe welds in the coolant system of a Nuclear Power Plant (NPP). The obtained results show that the combination of field data with expert knowledge can allow reducing the uncertainty in degradation estimation and RUL prediction.
Cannarile F., Compare M., Zio E.
We develop a First Principle Model (FPM) simulator of a solenoid micro-valve of the control system of a train braking system. This is used for failure diagnostic when field data of normal and abnormal system behaviors are lacking. A procedure is proposed to adjust the diagnostic model once field data are available.
Cannarile F., Baraldi P., Compare M., Borghi D., Capelli L., Cocconcelli M., Lahrache A., Zio E.
Nothing found, try to update filter.
Morales Matamoros O., Takeo Nava J.G., Moreno Escobar J.J., Ceballos Chávez B.A.
Artificial intelligence (AI) has become a revolutionary tool in the automotive sector, specifically in quality management and issue identification. This article presents a systematic review of AI implementations whose target is to enhance production processes within Industry 4.0 and 5.0. The main methods analyzed are deep learning, artificial neural networks, and principal component analysis, which improve defect detection, process automation, and predictive maintenance. The manuscript emphasizes AI’s role in live auto part tracking, decreasing dependance on manual inspections, and boosting zero-defect manufacturing strategies. The findings indicate that AI quality control tools, like convolutional neural networks for computer vision inspections, considerably strengthen fault identification precision while reducing material scrap. Furthermore, AI allows proactive maintenance by predicting machine defects before they happen. The study points out the importance of incorporating AI solutions in actual manufacturing methods to ensure consistent adaptation to Industry 5.0 requirements. Future investigations should prioritize transparent AI approaches, cyber-physical system consolidation, and AI material enhancement for sustainable production. In general terms, AI is changing quality assurance in the automotive industry, improving efficiency, consistency, and long-term results.
Li S., Liu M., Ma N., He W.
The health of complex systems continues to decline as they operate over long periods of time, so it is important to assess the health state of complex systems. Belief rule base (BRB) is widely used in the field of health state assessment of complex systems as a semi-quantitative method that can address uncertainty effectively and with interpretability. In practical engineering, BRB still has problems: the incompleteness of expert knowledge and the inconsistency of the cognitive abilities of each expert have an effect on the construction of the model and interpretability. To address this problem, a complex system health state assessment method is proposed based on a joint multiexpert belief rule base (BRB-ME). Experts first build their own models, and a new multiexpert knowledge fusion algorithm is designed for the fusion of different expert models. The ER is used as the inference machine for the model. Next, a multi-population evolution whale optimization algorithm with multiexpert knowledge constraints (C-MEWOA) is used to optimize the BRB-ME model. Finally, the effectiveness of the BRB-ME model in health state assessment is verified through case studies of lithium-ion batteries and flywheels. Comparative studies have shown that the BRB-ME model can fuse multiexpert knowledge and has advantages in terms of the stability and accuracy of assessment results.
Zhang M., Chen H., Heidari A.A., Chen Y., Wu Z., Cai Z., Liu L.
The Harris Hawks optimization (HHO) algorithm, categorized as a powerful meta-heuristic algorithm (MA), has gained extensive usage because it lacks parameter adjustment and shows powerful optimization ability. At the same time, it cannot be ignored within the realm of feature selection. However, HHO is deficient in its capacity to escape local optima, which deserves attention. HHO did not perform enough local search in the exploitation stage, resulting in poor final results. This limitation occurs from time to time when facing the high-dimensional feature selection. To address these concerns, a modification of HHO incorporating the wormhole strategy (WS) is proposed, called WHHO. As an example of local search, WS can mutate around the current and optimal search positions. By ensuring population diversity and enhancing the algorithm's capability to escape from local optima, WS contributes to improving results accuracy. WHHO first conducted qualitative experiments at CEC 2017, including historical trajectory analysis, balance analysis, and diversity analysis. Then, WHHO was compared with seven classical MAs, seven advanced MAs, and five well-known HHO variants. In the three groups of experiments, careful consideration of mean, standard deviation, Friedman test, and Wilcoxon sign-rank test results reveals that WHHO secures the top ranking. Furthermore, the binary variant of the algorithm, BWHHO, combined with the k nearest neighbor classifier, was developed for high-dimensional feature selection. Compared with nine binary MAs, WHHO uses fewer features to predict more accurate results on twelve high-dimensional datasets. The fitness value of WHHO on twelve datasets won the overwhelming first place, surpassing the second place with an average ranking of 2.5833. WHHO's ability to reduce the number of selected features is particularly impressive while maintaining high prediction accuracy. In the face of thousands of features, WHHO consistently selects fewer features, with retention rates below 20% on all datasets. This highlights WHHO's optimized feature selection ability and its effectiveness in simplifying the prediction process. Therefore, WHHO is an effective improved optimizer, especially for dealing with high-dimensional feature selection, which has effective value.
Wang J., Liu P., Gao J., Liu T., Wang X.
Abstract
Existing deep learning-based models for mechanical fault diagnosis perform well in identifying predefined faults, but these models substantially degrade in performance when they encounter unknown faults. Thus, it is crucial to investigate open-set fault diagnosis that can handle unknown faults more efficiently. Current methods for open-set fault diagnosis in machinery face challenges by the lack of hierarchical structure in feature representation and the overlapping regions of known and unknown sample distributions. To solve these problems, we propose a composite dual-branching dynamic triplet multivariate constrained (CDDTMC) model for mechanical open-set fault diagnosis. The CDDTMC framework consists of three main core modules: a feature extraction module, a structural constraint module and a fault diagnosis module. In the feature extraction module a composite two-branch network is designed to extract hierarchical feature representations from known samples. After extracting the sample features, it represents the samples with structural constraints using multivariate constraints based on bidirectional dynamic triplet loss to achieve discriminativeness and compactness. Determining the optimal decision boundary for each category based on the structural constraints and uses a distance-based diagnostic algorithm to identify fault diagnosis. We conducted experiments on two publicly available bearing datasets to validate the performance of the model. The results show that the model improves the Average Accuracy Classification (ACC) by 10.73% and 13.84%, respectively, compared to other comparative model.
Vieira A.F., Filho M.R., Eguea J.P., Ribeiro M.L.
