Singh, Sushant K
Publications
49
Citations
1 642
h-index
17
- Advances in Space Research (1)
- Advances in Water Security (1)
- AIMS Medical Science (1)
- AIMS Public Health (1)
- Applied Sciences (Switzerland) (1)
- Catena (2)
- Clinica Chimica Acta (1)
- Current Science (1)
- Ecotoxicology and Environmental Safety (1)
- Emerging Contaminants (1)
- Environment (1)
- Environment Systems and Decisions (1)
- Environmental Science and Pollution Research (1)
- Frontiers in Ecology and the Environment (1)
- Frontiers in Environmental Science (1)
- Geocarto International (3)
- Groundwater for Sustainable Development (1)
- International Journal of Environmental Research and Public Health (2)
- International Journal of Structural Integrity (1)
- International Journal of Sustainable Built Environment (1)
- Journal of Environmental Management (1)
- Mathematical Problems in Engineering (1)
- Natural Hazards (1)
- Open Geospatial Data, Software and Standards (1)
- Quaternary Science Reviews (1)
- Sustainability (2)
- Vietnam Journal of Earth Sciences (1)
Nothing found, try to update filter.
Singh S.K.
This opinion article explores the evolving responsibilities of data scientists in the current data-driven landscape, in which ethical, privacy, and governance standards have grown considerably in importance. Although the job of data scientist initially attracted attention for its allure and high earning potential, in recent years, it has become associated with a particularly high level of responsibility, requiring practitioners to balance their technical skills with a commitment to social impact and accountability. This article examines the essential qualifications and criteria of a responsible data scientist, including a robust ethical awareness, an understanding of privacy safeguards and transparency, and a commitment to continuous learning. This article also discusses hiring practices that prioritize these qualities and outlines strategies for fostering a data-driven culture grounded in responsibility and trust. In the current landscape, responsible data scientists not only analyze data but also protect ethical data practices, which is crucial to building a transparent, fair, and sustainable digital world. This article also provides a framework and guidelines for identifying and recruiting responsible data scientists.
K. Singh S.
Prasad P., Singh S.K., Ghosh S., Dutta S., Sinha D.
The metalloid arsenic (As) induces oxidative stress is a well-known fact. However, the extent of variation of oxidative stress according to different exposure levels of As in groundwater and the mechanism responsible for As mediated oxidative stress is yet to be elucidated in a human population of West Bengal. In the present study, we have investigated the impact of low level (> 10 ≤ 50 µg/L) and high-level groundwater As (> 50 µg/L) on cellular redox status, DNA damage, and repair mechanisms in chronically exposed rural women of West Bengal. Prediction models of ordinary least square regression of nail As, forced vital capacity (FVC) %, and that of forced expiratory volume during the first one second (FEV1) % deciphered that accumulation of As in nails may predict hemoglobin deficiency. Moreover, consumption of As-laced water tends to decrease FEV1% and FVC%. A strong positive correlation was observed between water and nail As level and reactive oxygen species (ROS) generation. ROS, perturbed nuclear factor erythroid 2–related factor 2(Nrf2)/ Kelch-like ECH-associated protein 1 (Keap1) redox regulation, compromised antioxidant defense machinery-superoxide dismutase (SOD), glutathione peroxidase (GPx), glutathione transferase (GST), induced DNA damage, and suppressed DNA repair proteins-poly ADP ribose polymerase1(PARP1)/ X-ray repair cross-complementing protein 1(XRCC1)/ 8-oxoguanine glycosylase (OGG1) in a dose-dependent manner. All the low and high As areas had very high cancer risk values for the exposed population. It has been predicted that if the As level in the drinking water of a 40-year adult increases by 2 ug/L, the likelihood of the cancer risk will increase by 10%, keeping the body weight and amount of water intake constant. Thus, long-term exposure to either low or high As is seriously affecting the lives of asymptomatic women who are vulnerable to developing carcinogenic changes after a period of latency.
Ghasemian B., Shahabi H., Shirzadi A., Al-Ansari N., Jaafari A., Geertsema M., Melesse A.M., Singh S.K., Ahmad A.
Landslides can be a major challenge in mountainous areas that are influenced by climate and landscape changes. In this study, we propose a hybrid machine learning model based on a rotation forest (RoF) meta classifier and a random forest (RF) decision tree classifier called RoFRF for landslide prediction in a mountainous area near Kamyaran city, Kurdistan Province, Iran. We used 118 landslide locations and 25 conditioning factors from which their predictive usefulness was measured using the chi-square technique in a 10-fold cross-validation analysis. We used the sensitivity, specificity, accuracy, F1-measure, Kappa, and area under the receiver operating characteristic curve (AUC) to validate the performance of the proposed model compared to the Artificial Neural Network (ANN), Logistic Model Tree (LMT), Best First Tree (BFT), and RF models. The validation results demonstrated that the landslide susceptibility map produced by the hybrid model had the highest goodness-of-fit (AUC = 0.953) and higher prediction accuracy (AUC = 0.919) compared to the benchmark models. The hybrid RoFRF model proposed in this study can be used as a robust predictive model for landslide susceptibility mapping in the mountainous regions around the world.
Singh S.K., Taylor R.W., Pradhan B., Shirzadi A., Pham B.T.
This study evaluates state-of-the-art machine learning models in predicting the most sustainable arsenic mitigation preference. A Gaussian distribution-based Naïve Bayes (NB) classifier scored the highest Area Under the Curve (AUC) of the Receiver Operating Characteristic curve (0.82), followed by Nu Support Vector Classification (0.80), and K-Neighbors (0.79). Ensemble classifiers scored higher than 70% AUC, with Random Forest being the top performer (0.77), and Decision Tree model ranked fourth with an AUC of 0.77. The multilayer perceptron model also achieved high performance (AUC=0.75). Most linear classifiers underperformed, with the Ridge classifier at the top (AUC=0.73) and perceptron at the bottom (AUC=0.57). A Bernoulli distribution-based Naïve Bayes classifier was the poorest model (AUC=0.50). The Gaussian NB was also the most robust ML model with the slightest variation of Kappa score on training (0.58) and test data (0.64). The results suggest that nonlinear or ensemble classifiers could more accurately understand the complex relationships of socio-environmental data and help develop accurate and robust prediction models of sustainable arsenic mitigation. Furthermore, Gaussian NB is the best option when data is scarce.
Singh S.K., Taylor R.W., Thadaboina V.
This study uses six machine learning (ML) algorithms to evaluate and predict individuals' social resilience towards arsenicosis-affected people in an arsenic-risk society of rural India. Over 50% of the surveyed communities were found to be resilient towards arsenicosis patients. Logistic regression with inbuilt cross-validation (LRCV) model scored the highest accuracy (76%), followed by Gaussian distribution-based naïve Bayes (GNB) model (74%), C-Support Vector (SVC) (74%), K-neighbors (Kn) (73%), Random Forest (RF) (72%), and Decision Tree (DT) (67%). The LRCV also scored the highest kappa value of 0.52, followed by GNB (0.48), SVC (0.48), Kn (0.46), RF (0.42), and DT (0.31). Caste, education, occupation, housing status, sanitation behaviors, trust in others, non-profit and private organizations, social capital, and awareness played a key role in shaping social resilience towards arsenicosis patients. The authors opine that LRCV and GNB could be promising methods to develop models on similar data generated from a risk society.
Singh S.K., Shirzadi A., Pham B.T.
This study presents a critical review of the application of artificial intelligence (AI) in developing prediction models of globally concerning groundwater contaminants, including arsenic, fluoride, and nitrate. Groundwater is arsenic-contaminated in 109 countries, fluoride-contaminated in 84, and nitrate-polluted in 60. Cumulatively, these groundwater contaminations adversely impact the lives of more than 300 million of these countries’ inhabitants. Twenty-four countries have problems with all three contaminants. Fifty-nine countries have issues with arsenic and fluoride in their groundwater, and 63 have at least two groundwater-contamination problems. An array of AI techniques, including machine learning (ML) and deep learning (DL), has been applied in developing arsenic-, fluoride-, and nitrate-prediction models, the most frequently used models being logistic regressions and random forests. We recommend developing such prediction models with substantially larger datasets and meaningful, statistically significant predictors. Considering that contamination is highly regional, it is also advisable to develop local prediction models and evaluate at least several robust and relevant algorithms when it comes to final model selection. Hybrid ML–DL models could be especially useful, considering the many-dimensional nature of environmental data. Environmental scientists should also be trained in various AI techniques. AI prediction models can be used as a decision-support system and to create proactive environmental-management policies.
Pham B.T., Phong T.V., Avand M., Al-Ansari N., Singh S.K., Le H.V., Prakash I.
In this study, the main aim is to improve performance of the voting feature intervals (VFIs), which is one of the most effective machine learning models, using two robust ensemble techniques, namely, AdaBoost and MultiBoost for landslide susceptibility assessment and prediction. For this, two hybrid models, namely, AdaBoost-based Voting Feature Intervals (ABVFIs) and MultiBoost-based Voting Feature Intervals (MBVFIs) were developed and validated using landslide data collected from one of the landslide affected districts of Vietnam, namely, Muong Lay. Quantitative validation methods including area under the ROC curve (AUC) were used to evaluate model performance. The results indicated that both the newly developed ensemble models ABVFI (AUC = 0.859) and MBVFI (AUC = 0.839) outperformed the single VFI (AUC = 0.824) model. Thus, ensemble framework-based VFI algorithms can be used for the accurate spatial prediction of landslides, which can also be applied in other landslide prone regions of the world. Landslide susceptibility maps developed by ensemble VFI models can be used for better landslide prevention and risk management of the area.
Pham T.B., Singh S.K., Ly H.
Soil Coefficient of Consolidation (Cv) is a crucial mechanical parameter and used to characterize whether the soil undergoes consolidation or compaction when subjected to pressure. In order to define such a parameter, the experimental approaches are costly, time-consuming, and required appropriate equipment to perform the tests. In this study, the development of an alternative manner to estimate the Cv, based on Artificial Neural Network (ANN), was conducted. A database containing 188 tests was used to develop the ANN model. Two structures of ANN were considered, and the accuracy of each model was assessed using common statistical measurements such as the coefficient of determination (R2), root mean square error (RMSE), and mean absolute error (MAE). In performing 600 simulations in each case, the ANN structure containing 14 neurons was statistically superior to the other one. Finally, a typical ANN result was presented to prove that it can be an excellent predictor of the problem, with a satisfying accuracy performance that yielded of RMSE = 0.0614, MAE = 0.0415, and R2 = 0.99727. This study might help in quick and accurate prediction of the Cv used in civil engineering problems.
Pham B.T., Phong T.V., Nguyen-Thoi T., Trinh P.T., Tran Q.C., Ho L.S., Singh S.K., Duyen T.T., Nguyen L.T., Le H.Q., Le H.V., Hanh N.T., Quoc N.K., Prakash I.
Landslide susceptibility mapping has become one of the most important tools for the management of landslide hazards. In this study, we proposed a novel approach to improve the performance of Credal Decision Tree (CDT) by using four ensemble frameworks: Bagging, Dagging, Decorate, and Rotation Forest (RF) for landslide susceptibility mapping. A total number of 180 past and present landslides data of the Muong Lay district (Viet Nam) was analyzed and used for generating training and validation of the models. Several standard statistical performance evaluation metrics, such as negative predictive value, positive predictive value, root mean square error, accuracy, sensitivity, specificity, Kappa, Area Under the receiver operating Characteristic curve (AUC) were used to evaluate performance of the models. Results indicated that all the developed and applied models performed well (AUC: 0.842–0.886) but performance of the RF-CDT (AUC: 0.886) model is the best. Therefore, the RF-CDT ensemble model can be used for the correct landslide susceptibility mapping and for proper landslide management not only of the study area but also other hilly areas of the world.
Nhu V., Zandi D., Shahabi H., Chapi K., Shirzadi A., Al-Ansari N., Singh S.K., Dou J., Nguyen H.
This paper aims to apply and compare the performance of the three machine learning algorithms–support vector machine (SVM), bayesian logistic regression (BLR), and alternating decision tree (ADTree)–to map landslide susceptibility along the mountainous road of the Salavat Abad saddle, Kurdistan province, Iran. We identified 66 shallow landslide locations, based on field surveys, by recording the locations of the landslides by a global position System (GPS), Google Earth imagery and black-and-white aerial photographs (scale 1: 20,000) and 19 landslide conditioning factors, then tested these factors using the information gain ratio (IGR) technique. We checked the validity of the models using statistical metrics, including sensitivity, specificity, accuracy, kappa, root mean square error (RMSE), and area under the receiver operating characteristic curve (AUC). We found that, although all three machine learning algorithms yielded excellent performance, the SVM algorithm (AUC = 0.984) slightly outperformed the BLR (AUC = 0.980), and ADTree (AUC = 0.977) algorithms. We observed that not only all three algorithms are useful and effective tools for identifying shallow landslide-prone areas but also the BLR algorithm can be used such as the SVM algorithm as a soft computing benchmark algorithm to check the performance of the models in future.
K Singh S.
This article presents the status of countries affected by COVID-19 (as of mid-May 2020) and their preparedness to combat the after-effects of the pandemic. The report also provides an analysis of how human behavior may have triggered such a global pandemic and why humans need to consider living sustainably to make our future world livable for all. COVID-19 originated in the city of Wuhan, China in December 2019. As of mid-May, it has spread to 213 countries and territories worldwide. The World Health Organization has declared COVID-19 a global pandemic, with a death toll of over 300,000 to date. The U.S. is currently the most impacted country. Collaborative efforts of scientists and politicians across the world will be needed to better plan and utilize global health resources to combat this global pandemic. Machine learning-based prediction models could also help by identifying potential COVID-19-prone areas and individuals. The cause of the emergence of COVID-19 is still a matter of research; however, one consistent theme is humanity's unsustainable behavior. By sustainably interacting with nature, humans may have avoided this pandemic. If unsustainable human practices are not controlled through education, awareness, behavioral change, as well as sustainable policy creation and enforcement, there could be several such pandemics in our future.
K. Singh S.
Citation: Sushant K. Singh. Global decision support dashboard of COVID-19[J]. AIMS Medical Science, 2020, 7(2): 40-42. doi: 10.3934/medsci.2020003
Nhu V., Shirzadi A., Shahabi H., Singh S.K., Al-Ansari N., Clague J.J., Jaafari A., Chen W., Miraki S., Dou J., Luu C., Górski K., Thai Pham B., Nguyen H.D., Ahmad B.B.
Shallow landslides damage buildings and other infrastructure, disrupt agriculture practices, and can cause social upheaval and loss of life. As a result, many scientists study the phenomenon, and some of them have focused on producing landslide susceptibility maps that can be used by land-use managers to reduce injury and damage. This paper contributes to this effort by comparing the power and effectiveness of five machine learning, benchmark algorithms—Logistic Model Tree, Logistic Regression, Naïve Bayes Tree, Artificial Neural Network, and Support Vector Machine—in creating a reliable shallow landslide susceptibility map for Bijar City in Kurdistan province, Iran. Twenty conditioning factors were applied to 111 shallow landslides and tested using the One-R attribute evaluation (ORAE) technique for modeling and validation processes. The performance of the models was assessed by statistical-based indexes including sensitivity, specificity, accuracy, mean absolute error (MAE), root mean square error (RMSE), and area under the receiver operatic characteristic curve (AUC). Results indicate that all the five machine learning models performed well for shallow landslide susceptibility assessment, but the Logistic Model Tree model (AUC = 0.932) had the highest goodness-of-fit and prediction accuracy, followed by the Logistic Regression (AUC = 0.932), Naïve Bayes Tree (AUC = 0.864), ANN (AUC = 0.860), and Support Vector Machine (AUC = 0.834) models. Therefore, we recommend the use of the Logistic Model Tree model in shallow landslide mapping programs in semi-arid regions to help decision makers, planners, land-use managers, and government agencies mitigate the hazard and risk.
Nothing found, try to update filter.
Ke C., Sun P., Zhang S., Li R., Sang K.
This study aims to assess the sensitivity of landslide susceptibility mapping (LSM) to various sampling strategies used for non-landslide samples. The study area is Tianshui city, Gansu province, China. Three types of landslide samples, combined with four machine learning models, resulted in a total of 12 scenarios. The receiver operating characteristic curve (ROC), landslide susceptibility index and the mapping distribution characteristics were calculated to access the influences of different sampling strategies and models. The results indicate that the low susceptibility areas sampling strategy yields the highest accuracy for the landslide susceptibility prediction model, followed by the stratified sampling from engineering geological petrofabric (EGP) strategy, and lastly, the random sampling strategy. Analyzing from the perspective of factor importance and the distribution law of landslide susceptibility index under each model, the models employing the stratified sampling from EGP strategy demonstrate greater robustness. In contrast, the models using the random sampling strategy exhibit lower precision and more randomness. In general, the coupled model exhibits strong performance, the frequency ratio coupled adaptive boosting model (FR-AB) demonstrates high sensitivity, while the other models are characterized by their generalizability and robustness. The results reveal the effects of non-landslide sampling strategies and different coupled models on the prediction performance of landslide susceptibility mapping, which provides a reference for subsequent researchers to obtain more reasonable landslide susceptibility mapping.
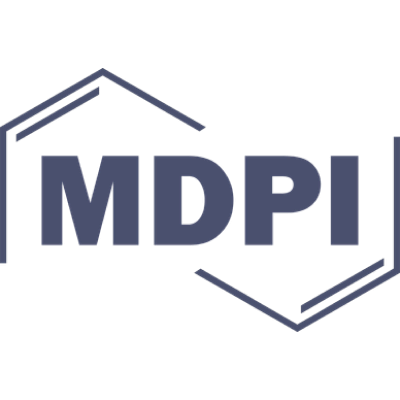
Moges W.K., Tegegne A.S., Mitku A.A., Tesfahun E., Hailemeskel S.
Low birth weight (LBW) is a critical global health issue that affects infants disproportionately, particularly in developing countries. This study adopted causal machine learning (CML) algorithms for predicting LBW in newborns, drawing from midwife-led continuity care (MLCC). A quasi-experimental study was carried out in the North Shoa Zone of Ethiopia from August 2019 to September 2020. A total of 1166 women were allocated into two groups. The first group, the MLCC group, received all their antenatal, labor, birth, and immediate post-natal care from a single midwife. The second group received care from various staff members at different times throughout their pregnancy and childbirth. In this study, CML was implemented to predict LBW. Data preprocessing, including data cleaning, was conducted. CML was then employed to identify the most suitable classifier for predicting LBW. Gradient boosting algorithms were used to estimate the causal effect of MLCC on LBW. Moreover, meta-learner algorithms were utilized to estimate the individual treatment effect (ITE), the average treatment effect (ATE), and performance. Moreover, meta-learner algorithms were utilized to estimate the individual treatment effect (ITE), the average treatment effect (ATE), and performance. The study results revealed that Causal K-Nearest Neighbors (CKNN) was the most effective classifier based on accuracy and estimated LBW using a 94.52% accuracy, 90.25% precision, 92.57% recall, and an F1 score of 88.2%. Meconium aspiration, perinatal mortality, pregnancy-induced hypertension, vacuum babies in need of resuscitation, and previous surgeries on their reproductive organs were identified as the top five features affecting LBW. The estimated impact of MLCC versus other professional groups on LBW was analyzed using gradient boosting algorithms and was found to be 0.237. The estimated ATE for the S-learner was 0.284, which is lower than the true ATE of 0.216. Additionally, the estimated ITE for both the T-learner and X-learner was less than -0.5, indicating that mothers would not choose to participate in the MLCC program. Based on these findings, the CKNN classifier demonstrated a higher accuracy and effectiveness. The S-learner and R-learner models, utilizing the XGBoost Regressor and BaseSRegressor, provided accurate estimations of ITE for assessing the impact of the MLCC program. Promoting the MLCC program could help stabilize LBW outcomes.