Neural networks (NNs) have revolutionized various fields, including aeronautics where it is applied in computational fluid dynamics, finite element analysis, load prediction, and structural optimization. Particularly in optimization, neural networks and deep neural networks are extensively employed to enhance the efficiency of genetic algorithms because, with this tool, it is possible to speed up the finite element analysis process, which will also speed up the optimization process. The main objective of this paper is to present how neural networks can help speed up the process of optimizing the geometries and composition of composite structures (dimension, topology, volume fractions, reinforcement architecture, matrix/reinforcement composition, etc.) compared to the traditional optimization methods. This article stands out by showcasing not only studies related to aeronautics but also those in the field of mechanics, emphasizing that the underlying principles are shared and applicable to both domains. The use of NNs as a surrogate model has been demonstrated to be a great tool for the optimization process; some studies have shown that the NNs are accurate in their predictions, with an MSE of 1×10−5 and MAE of 0.007%. It has also been observed that its use helps to reduce optimization time, such as up to a speed 47.5 times faster than a full aeroelastic model.
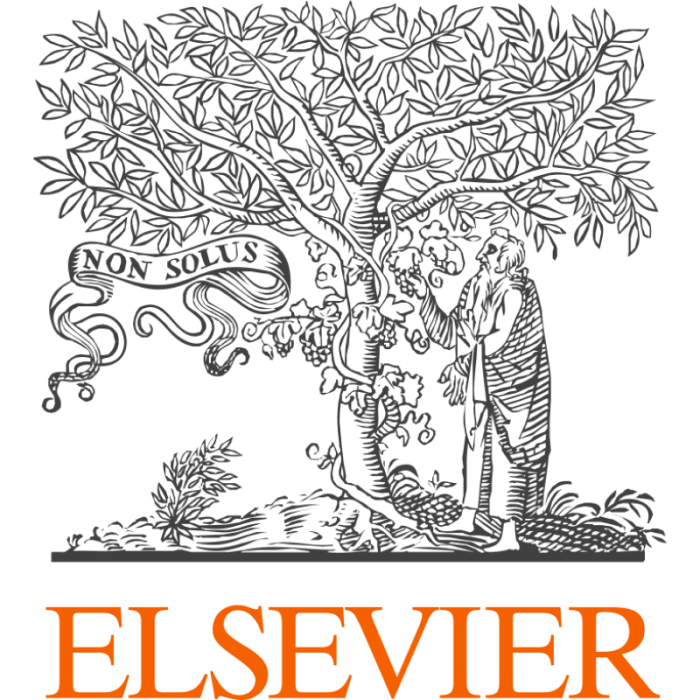
Mondal S., Das S., Vrana V.G.
In this paper, we examine the role of artificial intelligence (AI) in sovereignty and carbon neutrality, emphasizing digital inclusion and climate-resilient AI strategies for emerging markets. Considering the previous studies on AI for carbon neutrality and digital inclusion for climate research along with technology policy frameworks as a guide, this paper undertakes Partial Least Squares Structural Equation Modelling (PLS-SEM) with AI strategies and carbon neutrality outcomes. At the same time, fuzzy-set Qualitative Comparative Analysis (fsQCA) is used to reveal different configurations leading to achieving climate resilience. The model covers various aspects of AI-enabled policy, including technology adoption, policy frameworks, digital literacy, and public engagement. Survey data were collected from key stakeholders in climate policy, technology sectors, and local communities using a structured survey to understand their attitudes towards negative emissions technologies from prominent experts in emerging countries like Vietnam, Italy, Malaysia, and Greece. PLS-SEM results reveal the importance of AI in developing carbon neutrality, a critical AI strategic dimension (Data analytics capability and policy support). Some aspects of the fsQCA findings present heterogeneous outcomes, highlighting complex combinations of digital inclusion, AI adoption, and climate resilience which are industry-specific. This study would further enrich the literature concerning climate strategies by exploring AI, digital inclusion, and carbon neutrality interactions. Theoretically, practical and enriching suggestions for future research are derived to help AI intelligence infuse sustainable climate actions.
Peng C., Sun Y., Su S., guo C.
Abstract
The aero-engine is the heart of an airplane. Predicting the remaining useful lifetime (RUL) of an aero-engine bears great significance, not only for improving the reliability and safety of the aero-engine but also for ensuring aircraft safety and performance. However, both issues, namely the selection of uncorrelated parameters for RUL estimation and the lack of a standard theoretical methodology for Health Index (HI) construction, inevitably impact the prediction accuracy. Here, we proposed an improved similarity-based RUL prediction method considering the degradation degree of multiple condition monitoring parameters for aero-engines. This method includes the improved minimum-redundancy maximum-relevancy approach for the quantitative selection of key parameters, and the similarity matching approach which takes into account the degradation degree of multiple parameters instead of constructing HI. The effectiveness of the proposed method was evaluated on turbine engine datasets. Experimental results show that (1) compared with other feature selection methods, the root mean square error (RMSE) of the proposed method is reduced by 12.3%; (2) compared with other RUL prediction methods, the RMSE of the proposed method is smaller than most methods but without a complicated training process. The results demonstrate that the proposed method achieves highly competitive prediction performance. By employing the proposed method, it is possible to significantly reduce the risk of engine failures, thereby improving safety and economic efficiency.
Gomaa S., Abdalla M., Salem K.G., Nasr K., Emara R., Wang Q., El-hoshoudy A.N.
AbstractThe accurate estimation of gas viscosity remains a pivotal concern for petroleum engineers, exerting substantial influence on the modeling efficacy of natural gas operations. Due to their time-consuming and costly nature, experimental measurements of gas viscosity are challenging. Data-based machine learning (ML) techniques afford a resourceful and less exhausting substitution, aiding research and industry at gas modeling that is incredible to reach in the laboratory. Statistical approaches were used to analyze the experimental data before applying machine learning. Seven machine learning techniques specifically Linear Regression, random forest (RF), decision trees, gradient boosting, K-nearest neighbors, Nu support vector regression (NuSVR), and artificial neural network (ANN) were applied for the prediction of methane (CH4), nitrogen (N2), and natural gas mixture viscosities. More than 4304 datasets from real experimental data utilizing pressure, temperature, and gas density were employed for developing ML models. Furthermore, three novel correlations have developed for the viscosity of CH4, N2, and composite gas using ANN. Results revealed that models and anticipated correlations predicted methane, nitrogen, and natural gas mixture viscosities with high precision. Results designated that the ANN, RF, and gradient Boosting models have performed better with a coefficient of determination (R2) of 0.99 for testing data sets of methane, nitrogen, and natural gas mixture viscosities. However, linear regression and NuSVR have performed poorly with a coefficient of determination (R2) of 0.07 and − 0.01 respectively for testing data sets of nitrogen viscosity. Such machine learning models offer the industry and research a cost-effective and fast tool for accurately approximating the viscosities of methane, nitrogen, and gas mixture under normal and harsh conditions.