Chen W., Wang C., Zhao X., Bai L., He Q., Chen X., Zhao Q., Zhao R., Li T., Tsangaratos P., Ilia I.
Landslide, as a significant global natural hazard, threatening human settlements and the natural environment. The present study introduces a novel approach to landslide susceptibility assessment by integrating the Forest Attribute Penalty (FPA) model with three ensemble algorithms—AdaBoost (AB), Rotation Forest (RF), and Random Subspace (RS)—and utilizing the Evidential Belief Function (EBF) to weight the classes of landslide-related factors. To evaluate the performance of the developed methodology, Yanchuan County, China, was chosen as the appropriate study area. Three hundred and eleven landslide areas were identified through remote sensing and field investigations, which were randomly divided into 70% for model training and 30% for model evaluation, whereas sixteen landslide – related factors were considered, such as elevation, slope aspect, profile curvature, plan curvature, convergence index, slope length, terrain ruggedness index, topographic position index, distance to roads, distance to rivers, NDVI, land use, soil, rainfall, and lithology. EBF was employed to analyze the spatial correlation between these factors and landslide occurrences, providing the class weights of each factor for the implementation of FPA and the ensemble models. The next step involved the generation of the landslide susceptibility maps based on the models, with findings showing that more than half of the study area is classified as very low susceptibility. Model performance was assessed using receiver operating characteristic (ROC) curves and other statistical metrics, with the RFFPA model achieving the highest predictive ability, with AUC values of 0.878 and 0.890 for training and validation datasets, respectively. The AFPA and RSFPA hybrid models, however, demonstrated weaker predictive abilities compared to the FPA model. The study highlights the importance of optimizing model performance and evaluating the suitability of ensemble approaches, emphasizing the role of topographical and environmental settings in influencing model accuracy. The use of EBF for weight calculation proved crucial in improving model outcomes, suggesting that this approach could be further refined and adapted to other regions with similar geomorphological settings for better land use planning and risk management.
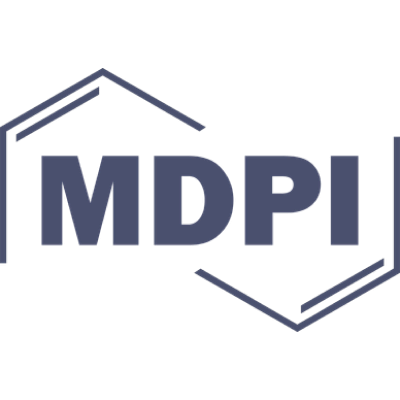
Nothing found, try to update filter.
Zhou W., Guo Q., Lei J., Yu L., Hwang J.
Using attention mechanisms in saliency detection networks enables effective feature extraction, and using linear methods can promote proper feature fusion, as verified in numerous existing models. Current networks usually combine depth maps with red-green-blue (RGB) images for salient object detection (SOD). However, fully leveraging depth information complementary to RGB information by accurately highlighting salient objects deserves further study. We combine a gated attention mechanism and a linear fusion method to construct a dual-stream interactive recursive feature-reshaping network (IRFR-Net). The streams for RGB and depth data communicate through a backbone encoder to thoroughly extract complementary information. First, we design a context extraction module (CEM) to obtain low-level depth foreground information. Subsequently, the gated attention fusion module (GAFM) is applied to the RGB depth (RGB-D) information to obtain advantageous structural and spatial fusion features. Then, adjacent depth information is globally integrated to obtain complementary context features. We also introduce a weighted atrous spatial pyramid pooling (WASPP) module to extract the multiscale local information of depth features. Finally, global and local features are fused in a bottom-up scheme to effectively highlight salient objects. Comprehensive experiments on eight representative datasets demonstrate that the proposed IRFR-Net outperforms 11 state-of-the-art (SOTA) RGB-D approaches in various evaluation indicators.
Zhou Y., Xu G., Tang K., Tian L., Sun Y.
Chinese second generation of the Audio Video Coding Standard, known as the AVS2, competing with HEVC/H.265 and AV1, has become a well-known video compression standard. Many unique tools have been developed and incorporated in AVS2. This paper proposes several encoder optimization schemes with respect to rate-distortion optimization (RDO). Specifically, by exploiting the close relationship between the total number of referenced pictures and the hierarchy of reference structure, a reference structure determined parameter (RSDP) algorithm reallocates Lagrange multiplier. Moreover, an adaptive quantization parameter offset (AQPO) algorithm is provided at both picture-level and Groups of Pictures (GoP)-level to adjust quantization parameters adaptively on top of the preassigned ones. Both RSDP algorithm and AQPO algorithm have already been adopted by AVS2 and incorporated in its reference software. Experimental results show that in the low-delay, RSDP and AQPO achieved savings of 1.35% and 1.26% on BDBR, respectively. • A frame level QP and λ allocation named reference structure determined parameter (RSDP) algorithm is proposed to satisfy GoP length 4, 8, and 16 respectively. • A GoP level QP and λ allocation named adaptive quantization parameter offset (AQPO) algorithm is proposed to deal with low-delay configuration. • Both RSDP and AQPO algorithms have achieved remarkable coding performance under BD-rate statistic units.
Ghasemian B., Shahabi H., Shirzadi A., Al-Ansari N., Jaafari A., Kress V.R., Geertsema M., Renoud S., Ahmad A.
We mapped landslide susceptibility in Kamyaran city of Kurdistan Province, Iran, using a robust deep-learning (DP) model based on a combination of extreme learning machine (ELM), deep belief network (DBN), back propagation (BP), and genetic algorithm (GA). A total of 118 landslide locations were recorded and divided in the training and testing datasets. We selected 25 conditioning factors, and of these, we specified the most important ones by an information gain ratio (IGR) technique. We assessed the performance of the DP model using statistical measures including sensitivity, specificity, accuracy, F1-measure, and area under-the-receiver operating characteristic curve (AUC). Three benchmark algorithms, i.e., support vector machine (SVM), REPTree, and NBTree, were used to check the applicability of the proposed model. The results by IGR concluded that of the 25 conditioning factors, only 16 factors were important for our modeling procedure, and of these, distance to road, road density, lithology and land use were the four most significant factors. Results based on the testing dataset revealed that the DP model had the highest accuracy (0.926) of the compared algorithms, followed by NBTree (0.917), REPTree (0.903), and SVM (0.894). The landslide susceptibility maps prepared from the DP model with AUC = 0.870 performed the best. We consider the DP model a suitable tool for landslide susceptibility mapping.
Yin L., Wang L., Keim B.D., Konsoer K., Zheng W.
The Yangtze River has been the primary support of the resources and transportation of China. The Three Gorges Dam and Reservoir on the Yangtze River is one of the world’s largest dams. The influence caused by the dam and reservoir on the river system has been overwhelming and destructive. For better water resource use and flood-prevention planning, more understanding is needed regarding the dam’s impact on river discharge, regional precipitation, and frequency of extreme rainfall events. This study aims to analyze the changes in river discharge and regional precipitation records before and after the construction of the Three Gorges Dam. This research examines temporal correlations among these data by collecting daily dam injection and dam discharge records, the precipitation from ground stations, and river discharge. The time series are analyzed with the wavelet analysis. The precipitation datasets decrease in wavelet magnitude after 1998 when the dam was built in the wavelet analysis. The annual cycle, shown as a bright year line through the time range, still exists in the analysis result after 1998, but the magnitude of the annual cycle has reduced. The river discharge shows a decrease of wavelet magnitude at the three downstream locations. The possible explanation of this pattern could be the human-controlled dam discharge. The constant water level maintained in the reservoir by human control would slow down the flow speed and stabilize it.
Yin L., Wang L., Zheng W., Ge L., Tian J., Liu Y., Yang B., Liu S.
Swarm-C satellite, a new instrument for atmospheric study, has been the focus of many studies to evaluate its usage and accuracy. This paper takes the Swarm-C satellite as a research object to verify the Swarm-C accelerometer’s inversion results. This paper uses the two-row orbital elements density inversion to verify the atmospheric density accuracy results of the Swarm-C satellite accelerometer. After the accuracy of the satellite data is verified, this paper conducts comparative verification and empirical atmospheric model evaluation experiments based on the Swarm-C accelerometer’s inversion results. After comparing with the inversion results of the Swarm-C semi-major axis attenuation method, it is found that the atmospheric density obtained by inversion using the Swarm-C accelerometer is more dynamic and real-time. It shows that with more available data, the Swarm-C satellite could be a new high-quality instrument for related studies along with the well-established satellites. After evaluating the performance of the JB2008 and NRLMSISE-00 empirical atmospheric models using the Swarm-C accelerometer inversion results, it is found that the accuracy and real-time performance of the JB2008 model at the altitude where the Swarm-C satellite is located are better than the NRLMSISE-00 model.
Liu B., Spiekermann R., Zhao C., Püttmann W., Sun Y., Jasper A., Uhl D.
Charcoal remains and bulk lignites collected from the late Pliocene Jinsuo Basin in Yunnan, southwestern China, have been studied to reveal changes in the wildfire regime related to changes of the palaeoenvironment, palaeoclimate, and paleobotany. Different types of wildfire occurred in this paleomire with a predominance of low-temperature surface fires, as indicated by mean inertinite reflectance (Ro) values ranging from 1% to 2% in most samples. High-temperature fires are less recorded and occurred more frequently in dark layers compared with pale layers and charcoal layers, as deduced from the elevated proportion of high Ro values (>3%) produced by crown fires or high-temperature surface fires. Wildfire distribution in pale and dark layers was probably influenced by, changes of both plant community and of the depositional environment during their formation. Charcoal layers represent in situ surface and ground fires, whereas pale and dark layers probably record both in situ and remote fire events inside and/or around the basin. PAH were detected in all samples throughout the whole lignite seam, indicating that burning temperatures were low (
Zhang K., Shalehy M.H., Ezaz G.T., Chakraborty A., Mohib K.M., Liu L.
Accurately quantifying and assessing the flood risks is critical for flood hazard mitigation and prevention. This study proposed a new integrated flood risk assessment framework to map flood risk, hazard and vulnerability by coupling the HEC-HMS hydrological model, the HEC-RAS 1D and 2D hydraulic models, and a bottom-up hazard vulnerability analysis. The Old Brahmaputra River floodplain of Bangladesh, a flood-prone region, was chosen as a case study. The coupled hydrological-hydraulic model shows a comparable robust performance in both calibration and validation periods with Nash-Sutcliffe efficiency coefficient = 0.93 (0.81), coefficient of determination = 0.95 (0.89), and percent bias = −1.17% (2.40%) for the calibration (validation) period. Our results indicate that the assessed risk levels are roughly consistent with the overall property distribution and flood-hazard potential in the study area. The proposed framework and associated findings are valuable for developing adaptation strategies and early-warning systems to reduce flood impacts in the future.
Asadi M., Goli Mokhtari L., Shirzadi A., Shahabi H., Bahrami S.
The main purpose of this study was to compare the performance of Support Vector Machines (SVM), Stochastic Gradient Descent (SGD), and Bayesian Logistic Regression (BLR) algorithms for landslide susceptibility modeling in the Yozidar-Degaga region, Iran. Initially, a distribution map with 175 landslides and 175 non-landslide locations was prepared and the data were classified into a ratio of 80% and 20% for training and model validation, respectively. Based on Information Gain Ratio (IGR) technique, 13 derived factors from topographic data, land cover and rainfall were selected for modeling. Then, the SVM, SGD, and BLR algorithms were selected based on size of the data and required accuracy of the output, to learn and prepare landslide susceptibility maps. Statistical criteria were employed to evaluate the models for both training and validation datasets. Finally, the performance of these models was evaluated by the area under the receiver operating curve (AUC). The results showed that SVM algorithm (AUC = 0.920) performed better than SGD (AUC = 0.918) and BLR (AUC = 0.918) algorithms. Therefore, the SVM model can be suggested as a useful tool for better management of landslide-affected areas in the study area. In this study, all three models (SVM, SGD and BLR) were implemented in WEKA 3.6.9 software environment to prepare landslide susceptibility maps.
Chen Z., Liu Z., Yin L., Zheng W.
Dam construction brings both benefits and harms to the ecological environment. This paper focuses on the impact of the construction of mega-dams on regional climate. Based on the national precipitation and temperature data from 1990 to 2012 from more than 2400 national stations of the National Meteorological Information Center, this paper analyzes Xiaolangdi Dam's influence on the Yellow River's climate and the Three Gorges Dam of the Yangtze River with geostatistics knowledge. The following results are obtained. (1) According to the analysis of the climate time and space distribution in the Yellow River Basin and the Yangtze River Basin, the dam's construction caused a drop in temperature in the reservoir area, which decreased by about 0.12 °C. On the other hand, the dam's construction also caused the temperature in the downstream area to rise by about 0.6 °C. (2) According to the analysis of regional climate correlation, the rise of the dam's water level will cause the temperature of the reservoir area to drop to a certain extent. The correlation between the temperature in the Three Gorges and the water level changes in the Three Gorges reaches −0.578. (3) According to regional climate statistics analysis, the dam's construction has reduced the temperature difference in the reservoir area. • Based on the national precipitation and temperature data from 1990 to 2012 from more than 2400 national stations of the National Meteorological Information Center. • Focuses on the impact of the construction of mega-dams, Xiaolangdi Dam and Three Gorges Dam, and analyzes their influence on the Yellow River's climate. • According to the analysis, dams' construction leads to decreased temperature in the reservoir area. The construction of dams also leads to an increase in temperature in downstream areas. • According to the correlation analysis of regional climate, the increase of dam water level will lead to a decrease in temperature within the reservoir area. • According to the regional climate's statistical analysis, the Dam's construction reduces the reservoir area's temperature difference.
Luan D., Liu A., Wang X., Xie Y., Wu Z.
Disaster medical rescue in China mainly adopts the “on-site rescue” model. Whether the location of emergency temporary blood supply sites is reasonable or not directly affects the rescue efficiency. The paper studies the robust location-allocation for emergency temporary blood supply after disaster. First, the factors of several candidate sites were quantified by the entropy-based TOPSIS method, and 12 candidate blood supply sites with higher priority were selected according to the evaluation indicators. At the same time, the uncertainty of blood demand at each disaster site increased the difficulty of decision-making, and then, a robust location model (MIRP) was constructed with minimum cost with time window constraints. It is also constrained by the uncertain demand for blood in three scenarios. Second, the survival probability function was introduced, and the time window limit was given at the minimum cost to maximize the survival probability of the suffered people. Finally, the numerical example experiments demonstrate that the increase in demand uncertainty and survival probability cause the MIRP model to generate more costs. Compared with the three MIRP models, the MIRP-ellipsoid set model gained better robustness. Also, given the necessary restrictions on the time window, the cost can be reduced by about 13% with the highest survival probability. Decision-makers can select different combinations of uncertainty levels and demand disturbance ratios and necessary time constraints to obtain the optimal location-allocation solution according to risk preference and actual conditions.
Singh S.K., Taylor R.W., Thadaboina V.
This study uses six machine learning (ML) algorithms to evaluate and predict individuals' social resilience towards arsenicosis-affected people in an arsenic-risk society of rural India. Over 50% of the surveyed communities were found to be resilient towards arsenicosis patients. Logistic regression with inbuilt cross-validation (LRCV) model scored the highest accuracy (76%), followed by Gaussian distribution-based naïve Bayes (GNB) model (74%), C-Support Vector (SVC) (74%), K-neighbors (Kn) (73%), Random Forest (RF) (72%), and Decision Tree (DT) (67%). The LRCV also scored the highest kappa value of 0.52, followed by GNB (0.48), SVC (0.48), Kn (0.46), RF (0.42), and DT (0.31). Caste, education, occupation, housing status, sanitation behaviors, trust in others, non-profit and private organizations, social capital, and awareness played a key role in shaping social resilience towards arsenicosis patients. The authors opine that LRCV and GNB could be promising methods to develop models on similar data generated from a risk society.
Wang S., Zhang K., Chao L., Li D., Tian X., Bao H., Chen G., Xia Y.
• Quantified the uncertainty in the radar and GSMaP QPEs in a China’s hilly humid region. • Investigated the radar and GSMaP QPEs utility for integrated flood and landslide forecasting. • Developed a dynamic bias correction method to improve the radar and GSMaP QPEs. • Explored the impacts of time interval and spatial resolution on the bias-correction performance. It is important to develop the integrated flood and landslide modeling system driven by radar and satellite to predict these hazards to mitigate their damages. In this study, we investigated the utility of the C-band, one-polarization radar quantitative precipitation estimation (QPE) and the Global Satellite Mapping of Precipitation (GSMaP) satellite QPE for the integrated prediction of floods and landslides in two hilly basins of southern Shaanxi Province of China. We further developed a dynamic bias correction to reduce uncertainty in radar and satellite QPEs using gauge observations and explored the impacts of gauge density and spatial resolution of QPE on the effectiveness of bias correction. Our results show that the radar and GSMaP QPEs have respective large negative and positive biases. The bias-correction method has significantly improved the quality of both radar and GSMaP QPEs and the associated accuracies in the simulated hydrological processes and slope stability. The bias-correction method with a correction time interval of 24 h can achieve the optimal results for both radar and GSMaP QPEs. Although gauge density and spatial resolution impact the accuracy of the bias-corrected methods for both radar and GSMaP, inclusion of the observations from even a small number of rain gauges will be helpful for reducing the uncertainty in the radar and satellite QPEs.
Li H., Deng J., Yuan S., Feng P., Arachchige D.D.
Wind turbines are widely installed as the new source of cleaner energy production. Dynamic and random stress imposed on the generator bearing of a wind turbine may lead to overheating and failure. In this paper, a data-driven approach for condition monitoring of generator bearings using temporal temperature data is presented. Four algorithms, the support vector regression machine, neural network, extreme learning machine, and the deep belief network are applied to model the bearing behavior. Comparative analysis of the models has demonstrated that the deep belief network is most accurate. It has been observed that the bearing failure is preceded by a change in the prediction error of bearing temperature. An exponentially-weighted moving average (EWMA) control chart is deployed to trend the error. Then a binary vector containing the abnormal errors and the normal residuals are generated for classifying failures. LS-SVM based classification models are developed to classify the fault bearings and the normal ones. The proposed approach has been validated with the data collected from 11 wind turbines.
Li H., Deng J., Feng P., Pu C., Arachchige D.D., Cheng Q.