Feng T., Guo L., Chen T., Gao H.
In the field of remaining useful life (RUL) prediction, the most prominent task is constructing an accurate prediction model. However, it is difficult for single prediction models to satisfy multiple application situations. Therefore, a new nonlinear ensemble RUL prediction framework based on dynamic-matched weights is proposed in this paper. In the proposed framework, the neural network-based method and the stochastic process-based method are first aggregated through a nonlinear weighting formulation to mitigate data limitations and lack of a priori knowledge. Then, a novel ensemble weight dynamic matching algorithm is designed to achieve time-varying weight matching and improve the prediction accuracy. Finally, the ensemble RUL prediction result is characterized by the probability density function (PDF) of the remaining life. Through two milling cutter experiments, the proposed nonlinear ensemble RUL prediction framework is verified with better comprehensive performance. The cumulative relative accuracy (CRA) of the prediction results is greater than 0.6, which outperforms the commonly used tool RUL prediction method.
Li B., Xue S., Fu Y., Tang Y., Zhao Y.
In order to bridge the gap between the ideal experimental environment and practical engineering applications, many fault diagnosis studies have focused on transfer learning-based methods. However, the research on cross-domain fault diagnosis of aero-engine is obviously insufficient. Besides, most existing studies only consider the impact of global discrepancies between the source and target domains, while overlooking the contributions of individual samples, and the models proposed by these works usually implement the two key steps of distribution discrepancies alignment and fault identification separately, which may lead to the loss of some critical information. To tackle this issue, a novel weighted kernel adaption extreme learning machine is proposed for cross-domain fault diagnosis of aero-engine. Specifically, the proposed approach integrates white cosine similarity (WCS), projected maximum mean discrepancy (PMMD) and kernel extreme learning machine (KELM), which allows the diagnostic model to consider the contributions of individual samples to the alignment of global distribution discrepancies while minimizing the distribution discrepancies and diagnostic error. Finally, the proposed approach is evaluated by extensive tests, including traditional and cross-domain fault diagnosis experiments, and the results of improved diagnostic performance confirm the effectiveness of the proposed approach.
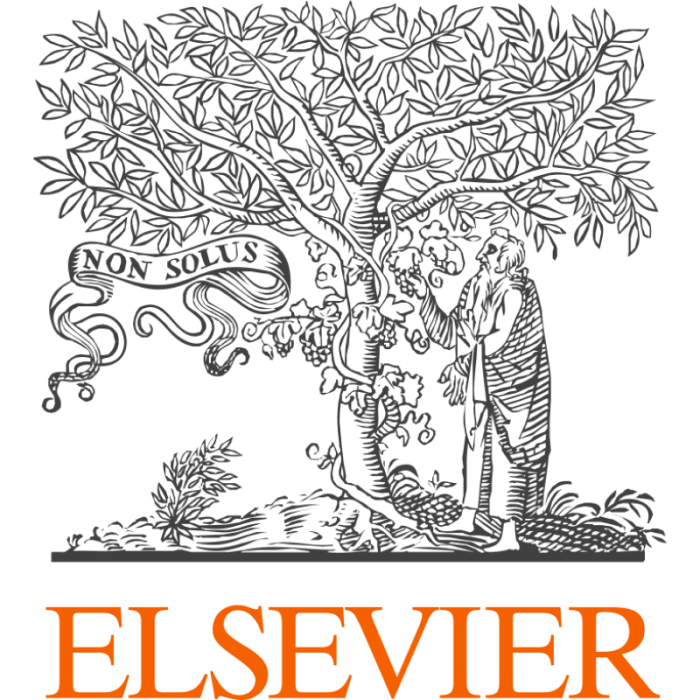
Nothing found, try to update filter.
Cadei L., Rossi G., Lancia L., Loffreno D., Corneo A., Milana D., Montini M., Purlalli E., Fier P., Carducci F., Nizzolo R.
Abstract
This paper reports the development and tests of an advance methodologies to predict Upstream plant risky events, such as flaring, applying an integrated framework. The core idea is to exploit Machine Learning and big data analytics techniques to tackle and manage both major upsets that would lead to significant inefficiency and loss. The tool is developed for complex upstream production system, where upset could be caused by a huge amount of heterogeneous factors, exploiting data driven monitoring systems to identify the weak signals of the upcoming events.
The framework proposed is mainly composed by a strong pipeline divided in 3 modules operating before (predictive phase), during and after the event. The former aims to reduce the probability of an event, the second works on the severity and the third one has a dual function: reporting upsets and feedback gathering system to be used to further improve the analytics implemented. The Predictive component alerts operators when it recognizes a dangerous pattern among the parameters considered. The other two components can support this one and can be exploited to detect early signs of deviations from the proper operating envelope, while predictive performances are not satisfying. Moreover, during an event occurrence, operators can promptly identify the causes of the upset through the entire production system. This allows a faster reaction and consequently a significant reduction in magnitude. The solution proposed provides 2 complementary methodologies:
an agnostic anomaly detection system, helping to map plant functional unit anomalous behavior, as a dynamic operating envelope, and identifying the most affected ones;
A real time root-cause analysis, as a vertical solution, obtained learning from the monitoring of the different specific functional unit;
The tool is also able to provide an automatic event register using information provided by the root-cause system, including operator feedbacks that will improve the performances of each module of the framework.
The entire pipeline developed has been applied on-line, working with real time data coming from an operating oilfield, with special focus on blowdown and flaring system. The robust architecture generated is able to overcome some main issues related to the complexity of Upstream production assets such as lack of data, quick dynamic of physical phenomena analysed and randomness of upsets. The first test demonstrates that the tool accuracy allows to identify and suggest actions on 35% of the most dangerous flaring events occurring. Moreover, the effectiveness increase significantly proving a real time root-cause analysis considering both strong and weak signals that cause dangerous overpressures through the treatment plant.
Zio E.