To maximize energy extraction, the nacelle of a wind turbine follows the wind direction. Accurate prediction of wind direction is vital for yaw control. A tandem hybrid approach to improve the prediction accuracy of the wind direction data is developed. The proposed approach in this paper includes the bilinear transformation, effective data decomposition techniques, long-short-term-memory recurrent neural networks (LSTM-RNNs), and error decomposition correction methods. In the proposed approach, the angular wind direction data is firstly transformed into time-series to accommodate the full range of yaw motion. Then, the continuous transformed series are decomposed into a group of subseries using a novel decomposition technique. Next, for each subseries, the wind directions are predicted using LSTM-RNNs. In the final step, it decomposed the errors for each predicted subseries to correct the predicted wind direction and then perform inverse bilinear transformation to obtain the final wind direction forecasting. The robustness and effectiveness of the proposed approach are verified using data collected from a wind farm located in Huitengxile, Inner Mongolia, China. Computational results indicate that the proposed hybrid approach outperforms the other single approaches tested to predict the nacelle direction over short-time horizons. The proposed approach can be useful for practical wind farm operations.
Guyennon N., Salerno F., Rossi D., Rainaldi M., Calizza E., Romano E.
• Increased variability in precipitation is the main driver of lake water crisis. • Increased temperature plays a marginal role on long-term lake level variations. • Abstraction variably impact on the lake level variability double during last decades. • Machine learning approach can overcome the lack of knowledge on subsurface flow. • Random forest demonstrates notable predictive and inference capacity. Lake Bracciano has been historically used as a strategic water reservoir for the city of Rome (Italy) since ancient times. However, following the severe water crisis of 2017, water abstraction has been completely stopped. The relative impact of the various drivers of change (climatological and management) on fluctuations in lake water level is not yet clear. To quantify this impact, we applied the Random Forest (RF) machine learning approach, taking advantage of a century of observations. Since the late 1990s the monthly variation in lake water levels has doubled, as has variation in monthly abstraction. Increased variation in annual cumulated precipitation and a rise in mean air temperature have also been observed. The RF machine learning approach made it possible to confirm the marginal role of temperature, the increasing role of abstraction during the last two decades (from 24 % to 39 %), and the key role played by the increased precipitation variability. These results highlight the notable prediction and inference capabilities of RF in a complex and partially unknown hydrological context. We conclude by discussing the limits of this approach, which are mainly associated with its capacity to generates scenarios compared to physical based models.
Total publications
49
Total citations
1642
Citations per publication
33.51
Average publications per year
4.08
Average coauthors
2.92
Publications years
2014-2025 (12 years)
h-index
17
i10-index
24
m-index
1.42
o-index
66
g-index
40
w-index
7
Metrics description
h-index
A scientist has an h-index if h of his N publications are cited at least h times each, while the remaining (N - h) publications are cited no more than h times each.
i10-index
The number of the author's publications that received at least 10 links each.
m-index
The researcher's m-index is numerically equal to the ratio of his h-index to the number of years that have passed since the first publication.
o-index
The geometric mean of the h-index and the number of citations of the most cited article of the scientist.
g-index
For a given set of articles, sorted in descending order of the number of citations that these articles received, the g-index is the largest number such that the g most cited articles received (in total) at least g2 citations.
w-index
If w articles of a researcher have at least 10w citations each and other publications are less than 10(w+1) citations, then the researcher's w-index is equal to w.
Top-100
Fields of science
1
2
3
4
5
6
|
|
General Medicine
|
General Medicine, 6, 12.24%
General Medicine
6 publications, 12.24%
|
Water Science and Technology
|
Water Science and Technology, 6, 12.24%
Water Science and Technology
6 publications, 12.24%
|
Geography, Planning and Development
|
Geography, Planning and Development, 6, 12.24%
Geography, Planning and Development
6 publications, 12.24%
|
Health, Toxicology and Mutagenesis
|
Health, Toxicology and Mutagenesis, 5, 10.2%
Health, Toxicology and Mutagenesis
5 publications, 10.2%
|
Environmental Engineering
|
Environmental Engineering, 4, 8.16%
Environmental Engineering
4 publications, 8.16%
|
Public Health, Environmental and Occupational Health
|
Public Health, Environmental and Occupational Health, 4, 8.16%
Public Health, Environmental and Occupational Health
4 publications, 8.16%
|
Renewable Energy, Sustainability and the Environment
|
Renewable Energy, Sustainability and the Environment, 4, 8.16%
Renewable Energy, Sustainability and the Environment
4 publications, 8.16%
|
Management, Monitoring, Policy and Law
|
Management, Monitoring, Policy and Law, 3, 6.12%
Management, Monitoring, Policy and Law
3 publications, 6.12%
|
General Engineering
|
General Engineering, 2, 4.08%
General Engineering
2 publications, 4.08%
|
Environmental Chemistry
|
Environmental Chemistry, 2, 4.08%
Environmental Chemistry
2 publications, 4.08%
|
Pollution
|
Pollution, 2, 4.08%
Pollution
2 publications, 4.08%
|
General Environmental Science
|
General Environmental Science, 2, 4.08%
General Environmental Science
2 publications, 4.08%
|
Ecology, Evolution, Behavior and Systematics
|
Ecology, Evolution, Behavior and Systematics, 2, 4.08%
Ecology, Evolution, Behavior and Systematics
2 publications, 4.08%
|
Atmospheric Science
|
Atmospheric Science, 2, 4.08%
Atmospheric Science
2 publications, 4.08%
|
Earth-Surface Processes
|
Earth-Surface Processes, 2, 4.08%
Earth-Surface Processes
2 publications, 4.08%
|
Global and Planetary Change
|
Global and Planetary Change, 2, 4.08%
Global and Planetary Change
2 publications, 4.08%
|
General Earth and Planetary Sciences
|
General Earth and Planetary Sciences, 2, 4.08%
General Earth and Planetary Sciences
2 publications, 4.08%
|
Computer Science Applications
|
Computer Science Applications, 1, 2.04%
Computer Science Applications
1 publication, 2.04%
|
Multidisciplinary
|
Multidisciplinary, 1, 2.04%
Multidisciplinary
1 publication, 2.04%
|
Process Chemistry and Technology
|
Process Chemistry and Technology, 1, 2.04%
Process Chemistry and Technology
1 publication, 2.04%
|
General Materials Science
|
General Materials Science, 1, 2.04%
General Materials Science
1 publication, 2.04%
|
General Mathematics
|
General Mathematics, 1, 2.04%
General Mathematics
1 publication, 2.04%
|
Instrumentation
|
Instrumentation, 1, 2.04%
Instrumentation
1 publication, 2.04%
|
Mechanical Engineering
|
Mechanical Engineering, 1, 2.04%
Mechanical Engineering
1 publication, 2.04%
|
Mechanics of Materials
|
Mechanics of Materials, 1, 2.04%
Mechanics of Materials
1 publication, 2.04%
|
Civil and Structural Engineering
|
Civil and Structural Engineering, 1, 2.04%
Civil and Structural Engineering
1 publication, 2.04%
|
Waste Management and Disposal
|
Waste Management and Disposal, 1, 2.04%
Waste Management and Disposal
1 publication, 2.04%
|
Space and Planetary Science
|
Space and Planetary Science, 1, 2.04%
Space and Planetary Science
1 publication, 2.04%
|
Earth and Planetary Sciences (miscellaneous)
|
Earth and Planetary Sciences (miscellaneous), 1, 2.04%
Earth and Planetary Sciences (miscellaneous)
1 publication, 2.04%
|
Ecology
|
Ecology, 1, 2.04%
Ecology
1 publication, 2.04%
|
Geophysics
|
Geophysics, 1, 2.04%
Geophysics
1 publication, 2.04%
|
Aerospace Engineering
|