• Main PHM challenges in industry 4.0: physics, data and solution requirements. • Data challenges: missing of anomalies, labels and the continuously monitored data. • Advancing methods of detection, diagnostics and prognostics for data challenge. • Solution requirements challenge: interpretability, security, uncertainty of models. We are performing the digital transition of industry, living the 4th industrial revolution, building a new World in which the digital, physical and human dimensions are interrelated in complex socio-cyber-physical systems. For the sustainability of these transformations, knowledge, information and data must be integrated within model-based and data-driven approaches of Prognostics and Health Management (PHM) for the assessment and prediction of structures, systems and components (SSCs) evolutions and process behaviors, so as to allow anticipating failures and avoiding accidents, thus, aiming at improved safe and reliable design, operation and maintenance. There is already a plethora of methods available for many potential applications and more are being developed: yet, there are still a number of critical problems which impede full deployment of PHM and its benefits in practice. In this respect, this paper does not aim at providing a survey of existing works for an introduction to PHM nor at providing new tools or methods for its further development; rather, it aims at pointing out main challenges and directions of advancements, for full deployment of condition-based and predictive maintenance in practice.
Hu Y., Miao X., Si Y., Pan E., Zio E.
• The life cycle of prognostics and health management (PHM) is structuredinto DEsign, DEvelopment and DEcision phases (DE3). • Essences, key activities and interconnections of PHM DE3aresummarized. • Gaps, challengesand future researchdirectionsof PHM DE3are proposed. Prognostics and health management (PHM) is an enabling technology used to maintain the reliable, efficient, economic and safe operation of engineering equipment, systems and structures. In the last 5 years, many PHM review works have been published for discussing and summarizing the state-of-the-art of different approaches and methods, usage modes and applications of PHM. This paper intends to enrich the PHM state-of-the-art by reviewing the PHM works from three different points of view: DEsign, DEvelopment and DEcision (DE 3 ). We dig into the problem essence of the DE 3 of PHM by reviewing the research work, extracting the research conclusions from 235 related publications, and exposing the current methodologies and solution frameworks for addressing the DE 3 issues. We identify the gaps and challenges of existing PHM concerning the DE 3 , and point out issues and opportunities of future PHM researches. We expect this review work to provide clear directions for assisting the researchers and practitioners in advancing the PHM methodologies and maturing them into practical PHM technologies.
Gugulothu N., TV V., Malhotra P., Vig L., Agarwal P., Shroff G.
We consider the problem of estimating the remaining useful life (RUL) of a system or a machine from sensor data. Many approaches for RUL estimation based on sensor data make assumptions about how machines degrade. Additionally, sensor data from machines is noisy and often suffers from missing values in many practical settings. We propose Embed-RUL: a novel approach for RUL estimation from sensor data that does not rely on any degradation-trend assumptions, is robust to noise, and handles missing values. Embed-RUL utilizes a sequence-to-sequence model based on Recurrent Neural Networks (RNNs) to generate embeddings for multivariate time series subsequences. The embeddings for normal and degraded machines tend to be different, and are therefore found to be useful for RUL estimation. We show that the embeddings capture the overall pattern in the time series while filtering out the noise, so that the embeddings of two machines with similar operational behavior are close to each other, even when their sensor readings have significant and varying levels of noise content. We perform experiments on publicly available turbofan engine dataset and a proprietary real-world dataset, and demonstrate that Embed-RUL outperforms the previously reported state-of-the-art (Malhotra, TV, et al., 2016) on several metrics.
Truong C., Oudre L., Vayatis N.
This article presents a selective survey of algorithms for the offline detection of multiple change points in multivariate time series. A general yet structuring methodological strategy is adopted to organize this vast body of work. More precisely, detection algorithms considered in this review are characterized by three elements: a cost function, a search method and a constraint on the number of changes. Each of those elements is described, reviewed and discussed separately. Implementations of the main algorithms described in this article are provided within a Python package called ruptures.
Cadei L., Rossi G., Montini M., Fier P., Milana D., Corneo A., Lancia L., Loffreno D., Purlalli E., Carducci F., Nizzolo R., Sophia G.
As Industrial plants evolve towards massive digitalization and their "digital twin" architectures are constantly enriched with a wide range of advanced analytics solutions that are becoming part of the ordinary control operations, the quality of the input data becomes of paramount importance. But as the number of variables, KPIs and processes monitored in plants raise of several orders of magnitude, automated procedures are needed for an effective monitoring.
We have developed a novelty detection framework with the objective of monitoring each and every variable in the plant, detecting anomalies in real time and therefore allowing the operators to investigate more in depth on the possible causes before any damage is made.
In order to do so, we had to define the "normality" of a signal, which can heavily vary from one to the other. Therefore, we setup a learning procedure that integrates several steps.
First, we need to distinguish the normal periods from the anomalous ones. While there are failures that are known to have impacted at certain times certain sections of the plant, three will definitely be more anomalies that have remained hidden so far. We hence labelled each timestamp of the series using an isolation forest algorithm.
Using the obtained normal dataset, we then extracted for each sensor the features that we have identified to better characterize a time series in its normal operating conditions. First, we select the signals in the plant that are the most correlated with the one at hand and fit a Ridge Regression and estimate the residual distribution. Then, we extract statistics such as mean, standard deviation, mean length and frequency of frozen periods, outliers, NaNs, Fast Fourier Transform and Welch’s approach for spectral density estimation. Finally, we heuristically define a number of tests capable of distinguishing a normal from an anomalous time window.
With this procedure, we are able to detect in real time whenever a signal is behaving differently from the way it is expected to. Depending on the operator’s experience, it would then be possible to understand whether it is a sensor malfunction or whether it indicates that something is wrong with the physical phenomenon, determining different actions, such as maintenance rescheduling. We have therefore conceived a dashboard that allows each operator to input its feedback, producing a refined dataset ready for continuous retraining. Ultimately, this anomaly detection framework will be used also to filter out the inputs to many advanced analytics solutions, guaranteeing the quality of their results.
Milani A.E., Antonello F., Baraldi P., Zio E.
Siahpour S., Ainapure A., Li X., Lee J.
Rocchetta R., Petkovic M., Gao Q.
Altarabichi M.G., Mashhadi P., Fan Y., Pashami S., Nowaczyk S., Moral P.D., Rahat M., Rögnvaldsson T.
Xiong R., Carleton Athey S., Bayati M., Imbens G.W.
In this paper, we study the problem of designing experiments that are conducted on a set of units such as users or groups of users in an online marketplace, for multiple time periods such as weeks or months. These experiments are particularly useful to study the treatments that have causal effects on both current and future outcomes (instantaneous and lagged effects). The design problem involves selecting a treatment time for each unit, before or during the experiment, in order to most precisely estimate the instantaneous and lagged effects, post experimentation. This optimization of the treatment decisions can directly minimize the opportunity cost of the experiment by reducing its sample size requirement. The optimization is an NP-hard integer program for which we provide a near-optimal solution, when the design decisions are performed all at the beginning (fixed-sample-size designs). Next, we study sequential experiments that allow adaptive decisions during the experiments, and also potentially early stop the experiments, further reducing their cost. However, the sequential nature of these experiments complicates both the design phase and the estimation phase. We propose a new algorithm, PGAE, that addresses these challenges by adaptively making treatment decisions, estimating the treatment effects, and drawing valid post-experimentation inference. PGAE combines ideas from Bayesian statistics, dynamic programming, and sample splitting. Using synthetic experiments on real data sets from multiple domains, we demonstrate that our proposed solutions for fixed-sample-size and sequential experiments reduce the opportunity cost of the experiments by over 50% and 70%, respectively, compared to benchmarks.
Cannarile F., Compare M., Baraldi P., Diodati G., Quaranta V., Zio E.
The objective of this work is the development of a fault diagnostic system for a shaker blower used in on-board aeronautical systems. Features extracted from condition monitoring signals and selected by the ELastic NET (ELNET) algorithm, which combines l 1 -penalty with the squared l 2 -penalty on model parameters, are used as inputs of a Multinomial Logistic regression (MLR) model. For validation, the developed approach is applied to experimental data acquired on a shaker blower system (as representative of aeronautical on-board systems) and on three additional experimental datasets of literature. The satisfactory diagnostic performances obtained show the potential of the method for developing sound diagnostic classifiers from a very large set of features, even when only few training data are available.
Cannarile F., Baraldi P., Zio E.
Data-driven methods for direct prognostic map the relationship between monitored parameters and equipment Remaining Useful Life (RUL). They typically require the availability of a set of run-to-failure degradation trajectories for model training. Yet, in many industrial applications, equipment is often replaced before they fail to avoid catastrophic consequences on production and safety. Then also, incomplete degradation trajectories are available. In this work, we develop a method for predicting equipment RUL, and the related uncertainty based on both complete and incomplete degradation trajectories. The method is based on the combined use of a similarity measure and Evidence Theory (EvT). Application of the method on two case studies shows that it provides accurate RUL predictions, also in comparison with a similarity-based regression method of literature.
Lu M.
Data heterogeneity is one of the big challenges in modern data analysis caused by the effects of unknown/unwanted factors introduced during data collection procedures. It will cause spurious estimation of variable effects when traditional methods are applied for feature selection which simply assume that data samples are independently and identically distributed. Although some existing statistical models can evaluate more accurately the significance of each variable by estimating and including unknown factors as covariates, they are categorized as filter methods suffering from variable redundancy and lack of predictability. Therefore, we propose an embedded feature selection method from a sparse learning perspective capable of adjusting unknown heterogeneity. Its performance is investigated by evaluating the classification performance using the selected features in multi-class classification problems. Benefitting from the effective adjustment of unknown heterogeneity and model selection strategy, the experimental results on synthetic data and three real-world benchmark data sets have shown that our method can achieve consistent superiority over several conventional embedded methods and existing statistical models.
Fan M., Zeng Z., Zio E., Kang R., Chen Y.
A sequential Bayesian approach is presented for remaining useful life (RUL) prediction of dependent competing failure processes (DCFP). The DCFP considered comprises of soft failure processes due to degradation and hard failure processes due to random shocks, where dependency arises due to the abrupt changes to the degradation processes brought by the random shocks. In practice, random shock processes are often unobservable, which makes it difficult to accurately estimate the shock intensities and predict the RUL. In the proposed method, the problem is solved recursively in a two-stage framework: in the first stage, parameters related to the degradation processes are updated using particle filtering, based on the degradation data observed through condition monitoring; in the second stage, the intensities of the random shock processes are updated using the Metropolis-Hastings algorithm, considering the dependency between the degradation and shock processes, and the fact that no hard failure has occurred. The updated parameters are, then, used to predict the RUL of the system. Two numerical examples are considered for demonstration purposes and a real dataset from milling machines is used for application purposes. Results show that the proposed method can be used to accurately predict the RUL in DCFP conditions.
Total publications
18
Total citations
176
Citations per publication
9.78
Average publications per year
2
Average coauthors
4.39
Publications years
2015-2023 (9 years)
h-index
5
i10-index
4
m-index
0.56
o-index
22
g-index
13
w-index
2
Metrics description
h-index
A scientist has an h-index if h of his N publications are cited at least h times each, while the remaining (N - h) publications are cited no more than h times each.
i10-index
The number of the author's publications that received at least 10 links each.
m-index
The researcher's m-index is numerically equal to the ratio of his h-index to the number of years that have passed since the first publication.
o-index
The geometric mean of the h-index and the number of citations of the most cited article of the scientist.
g-index
For a given set of articles, sorted in descending order of the number of citations that these articles received, the g-index is the largest number such that the g most cited articles received (in total) at least g2 citations.
w-index
If w articles of a researcher have at least 10w citations each and other publications are less than 10(w+1) citations, then the researcher's w-index is equal to w.