Aerospace Engineering, 1, 2.04%
Aerospace Engineering
1 publication, 2.04%
|
Toxicology
|
Toxicology, 1, 2.04%
Toxicology
1 publication, 2.04%
|
Astronomy and Astrophysics
|
Astronomy and Astrophysics, 1, 2.04%
Astronomy and Astrophysics
1 publication, 2.04%
|
Geology
|
Geology, 1, 2.04%
Geology
1 publication, 2.04%
|
Fluid Flow and Transfer Processes
|
Fluid Flow and Transfer Processes, 1, 2.04%
Fluid Flow and Transfer Processes
1 publication, 2.04%
|
Archeology
|
Archeology, 1, 2.04%
Archeology
1 publication, 2.04%
|
Urban Studies
|
Urban Studies, 1, 2.04%
Urban Studies
1 publication, 2.04%
|
Ecological Modeling
|
Ecological Modeling, 1, 2.04%
Ecological Modeling
1 publication, 2.04%
|
Show all (9 more) | |
1
2
3
4
5
6
|
Journals
1
2
3
|
|
Geocarto International
3 publications, 6.12%
|
|
International Journal of Environmental Research and Public Health
2 publications, 4.08%
|
|
Catena
2 publications, 4.08%
|
|
Sustainability
2 publications, 4.08%
|
|
Clinica Chimica Acta
1 publication, 2.04%
|
|
Environment Systems and Decisions
1 publication, 2.04%
|
|
Quaternary Science Reviews
1 publication, 2.04%
|
|
Environment
1 publication, 2.04%
|
|
Ecotoxicology and Environmental Safety
1 publication, 2.04%
|
|
Emerging Contaminants
1 publication, 2.04%
|
|
Mathematical Problems in Engineering
1 publication, 2.04%
|
|
Groundwater for Sustainable Development
1 publication, 2.04%
|
|
Frontiers in Ecology and the Environment
1 publication, 2.04%
|
|
Natural Hazards
1 publication, 2.04%
|
|
Applied Sciences (Switzerland)
1 publication, 2.04%
|
|
Journal of Environmental Management
1 publication, 2.04%
|
|
Frontiers in Environmental Science
1 publication, 2.04%
|
|
Current Science
1 publication, 2.04%
|
|
International Journal of Structural Integrity
1 publication, 2.04%
|
|
Advances in Space Research
1 publication, 2.04%
|
|
Environmental Science and Pollution Research
1 publication, 2.04%
|
|
AIMS Public Health
1 publication, 2.04%
|
|
Vietnam Journal of Earth Sciences
1 publication, 2.04%
|
|
AIMS Medical Science
1 publication, 2.04%
|
|
International Journal of Sustainable Built Environment
1 publication, 2.04%
|
|
Open Geospatial Data, Software and Standards
1 publication, 2.04%
|
|
Advances in Water Security
1 publication, 2.04%
|
|
Asian Journal of Research in Computer Science
1 publication, 2.04%
|
|
1
2
3
|
Citing journals
10
20
30
40
50
60
70
80
90
100
|
|
Journal not defined
|
Journal not defined, 98, 5.96%
Journal not defined
98 citations, 5.96%
|
Geocarto International
62 citations, 3.77%
|
|
Remote Sensing
60 citations, 3.65%
|
|
Sustainability
49 citations, 2.98%
|
|
Applied Sciences (Switzerland)
45 citations, 2.74%
|
|
Water (Switzerland)
42 citations, 2.56%
|
|
Environmental Science and Pollution Research
36 citations, 2.19%
|
|
Catena
35 citations, 2.13%
|
|
Groundwater for Sustainable Development
33 citations, 2.01%
|
|
Natural Hazards
33 citations, 2.01%
|
|
International Journal of Environmental Research and Public Health
29 citations, 1.77%
|
|
Environmental Earth Sciences
27 citations, 1.64%
|
|
Science of the Total Environment
24 citations, 1.46%
|
|
Bulletin of Engineering Geology and the Environment
23 citations, 1.4%
|
|
Advances in Water Security
22 citations, 1.34%
|
|
Arabian Journal of Geosciences
21 citations, 1.28%
|
|
ISPRS International Journal of Geo-Information
20 citations, 1.22%
|
|
Geoscience Frontiers
19 citations, 1.16%
|
|
Earth Science Informatics
18 citations, 1.1%
|
|
Stochastic Environmental Research and Risk Assessment
17 citations, 1.03%
|
|
Geomatics, Natural Hazards and Risk
16 citations, 0.97%
|
|
Land
15 citations, 0.91%
|
|
Journal of Environmental Management
14 citations, 0.85%
|
|
Journal of Hydrology
14 citations, 0.85%
|
|
Frontiers in Environmental Science
14 citations, 0.85%
|
|
Advances in Space Research
14 citations, 0.85%
|
|
Ecotoxicology and Environmental Safety
13 citations, 0.79%
|
|
Emerging Contaminants
12 citations, 0.73%
|
|
Scientific Reports
12 citations, 0.73%
|
|
Forests
11 citations, 0.67%
|
|
Environmental Pollution
11 citations, 0.67%
|
|
Landslides
10 citations, 0.61%
|
|
Ecological Informatics
10 citations, 0.61%
|
|
Ecological Indicators
10 citations, 0.61%
|
|
Mathematical Problems in Engineering
10 citations, 0.61%
|
|
Journal of Cleaner Production
9 citations, 0.55%
|
|
Environmental Science and Engineering
9 citations, 0.55%
|
|
Lecture Notes in Civil Engineering
9 citations, 0.55%
|
|
Journal of Mountain Science
9 citations, 0.55%
|
|
Environmental Geochemistry and Health
9 citations, 0.55%
|
|
Environmental Monitoring and Assessment
9 citations, 0.55%
|
|
Disaster Risk Reduction
9 citations, 0.55%
|
|
Applied Geomatics
8 citations, 0.49%
|
|
Frontiers in Earth Science
8 citations, 0.49%
|
|
Neural Computing and Applications
7 citations, 0.43%
|
|
Geosciences (Switzerland)
7 citations, 0.43%
|
|
Journal of the Indian Society of Remote Sensing
6 citations, 0.37%
|
|
International Journal of Digital Earth
6 citations, 0.37%
|
|
Physics and Chemistry of the Earth
6 citations, 0.37%
|
|
Environment, Development and Sustainability
6 citations, 0.37%
|
|
Water Resources Management
6 citations, 0.37%
|
|
Journal of Hazardous Materials
6 citations, 0.37%
|
|
Open Construction and Building Technology Journal
6 citations, 0.37%
|
|
PLoS ONE
6 citations, 0.37%
|
|
Materials
6 citations, 0.37%
|
|
IEEE Access
6 citations, 0.37%
|
|
SN Applied Sciences
6 citations, 0.37%
|
|
AIMS Public Health
6 citations, 0.37%
|
|
Soils in the Hindu Kush Himalayas
6 citations, 0.37%
|
|
Geological Journal
5 citations, 0.3%
|
|
Journal of Hydrology: Regional Studies
5 citations, 0.3%
|
|
Advances in Civil Engineering
5 citations, 0.3%
|
|
Lecture Notes in Networks and Systems
5 citations, 0.3%
|
|
Sensors
5 citations, 0.3%
|
|
Geoscience Letters
5 citations, 0.3%
|
|
Environmental Archaeology
5 citations, 0.3%
|
|
Engineering Applications of Artificial Intelligence
5 citations, 0.3%
|
|
Heliyon
5 citations, 0.3%
|
|
Chemosphere
5 citations, 0.3%
|
|
Marine Pollution Bulletin
4 citations, 0.24%
|
|
Environmental Research
4 citations, 0.24%
|
|
Natural Hazards Review
4 citations, 0.24%
|
|
Quaternary Science Reviews
4 citations, 0.24%
|
|
IEEE Journal of Selected Topics in Applied Earth Observations and Remote Sensing
4 citations, 0.24%
|
|
Biological Trace Element Research
4 citations, 0.24%
|
|
Ground Water
4 citations, 0.24%
|
|
International Journal of Environmental Science and Technology
4 citations, 0.24%
|
|
International Journal of Applied Earth Observation and Geoinformation
4 citations, 0.24%
|
|
Quaternary Science Advances
4 citations, 0.24%
|
|
Discover Environment
4 citations, 0.24%
|
|
Natural Resources Research
3 citations, 0.18%
|
|
Journal of Contaminant Hydrology
3 citations, 0.18%
|
|
Modern Cartography Series
3 citations, 0.18%
|
|
Journal of Island and Coastal Archaeology
3 citations, 0.18%
|
|
Knowledge-Based Systems
3 citations, 0.18%
|
|
Environment Systems and Decisions
3 citations, 0.18%
|
|
Environmental Modelling and Software
3 citations, 0.18%
|
|
Frontiers in Ecology and Evolution
3 citations, 0.18%
|
|
Water Practice and Technology
3 citations, 0.18%
|
|
Atmosphere
3 citations, 0.18%
|
|
Communications in Computer and Information Science
3 citations, 0.18%
|
|
Environmental Quality Management
3 citations, 0.18%
|
|
Proceedings of the Indian National Science Academy
3 citations, 0.18%
|
|
Holocene
3 citations, 0.18%
|
|
Gondwana Research
3 citations, 0.18%
|
|
Advances in Natural and Technological Hazards Research
3 citations, 0.18%
|
|
Environmental Technology and Innovation
3 citations, 0.18%
|
|
Multimedia Tools and Applications
3 citations, 0.18%
|
|
Journal of Earth System Science
3 citations, 0.18%
|
|
Symmetry
3 citations, 0.18%
|
|
Show all (70 more) | |
10
20
30
40
50
60
70
80
90
100
|
Publishers
2
4
6
8
10
|
|
Elsevier
10 publications, 20.41%
|
|
Springer Nature
5 publications, 10.2%
|
|
MDPI
5 publications, 10.2%
|
|
Taylor & Francis
4 publications, 8.16%
|
|
American Institute of Mathematical Sciences (AIMS)
2 publications, 4.08%
|
|
Wiley
1 publication, 2.04%
|
|
Emerald
1 publication, 2.04%
|
|
Frontiers Media S.A.