Top-100
Fields of science
1
2
3
|
|
Safety, Risk, Reliability and Quality
|
Safety, Risk, Reliability and Quality, 3, 16.67%
Safety, Risk, Reliability and Quality
3 publications, 16.67%
|
Electrical and Electronic Engineering
|
Electrical and Electronic Engineering, 2, 11.11%
Electrical and Electronic Engineering
2 publications, 11.11%
|
Computer Science (miscellaneous)
|
Computer Science (miscellaneous), 2, 11.11%
Computer Science (miscellaneous)
2 publications, 11.11%
|
Mechanical Engineering
|
Mechanical Engineering, 2, 11.11%
Mechanical Engineering
2 publications, 11.11%
|
Artificial Intelligence
|
Artificial Intelligence, 2, 11.11%
Artificial Intelligence
2 publications, 11.11%
|
Control and Systems Engineering
|
Control and Systems Engineering, 2, 11.11%
Control and Systems Engineering
2 publications, 11.11%
|
Industrial and Manufacturing Engineering
|
Industrial and Manufacturing Engineering, 1, 5.56%
Industrial and Manufacturing Engineering
1 publication, 5.56%
|
Energy Engineering and Power Technology
|
Energy Engineering and Power Technology, 1, 5.56%
Energy Engineering and Power Technology
1 publication, 5.56%
|
Nuclear Energy and Engineering
|
Nuclear Energy and Engineering, 1, 5.56%
Nuclear Energy and Engineering
1 publication, 5.56%
|
Civil and Structural Engineering
|
Civil and Structural Engineering, 1, 5.56%
Civil and Structural Engineering
1 publication, 5.56%
|
Control and Optimization
|
Control and Optimization, 1, 5.56%
Control and Optimization
1 publication, 5.56%
|
Aerospace Engineering
|
Aerospace Engineering, 1, 5.56%
Aerospace Engineering
1 publication, 5.56%
|
Logic
|
Logic, 1, 5.56%
Logic
1 publication, 5.56%
|
1
2
3
|
Journals
1
2
|
|
Proceedings of the Institution of Mechanical Engineers, Part O: Journal of Risk and Reliability
2 publications, 11.11%
|
|
Machines
1 publication, 5.56%
|
|
Lecture Notes in Computer Science
1 publication, 5.56%
|
|
Aerospace Science and Technology
1 publication, 5.56%
|
|
Engineering Applications of Artificial Intelligence
1 publication, 5.56%
|
|
International Journal of Prognostics and Health Management
1 publication, 5.56%
|
|
Annals of Nuclear Energy
1 publication, 5.56%
|
|
Fuzzy Sets and Systems
1 publication, 5.56%
|
|
1
2
|
Citing journals
5
10
15
20
25
|
|
Journal not defined
|
Journal not defined, 21, 11.8%
Journal not defined
21 citations, 11.8%
|
Machines
8 citations, 4.49%
|
|
Engineering Applications of Artificial Intelligence
8 citations, 4.49%
|
|
Mechanical Systems and Signal Processing
8 citations, 4.49%
|
|
IEEE Access
8 citations, 4.49%
|
|
Measurement: Journal of the International Measurement Confederation
6 citations, 3.37%
|
|
Reliability Engineering and System Safety
6 citations, 3.37%
|
|
Aerospace Science and Technology
6 citations, 3.37%
|
|
IEEE Transactions on Instrumentation and Measurement
5 citations, 2.81%
|
|
Measurement Science and Technology
5 citations, 2.81%
|
|
Proceedings of the Institution of Mechanical Engineers, Part O: Journal of Risk and Reliability
4 citations, 2.25%
|
|
IEEE Sensors Journal
4 citations, 2.25%
|
|
IEEE Internet of Things Journal
3 citations, 1.69%
|
|
Applied Sciences (Switzerland)
3 citations, 1.69%
|
|
Sensors
3 citations, 1.69%
|
|
Energies
3 citations, 1.69%
|
|
Lecture Notes in Computer Science
2 citations, 1.12%
|
|
Computers in Industry
2 citations, 1.12%
|
|
IFAC-PapersOnLine
2 citations, 1.12%
|
|
Artificial Intelligence Review
2 citations, 1.12%
|
|
Neurocomputing
2 citations, 1.12%
|
|
Communications in Computer and Information Science
2 citations, 1.12%
|
|
Scientific Reports
2 citations, 1.12%
|
|
Applied Soft Computing Journal
2 citations, 1.12%
|
|
Annals of Nuclear Energy
2 citations, 1.12%
|
|
Journal of Vibroengineering
1 citation, 0.56%
|
|
Micromachines
1 citation, 0.56%
|
|
IEEE Transactions on Neural Networks and Learning Systems
1 citation, 0.56%
|
|
Journal of Vibrational Engineering and Technologies
1 citation, 0.56%
|
|
Mechanics of Composite Materials
1 citation, 0.56%
|
|
Studies in Computational Intelligence
1 citation, 0.56%
|
|
Journal of Physics: Conference Series
1 citation, 0.56%
|
|
IEEE/ASME Transactions on Mechatronics
1 citation, 0.56%
|
|
Simulation Modelling Practice and Theory
1 citation, 0.56%
|
|
Complexity
1 citation, 0.56%
|
|
IEEE Transactions on Industrial Electronics
1 citation, 0.56%
|
|
Neural Computing and Applications
1 citation, 0.56%
|
|
Electronics (Switzerland)
1 citation, 0.56%
|
|
Environment Systems and Decisions
1 citation, 0.56%
|
|
Tunnelling and Underground Space Technology
1 citation, 0.56%
|
|
MATEC Web of Conferences
1 citation, 0.56%
|
|
Applied Acoustics
1 citation, 0.56%
|
|
Mobile Information Systems
1 citation, 0.56%
|
|
Chinese Journal of Mechanical Engineering (English Edition)
1 citation, 0.56%
|
|
Renewable Energy
1 citation, 0.56%
|
|
IEEE Transactions on Industrial Informatics
1 citation, 0.56%
|
|
Shock and Vibration
1 citation, 0.56%
|
|
Journal of Mechanical Science and Technology
1 citation, 0.56%
|
|
Petroleum Science and Technology
1 citation, 0.56%
|
|
Journal of Building Engineering
1 citation, 0.56%
|
|
Waste Management
1 citation, 0.56%
|
|
Infrared Physics and Technology
1 citation, 0.56%
|
|
Journal of Intelligent Systems
1 citation, 0.56%
|
|
Applied Energy
1 citation, 0.56%
|
|
Procedia Manufacturing
1 citation, 0.56%
|
|
Journal of Intelligent Manufacturing
1 citation, 0.56%
|
|
International Journal of Fuzzy Systems
1 citation, 0.56%
|
|
Advances in Intelligent Systems and Computing
1 citation, 0.56%
|
|
Procedia Computer Science
1 citation, 0.56%
|
|
Sustainability
1 citation, 0.56%
|
|
Renewable and Sustainable Energy Reviews
1 citation, 0.56%
|
|
Advanced Engineering Informatics
1 citation, 0.56%
|
|
IEEE Transactions on Control Systems Technology
1 citation, 0.56%
|
|
International Journal of Computer Integrated Manufacturing
1 citation, 0.56%
|
|
IEEE Systems Journal
1 citation, 0.56%
|
|
IEEE Transactions on Reliability
1 citation, 0.56%
|
|
Soft Computing
1 citation, 0.56%
|
|
AIP Conference Proceedings
1 citation, 0.56%
|
|
Cluster Computing
1 citation, 0.56%
|
|
Multimedia Tools and Applications
1 citation, 0.56%
|
|
International Journal of Advanced Manufacturing Technology
1 citation, 0.56%
|
|
Symmetry
1 citation, 0.56%
|
|
Biosystems Engineering
1 citation, 0.56%
|
|
Iranian Journal of Science and Technology - Transactions of Electrical Engineering
1 citation, 0.56%
|
|
Fuzzy Sets and Systems
1 citation, 0.56%
|
|
Eng—Advances in Engineering
1 citation, 0.56%
|
|
International Journal of Information Technology
1 citation, 0.56%
|
|
Vibration
1 citation, 0.56%
|
|
IEEE Transactions on Emerging Topics in Computational Intelligence
1 citation, 0.56%
|
|
Intelligent Systems with Applications
1 citation, 0.56%
|
|
Neuroscience Informatics
1 citation, 0.56%
|
|
AIMS Electronics and Electrical Engineering
1 citation, 0.56%
|
|
Advances in Computational Intelligence and Robotics
1 citation, 0.56%
|
|
JMST Advances
1 citation, 0.56%
|
|
Show all (54 more) | |
5
10
15
20
25
|
Publishers
1
2
3
4
|
|
Elsevier
4 publications, 22.22%
|
|
SAGE
2 publications, 11.11%
|
|
Springer Nature
1 publication, 5.56%
|
|
MDPI
1 publication, 5.56%
|
|
PHM Society
1 publication, 5.56%
|
|
1
2
3
4
|
Organizations from articles
2
4
6
8
10
|
|
Organization not defined
|
Organization not defined, 10, 55.56%
Organization not defined
10 publications, 55.56%
|
Polytechnic University of Milan
8 publications, 44.44%
|
|
CentraleSupélec
5 publications, 27.78%
|
|
Kyung Hee University
2 publications, 11.11%
|
|
École Nationale Supérieure des Mines de Paris
1 publication, 5.56%
|
|
Paris Sciences et Lettres
1 publication, 5.56%
|
|
2
4
6
8
10
|
Countries from articles
2
4
6
8
10
|
|
Italy
|
Italy, 10, 55.56%
Italy
10 publications, 55.56%
|
Country not defined
|
Country not defined, 8, 44.44%
Country not defined
8 publications, 44.44%
|
France
|
France, 8, 44.44%
France
8 publications, 44.44%
|
Republic of Korea
|
Republic of Korea, 2, 11.11%
Republic of Korea
2 publications, 11.11%
|
2
4
6
8
10
|
Citing organizations
5
10
15
20
25
30
35
40
45
|
|
Organization not defined
|
Organization not defined, 41, 23.3%
Organization not defined
41 citations, 23.3%
|
Polytechnic University of Milan
21 citations, 11.93%
|
|
Huazhong University of Science and Technology
7 citations, 3.98%
|
|
CentraleSupélec
7 citations, 3.98%
|
|
Shanghai Jiao Tong University
5 citations, 2.84%
|
|
University of Electronic Science and Technology of China
4 citations, 2.27%
|
|
Nanjing University of Aeronautics and Astronautics
4 citations, 2.27%
|
|
Southeast University
4 citations, 2.27%
|
|
Kyung Hee University
4 citations, 2.27%
|
|
École Nationale Supérieure des Mines de Paris
4 citations, 2.27%
|
|
Paris Sciences et Lettres
4 citations, 2.27%
|
|
Tsinghua University
3 citations, 1.7%
|
|
Beihang University
3 citations, 1.7%
|
|
Sichuan University
3 citations, 1.7%
|
|
Southwest Jiaotong University
3 citations, 1.7%
|
|
Université Paris-Saclay
3 citations, 1.7%
|
|
Savitribai Phule Pune University
2 citations, 1.14%
|
|
Symbiosis International University
2 citations, 1.14%
|
|
Duy Tan University
2 citations, 1.14%
|
|
Beijing Institute of Technology
2 citations, 1.14%
|
|
Zhejiang University
2 citations, 1.14%
|
|
University of Chinese Academy of Sciences
2 citations, 1.14%
|
|
Harbin Institute of Technology
2 citations, 1.14%
|
|
Xi'an Jiaotong University
2 citations, 1.14%
|
|
Northwestern Polytechnical University
2 citations, 1.14%
|
|
Harbin Engineering University
2 citations, 1.14%
|
|
Beijing Jiaotong University
2 citations, 1.14%
|
|
Shandong University of Science and Technology
2 citations, 1.14%
|
|
European Organization for Nuclear Research
2 citations, 1.14%
|
|
Guangdong University of Petrochemical Technology
2 citations, 1.14%
|
|
National Yang Ming Chiao Tung University
2 citations, 1.14%
|
|
University of Ulsan
2 citations, 1.14%
|
|
Ohio State University
2 citations, 1.14%
|
|
Shandong University
2 citations, 1.14%
|
|
National University of Defense Technology
2 citations, 1.14%
|
|
National Space Science Center, Chinese Academy of Sciences
2 citations, 1.14%
|
|
AGH University of Krakow
2 citations, 1.14%
|
|
University of São Paulo
2 citations, 1.14%
|
|
King Saud University
1 citation, 0.57%
|
|
University of Tehran
1 citation, 0.57%
|
|
National Institute of Technology Tiruchirappalli
1 citation, 0.57%
|
|
Kalinga Institute of Industrial Technology
1 citation, 0.57%
|
|
Thapar Institute of Engineering and Technology
1 citation, 0.57%
|
|
NED University of Engineering and Technology
1 citation, 0.57%
|
|
University of Isfahan
1 citation, 0.57%
|
|
Karadeniz Technical University
1 citation, 0.57%
|
|
Shahrekord University
1 citation, 0.57%
|
|
SRM Institute of Science and Technology
1 citation, 0.57%