1 publication, 2.04%
|
|
Indian Academy of Sciences
1 publication, 2.04%
|
|
Hindawi Limited
1 publication, 2.04%
|
|
Sciencedomain International
1 publication, 2.04%
|
|
Publishing House for Science and Technology, Vietnam Academy of Science and Technology (Publications)
1 publication, 2.04%
|
|
2
4
6
8
10
|
Organizations from articles
5
10
15
20
25
30
35
|
|
Organization not defined
|
Organization not defined, 32, 65.31%
Organization not defined
32 publications, 65.31%
|
Duy Tan University
9 publications, 18.37%
|
|
University of Kurdistan
7 publications, 14.29%
|
|
Ton Duc Thang University
6 publications, 12.24%
|
|
Vietnam Academy of Science and Technology
4 publications, 8.16%
|
|
Lulea University of Technology
4 publications, 8.16%
|
|
Research institute of forests and rangelands
3 publications, 6.12%
|
|
Nguyen Tat Thanh University
3 publications, 6.12%
|
|
University of Technology, Malaysia
3 publications, 6.12%
|
|
Nagaoka University of Technology
2 publications, 4.08%
|
|
University of Tehran
1 publication, 2.04%
|
|
Tarbiat Modares University
1 publication, 2.04%
|
|
Indian Institute of Technology (Banaras Hindu University) Varanasi
1 publication, 2.04%
|
|
Jadavpur University
1 publication, 2.04%
|
|
Sri Ramachandra Institute of Higher Education and Research
1 publication, 2.04%
|
|
University of Kashan
1 publication, 2.04%
|
|
VNU University of Science
1 publication, 2.04%
|
|
Le Quy Don Technical University
1 publication, 2.04%
|
|
Medical College and Hospital, Kolkata
1 publication, 2.04%
|
|
Calcutta National Medical College and Hospital
1 publication, 2.04%
|
|
Institute of Post-Graduate Medical Education and Research and Seth Sukhlal Karnani Memorial Hospital
1 publication, 2.04%
|
|
University of Technology Sydney
1 publication, 2.04%
|
|
Xi'An University of Science and Technology
1 publication, 2.04%
|
|
University of Newcastle Australia
1 publication, 2.04%
|
|
Korea University of Science and Technology
1 publication, 2.04%
|
|
Sejong University
1 publication, 2.04%
|
|
Korea Institute of Geoscience and Mineral Resources
1 publication, 2.04%
|
|
University of Central Florida
1 publication, 2.04%
|
|
Simon Fraser University
1 publication, 2.04%
|
|
University of Tokyo
1 publication, 2.04%
|
|
University of Florida
1 publication, 2.04%
|
|
University of Cincinnati
1 publication, 2.04%
|
|
Chittaranjan National Cancer Institute
1 publication, 2.04%
|
|
Kazimierz Pułaski University of Radom
1 publication, 2.04%
|
|
University of Missouri
1 publication, 2.04%
|
|
Show all (5 more) | |
5
10
15
20
25
30
35
|
Countries from articles
5
10
15
20
25
30
|
|
USA
|
USA, 28, 57.14%
USA
28 publications, 57.14%
|
Country not defined
|
Country not defined, 21, 42.86%
Country not defined
21 publications, 42.86%
|
India
|
India, 13, 26.53%
India
13 publications, 26.53%
|
Vietnam
|
Vietnam, 12, 24.49%
Vietnam
12 publications, 24.49%
|
Iran
|
Iran, 11, 22.45%
Iran
11 publications, 22.45%
|
Sweden
|
Sweden, 5, 10.2%
Sweden
5 publications, 10.2%
|
Malaysia
|
Malaysia, 4, 8.16%
Malaysia
4 publications, 8.16%
|
Australia
|
Australia, 3, 6.12%
Australia
3 publications, 6.12%
|
Japan
|
Japan, 3, 6.12%
Japan
3 publications, 6.12%
|
Canada
|
Canada, 2, 4.08%
Canada
2 publications, 4.08%
|
Republic of Korea
|
Republic of Korea, 2, 4.08%
Republic of Korea
2 publications, 4.08%
|
China
|
China, 1, 2.04%
China
1 publication, 2.04%
|
Norway
|
Norway, 1, 2.04%
Norway
1 publication, 2.04%
|
Poland
|
Poland, 1, 2.04%
Poland
1 publication, 2.04%
|
5
10
15
20
25
30
|
Citing organizations
50
100
150
200
250
300
|
|
Organization not defined
|
Organization not defined, 266, 16.2%
Organization not defined
266 citations, 16.2%
|
Duy Tan University
91 citations, 5.54%
|
|
Ton Duc Thang University
47 citations, 2.86%
|
|
Tarbiat Modares University
43 citations, 2.62%
|
|
Vietnam Academy of Science and Technology
43 citations, 2.62%
|
|
China University of Geosciences (Wuhan)
41 citations, 2.5%
|
|
Xi'An University of Science and Technology
41 citations, 2.5%
|
|
University of Kurdistan
39 citations, 2.38%
|
|
VNU University of Science
35 citations, 2.13%
|
|
University of Burdwan
32 citations, 1.95%
|
|
Lulea University of Technology
31 citations, 1.89%
|
|
Korea University of Science and Technology
29 citations, 1.77%
|
|
Korea Institute of Geoscience and Mineral Resources
26 citations, 1.58%
|
|
University of Tehran
25 citations, 1.52%
|
|
Research institute of forests and rangelands
25 citations, 1.52%
|
|
University of Technology, Malaysia
22 citations, 1.34%
|
|
Chengdu University of Technology
22 citations, 1.34%
|
|
Gorgan University of Agricultural Sciences and Natural Resources
20 citations, 1.22%
|
|
Vietnam National University, Hanoi
18 citations, 1.1%
|
|
University of Technology Sydney
18 citations, 1.1%
|
|
King Saud University
17 citations, 1.04%
|
|
Jamia Millia Islamia
17 citations, 1.04%
|
|
Sejong University
17 citations, 1.04%
|
|
King Khalid University
16 citations, 0.97%
|
|
Jadavpur University
15 citations, 0.91%
|
|
University of Calcutta
15 citations, 0.91%
|
|
Central South University
15 citations, 0.91%
|
|
Simon Fraser University
15 citations, 0.91%
|
|
China University of Geosciences (Beijing)
14 citations, 0.85%
|
|
University of Chinese Academy of Sciences
13 citations, 0.79%
|
|
Southwest Jiaotong University
13 citations, 0.79%
|
|
Texas State University
13 citations, 0.79%
|
|
University of Tabriz
12 citations, 0.73%
|
|
Indian Institute of Technology Roorkee
11 citations, 0.67%
|
|
Shanghai Jiao Tong University
11 citations, 0.67%
|
|
Jilin University
11 citations, 0.67%
|
|
National Technical University of Athens
11 citations, 0.67%
|
|
University of Vienna
11 citations, 0.67%
|
|
University of Delhi
10 citations, 0.61%
|
|
University of Kashan
10 citations, 0.61%
|
|
Sichuan University
10 citations, 0.61%
|
|
University of Manchester
10 citations, 0.61%
|
|
University of South-Eastern Norway
10 citations, 0.61%
|
|
Hiroshima University
10 citations, 0.61%
|
|
Nagaoka University of Technology
10 citations, 0.61%
|
|
Begum Rokeya University
10 citations, 0.61%
|
|
Shiraz University
9 citations, 0.55%
|
|
Soil Conservation and Watershed Management Research Institute
9 citations, 0.55%
|
|
Savitribai Phule Pune University
9 citations, 0.55%
|
|
Delhi Technological University
9 citations, 0.55%
|
|
Sari Agricultural Sciences and Natural Resources University
9 citations, 0.55%
|
|
Wuhan University
9 citations, 0.55%
|
|
Chang'an University
9 citations, 0.55%
|
|
University of Adelaide
9 citations, 0.55%
|
|
Leiden University
9 citations, 0.55%
|
|
University of Salzburg
9 citations, 0.55%
|
|
Indian Institute of Technology Kharagpur
8 citations, 0.49%
|
|
Banaras Hindu University
8 citations, 0.49%
|
|
Hanoi University of Mining and Geology
8 citations, 0.49%
|
|
Can Tho University
8 citations, 0.49%
|
|
National University of Malaysia
8 citations, 0.49%
|
|
Max Planck Institute of Geoanthropology
8 citations, 0.49%
|
|
King Abdulaziz University
7 citations, 0.43%
|
|
Indian Institute of Technology (Indian School of Mines) Dhanbad
7 citations, 0.43%
|
|
Islamic Azad University, Tehran
7 citations, 0.43%
|
|
Amity University, Noida
7 citations, 0.43%
|
|
Tenaga National University
7 citations, 0.43%
|
|
University of Naples Federico II
7 citations, 0.43%
|
|
University of Newcastle Australia
7 citations, 0.43%
|
|
Kangwon National University
7 citations, 0.43%
|
|
Institute of Geographic Sciences and Natural Resources Research, Chinese Academy of Sciences
7 citations, 0.43%
|
|
University of Engineering and Technology, Peshawar
6 citations, 0.37%
|
|
Aligarh Muslim University
6 citations, 0.37%
|
|
National Institute of Technology Patna