|
|
Fasa University
1 citation, 0.57%
|
|
Tongji University
1 citation, 0.57%
|
|
Katholieke Universiteit Leuven
1 citation, 0.57%
|
|
University of Lorraine
1 citation, 0.57%
|
|
KTH Royal Institute of Technology
1 citation, 0.57%
|
|
Multimedia University
1 citation, 0.57%
|
|
Goa University
1 citation, 0.57%
|
|
Nanjing University of Science and Technology
1 citation, 0.57%
|
|
China University of Geosciences (Wuhan)
1 citation, 0.57%
|
|
Lulea University of Technology
1 citation, 0.57%
|
|
German Jordanian University
1 citation, 0.57%
|
|
Chongqing University
1 citation, 0.57%
|
|
Yanshan University
1 citation, 0.57%
|
|
China Agricultural University
1 citation, 0.57%
|
|
University of Science and Technology Beijing
1 citation, 0.57%
|
|
Dalian Maritime University
1 citation, 0.57%
|
|
University of Oulu
1 citation, 0.57%
|
|
Norwegian University of Science and Technology
1 citation, 0.57%
|
|
Tianjin University
1 citation, 0.57%
|
|
University of Shanghai for Science and Technology
1 citation, 0.57%
|
|
China Three Gorges University
1 citation, 0.57%
|
|
University of Liverpool
1 citation, 0.57%
|
|
Huaiyin Institute of Technology
1 citation, 0.57%
|
|
University of Nottingham
1 citation, 0.57%
|
|
Dongguan University of Technology
1 citation, 0.57%
|
|
Xihua University
1 citation, 0.57%
|
|
Shenyang University of Technology
1 citation, 0.57%
|
|
National Taiwan Normal University
1 citation, 0.57%
|
|
Chaoyang University of Technology
1 citation, 0.57%
|
|
Harbin Normal University
1 citation, 0.57%
|
|
Heilongjiang University
1 citation, 0.57%
|
|
Xi'an University of Technology
1 citation, 0.57%
|
|
University of Pisa
1 citation, 0.57%
|
|
University of Wollongong
1 citation, 0.57%
|
|
Seoul National University
1 citation, 0.57%
|
|
City University of Hong Kong
1 citation, 0.57%
|
|
Hong Kong Polytechnic University
1 citation, 0.57%
|
|
West Virginia University
1 citation, 0.57%
|
|
Case Western Reserve University
1 citation, 0.57%
|
|
Soongsil University
1 citation, 0.57%
|
|
Harvard University
1 citation, 0.57%
|
|
New York University
1 citation, 0.57%
|
|
University of California, Davis
1 citation, 0.57%
|
|
Shaoxing University
1 citation, 0.57%
|
|
Henan University of Technology
1 citation, 0.57%
|
|
Nanyang Institute of Technology
1 citation, 0.57%
|
|
Woosong University
1 citation, 0.57%
|
|
Hunan University
1 citation, 0.57%
|
|
Wenzhou University
1 citation, 0.57%
|
|
University of Luxembourg
1 citation, 0.57%
|
|
International Hellenic University
1 citation, 0.57%
|
|
Federal University of Pernambuco
1 citation, 0.57%
|
|
Show all (70 more) | |
5
10
15
20
25
30
35
40
45
|
Citing countries
10
20
30
40
50
60
70
80
|
|
China
|
China, 73, 41.48%
China
73 citations, 41.48%
|
Italy
|
Italy, 27, 15.34%
Italy
27 citations, 15.34%
|
Country not defined
|
Country not defined, 22, 12.5%
Country not defined
22 citations, 12.5%
|
France
|
France, 16, 9.09%
France
16 citations, 9.09%
|
USA
|
USA, 13, 7.39%
USA
13 citations, 7.39%
|
Republic of Korea
|
Republic of Korea, 9, 5.11%
Republic of Korea
9 citations, 5.11%
|
India
|
India, 8, 4.55%
India
8 citations, 4.55%
|
United Kingdom
|
United Kingdom, 5, 2.84%
United Kingdom
5 citations, 2.84%
|
Brazil
|
Brazil, 4, 2.27%
Brazil
4 citations, 2.27%
|
Canada
|
Canada, 4, 2.27%
Canada
4 citations, 2.27%
|
Sweden
|
Sweden, 3, 1.7%
Sweden
3 citations, 1.7%
|
Germany
|
Germany, 2, 1.14%
Germany
2 citations, 1.14%
|
Portugal
|
Portugal, 2, 1.14%
Portugal
2 citations, 1.14%
|
Australia
|
Australia, 2, 1.14%
Australia
2 citations, 1.14%
|
Vietnam
|
Vietnam, 2, 1.14%
Vietnam
2 citations, 1.14%
|
Iran
|
Iran, 2, 1.14%
Iran
2 citations, 1.14%
|
Spain
|
Spain, 2, 1.14%
Spain
2 citations, 1.14%
|
Mexico
|
Mexico, 2, 1.14%
Mexico
2 citations, 1.14%
|
Poland
|
Poland, 2, 1.14%
Poland
2 citations, 1.14%
|
Switzerland
|
Switzerland, 2, 1.14%
Switzerland
2 citations, 1.14%
|
Algeria
|
Algeria, 1, 0.57%
Algeria
1 citation, 0.57%
|
Belgium
|
Belgium, 1, 0.57%
Belgium
1 citation, 0.57%
|
Greece
|
Greece, 1, 0.57%
Greece
1 citation, 0.57%
|
Egypt
|
Egypt, 1, 0.57%
Egypt
1 citation, 0.57%
|
Indonesia
|
Indonesia, 1, 0.57%
Indonesia
1 citation, 0.57%
|
Jordan
|
Jordan, 1, 0.57%
Jordan
1 citation, 0.57%
|
Latvia
|
Latvia, 1, 0.57%
Latvia
1 citation, 0.57%
|
Lithuania
|
Lithuania, 1, 0.57%
Lithuania
1 citation, 0.57%
|
Luxembourg
|
Luxembourg, 1, 0.57%
Luxembourg
1 citation, 0.57%
|
Malaysia
|
Malaysia, 1, 0.57%
Malaysia
1 citation, 0.57%
|
Netherlands
|
Netherlands, 1, 0.57%
Netherlands
1 citation, 0.57%
|
Norway
|
Norway, 1, 0.57%
Norway
1 citation, 0.57%
|
Pakistan
|
Pakistan, 1, 0.57%
Pakistan
1 citation, 0.57%
|
Saudi Arabia
|
Saudi Arabia, 1, 0.57%
Saudi Arabia
1 citation, 0.57%
|
North Macedonia
|
North Macedonia, 1, 0.57%
North Macedonia
1 citation, 0.57%
|
Serbia
|
Serbia, 1, 0.57%
Serbia
1 citation, 0.57%
|
Turkey
|
Turkey, 1, 0.57%
Turkey
1 citation, 0.57%
|
Finland
|
Finland, 1, 0.57%
Finland
1 citation, 0.57%
|
Czech Republic
|
Czech Republic, 1, 0.57%
Czech Republic
1 citation, 0.57%
|
Chile
|
Chile, 1, 0.57%
Chile
1 citation, 0.57%
|
Japan
|
Japan, 1, 0.57%
Japan
1 citation, 0.57%
|
Show all (11 more) | |
10
20
30
40
50
60
70
80
|
- We do not take into account publications without a DOI.
- Statistics recalculated daily.
This section displays the profiles of scientists registered on the platform. To display the full list, invite your colleagues to register.