6 citations, 0.37%
|
|
Beijing Normal University
6 citations, 0.37%
|
|
Vietnam National University Ho Chi Minh City
6 citations, 0.37%
|
|
Vietnam National University of Agriculture
6 citations, 0.37%
|
|
Vinh University
6 citations, 0.37%
|
|
Central University of Himachal Pradesh
6 citations, 0.37%
|
|
Nanjing Normal University
6 citations, 0.37%
|
|
Nanchang University
6 citations, 0.37%
|
|
National Yunlin University of Science and Technology
6 citations, 0.37%
|
|
Northwest University
6 citations, 0.37%
|
|
Chung-Ang University
6 citations, 0.37%
|
|
Óbuda University
6 citations, 0.37%
|
|
University of Amsterdam
6 citations, 0.37%
|
|
University of Tokyo
6 citations, 0.37%
|
|
University of Coimbra
6 citations, 0.37%
|
|
Florida International University
6 citations, 0.37%
|
|
Ibn Zohr University
6 citations, 0.37%
|
|
China Geological Survey
6 citations, 0.37%
|
|
Hacettepe University
5 citations, 0.3%
|
|
Ferdowsi University of Mashhad
5 citations, 0.3%
|
|
Jawaharlal Nehru University
5 citations, 0.3%
|
|
University of Kerala
5 citations, 0.3%
|
|
Khajeh Nasir Toosi University of Technology
5 citations, 0.3%
|
|
Ho Chi Minh City University of Technology
5 citations, 0.3%
|
|
Nguyen Tat Thanh University
5 citations, 0.3%
|
|
Hanoi University of Natural Resources and Environment
5 citations, 0.3%
|
|
National Botanical Research Institute
5 citations, 0.3%
|
|
Show all (70 more) | |
50
100
150
200
250
300
|
Citing countries
50
100
150
200
250
300
350
400
450
|
|
India
|
India, 412, 25.09%
India
412 citations, 25.09%
|
China
|
China, 340, 20.71%
China
340 citations, 20.71%
|
Country not defined
|
Country not defined, 182, 11.08%
Country not defined
182 citations, 11.08%
|
Iran
|
Iran, 175, 10.66%
Iran
175 citations, 10.66%
|
Vietnam
|
Vietnam, 167, 10.17%
Vietnam
167 citations, 10.17%
|
USA
|
USA, 147, 8.95%
USA
147 citations, 8.95%
|
Republic of Korea
|
Republic of Korea, 71, 4.32%
Republic of Korea
71 citations, 4.32%
|
Australia
|
Australia, 68, 4.14%
Australia
68 citations, 4.14%
|
Saudi Arabia
|
Saudi Arabia, 62, 3.78%
Saudi Arabia
62 citations, 3.78%
|
United Kingdom
|
United Kingdom, 58, 3.53%
United Kingdom
58 citations, 3.53%
|
Malaysia
|
Malaysia, 55, 3.35%
Malaysia
55 citations, 3.35%
|
Sweden
|
Sweden, 44, 2.68%
Sweden
44 citations, 2.68%
|
Italy
|
Italy, 40, 2.44%
Italy
40 citations, 2.44%
|
Bangladesh
|
Bangladesh, 39, 2.38%
Bangladesh
39 citations, 2.38%
|
Canada
|
Canada, 39, 2.38%
Canada
39 citations, 2.38%
|
Japan
|
Japan, 38, 2.31%
Japan
38 citations, 2.31%
|
Germany
|
Germany, 30, 1.83%
Germany
30 citations, 1.83%
|
Pakistan
|
Pakistan, 30, 1.83%
Pakistan
30 citations, 1.83%
|
Turkey
|
Turkey, 28, 1.71%
Turkey
28 citations, 1.71%
|
Romania
|
Romania, 26, 1.58%
Romania
26 citations, 1.58%
|
Netherlands
|
Netherlands, 25, 1.52%
Netherlands
25 citations, 1.52%
|
Spain
|
Spain, 24, 1.46%
Spain
24 citations, 1.46%
|
Austria
|
Austria, 23, 1.4%
Austria
23 citations, 1.4%
|
Greece
|
Greece, 21, 1.28%
Greece
21 citations, 1.28%
|
France
|
France, 19, 1.16%
France
19 citations, 1.16%
|
Brazil
|
Brazil, 18, 1.1%
Brazil
18 citations, 1.1%
|
Morocco
|
Morocco, 18, 1.1%
Morocco
18 citations, 1.1%
|
Algeria
|
Algeria, 17, 1.04%
Algeria
17 citations, 1.04%
|
Norway
|
Norway, 17, 1.04%
Norway
17 citations, 1.04%
|
Poland
|
Poland, 16, 0.97%
Poland
16 citations, 0.97%
|
Portugal
|
Portugal, 15, 0.91%
Portugal
15 citations, 0.91%
|
Mexico
|
Mexico, 14, 0.85%
Mexico
14 citations, 0.85%
|
Indonesia
|
Indonesia, 13, 0.79%
Indonesia
13 citations, 0.79%
|
Iraq
|
Iraq, 13, 0.79%
Iraq
13 citations, 0.79%
|
Hungary
|
Hungary, 12, 0.73%
Hungary
12 citations, 0.73%
|
Egypt
|
Egypt, 11, 0.67%
Egypt
11 citations, 0.67%
|
Ethiopia
|
Ethiopia, 10, 0.61%
Ethiopia
10 citations, 0.61%
|
Nigeria
|
Nigeria, 9, 0.55%
Nigeria
9 citations, 0.55%
|
New Zealand
|
New Zealand, 8, 0.49%
New Zealand
8 citations, 0.49%
|
Thailand
|
Thailand, 8, 0.49%
Thailand
8 citations, 0.49%
|
Tunisia
|
Tunisia, 8, 0.49%
Tunisia
8 citations, 0.49%
|
Kuwait
|
Kuwait, 7, 0.43%
Kuwait
7 citations, 0.43%
|
Nepal
|
Nepal, 7, 0.43%
Nepal
7 citations, 0.43%
|
Slovakia
|
Slovakia, 6, 0.37%
Slovakia
6 citations, 0.37%
|
Czech Republic
|
Czech Republic, 6, 0.37%
Czech Republic
6 citations, 0.37%
|
Switzerland
|
Switzerland, 6, 0.37%
Switzerland
6 citations, 0.37%
|
Russia
|
Russia, 5, 0.3%
Russia
5 citations, 0.3%
|
Qatar
|
Qatar, 5, 0.3%
Qatar
5 citations, 0.3%
|
Cyprus
|
Cyprus, 5, 0.3%
Cyprus
5 citations, 0.3%
|
South Africa
|
South Africa, 5, 0.3%
South Africa
5 citations, 0.3%
|
Belgium
|
Belgium, 4, 0.24%
Belgium
4 citations, 0.24%
|
Colombia
|
Colombia, 4, 0.24%
Colombia
4 citations, 0.24%
|
UAE
|
UAE, 4, 0.24%
UAE
4 citations, 0.24%
|
Oman
|
Oman, 4, 0.24%
Oman
4 citations, 0.24%
|
Ghana
|
Ghana, 3, 0.18%
Ghana
3 citations, 0.18%
|
Georgia
|
Georgia, 3, 0.18%
Georgia
3 citations, 0.18%
|
Dominican Republic
|
Dominican Republic, 3, 0.18%
Dominican Republic
3 citations, 0.18%
|
Jordan
|
Jordan, 3, 0.18%
Jordan
3 citations, 0.18%
|
Peru
|
Peru, 3, 0.18%
Peru
3 citations, 0.18%
|
Slovenia
|
Slovenia, 3, 0.18%
Slovenia
3 citations, 0.18%
|
Philippines
|
Philippines, 3, 0.18%
Philippines
3 citations, 0.18%
|
Sri Lanka
|
Sri Lanka, 3, 0.18%
Sri Lanka
3 citations, 0.18%
|
Ecuador
|
Ecuador, 3, 0.18%
Ecuador
3 citations, 0.18%
|
Burkina Faso
|
Burkina Faso, 2, 0.12%
Burkina Faso
2 citations, 0.12%
|
Bhutan
|
Bhutan, 2, 0.12%
Bhutan
2 citations, 0.12%
|
Denmark
|
Denmark, 2, 0.12%
Denmark
2 citations, 0.12%
|
Zimbabwe
|
Zimbabwe, 2, 0.12%
Zimbabwe
2 citations, 0.12%
|
Israel
|
Israel, 2, 0.12%
Israel
2 citations, 0.12%
|
Ireland
|
Ireland, 2, 0.12%
Ireland
2 citations, 0.12%
|
Cameroon
|
Cameroon, 2, 0.12%
Cameroon
2 citations, 0.12%
|
Kenya
|
Kenya, 2, 0.12%
Kenya
2 citations, 0.12%
|
Côte d'Ivoire
|
Côte d'Ivoire, 2, 0.12%
Côte d'Ivoire
2 citations, 0.12%
|
Moldova
|
Moldova, 2, 0.12%
Moldova
2 citations, 0.12%
|
Rwanda
|
Rwanda, 2, 0.12%
Rwanda
2 citations, 0.12%
|
Serbia
|
Serbia, 2, 0.12%
Serbia
2 citations, 0.12%
|
Tanzania
|
Tanzania, 2, 0.12%
Tanzania
2 citations, 0.12%
|
Uzbekistan
|
Uzbekistan, 2, 0.12%
Uzbekistan
2 citations, 0.12%
|
Montenegro
|
Montenegro, 2, 0.12%
Montenegro
2 citations, 0.12%
|
Chile
|
Chile, 2, 0.12%
Chile
2 citations, 0.12%
|
Jamaica
|
Jamaica, 2, 0.12%
Jamaica
2 citations, 0.12%
|
Kazakhstan
|
Kazakhstan, 1, 0.06%
Kazakhstan
1 citation, 0.06%
|
Antigua and Barbuda
|
Antigua and Barbuda, 1, 0.06%
Antigua and Barbuda
1 citation, 0.06%
|
Afghanistan
|
Afghanistan, 1, 0.06%
Afghanistan
1 citation, 0.06%
|
Bahamas
|
Bahamas, 1, 0.06%
Bahamas
1 citation, 0.06%
|
Barbados
|
Barbados, 1, 0.06%
Barbados
1 citation, 0.06%
|
Bosnia and Herzegovina
|
Bosnia and Herzegovina, 1, 0.06%
Bosnia and Herzegovina
1 citation, 0.06%
|
Brunei
|
Brunei, 1, 0.06%
Brunei
1 citation, 0.06%
|
Venezuela
|
Venezuela, 1, 0.06%
Venezuela
1 citation, 0.06%
|
Haiti
|
Haiti, 1, 0.06%
Haiti
1 citation, 0.06%
|
Honduras
|
Honduras, 1, 0.06%
Honduras
1 citation, 0.06%
|
Grenada
|
Grenada, 1, 0.06%
Grenada
1 citation, 0.06%
|
Dominica
|
Dominica, 1, 0.06%
Dominica
1 citation, 0.06%
|
Zambia
|
Zambia, 1, 0.06%
Zambia
1 citation, 0.06%
|
Yemen
|
Yemen, 1, 0.06%
Yemen
1 citation, 0.06%
|
Cambodia
|
Cambodia, 1, 0.06%
Cambodia
1 citation, 0.06%
|
Democratic Republic of the Congo
|
Democratic Republic of the Congo, 1, 0.06%
Democratic Republic of the Congo
1 citation, 0.06%
|
Cuba
|
Cuba, 1, 0.06%
Cuba
1 citation, 0.06%
|
|
, 1, 0.06%
1 citation, 0.06%
|
Mali
|
Mali, 1, 0.06%
Mali
1 citation, 0.06%
|
Martinique
|
Martinique, 1, 0.06%
Martinique
1 citation, 0.06%
|
Show all (70 more) | |
50
100
150
200
250
300
350
400
450
|
- We do not take into account publications without a DOI.
- Statistics recalculated daily.